Table Of Contents
Category
Real Estate
Marketing
CRM
FinTech
Healthcare & Medicine
1. What is Asset Management?
Asset management is the systematic process of developing, operating, maintaining, and selling assets in a cost-effective manner. It involves managing investments on behalf of clients, which can include individuals, institutions, and corporations. The primary objective is to maximize returns while minimizing risks associated with the assets. The global market for AI in asset management is projected to grow from $2.7 billion in 2022 to $13.4 billion by 2027, at a Compound Annual Growth Rate (CAGR) of 37.8%. According to a survey by Deloitte, 86% of financial services AI adopters believe that AI will be very or critically important to their business's success in the next two years. The adoption of AI in investment strategies has led to the creation of AI-powered hedge funds, which now manage over $940 billion in assets, representing a 700% increase since 2018.
1.1. Introduction to Asset Management
- Asset management encompasses a wide range of financial services, including managing various types of assets such as stocks, bonds, real estate, and other investment vehicles.
- The process is typically carried out by professional asset managers or firms that specialize in investment strategies, such as asset management companies and groups.
- Asset management is crucial for both individual investors and large institutions, as it helps in achieving financial goals and ensuring long-term growth.
- The industry has evolved significantly with the advent of technology, leading to the rise of robo-advisors in algorithm-driven investment strategies, including alternative investment management.
1.2. Core Functions and Goals
- Investment Strategy Development: Creating tailored investment strategies based on client objectives and risk tolerance, while utilizing market research and analysis to inform decisions.
- Portfolio Management: Constructing and managing a diversified portfolio to optimize returns and regularly rebalancing the portfolio to align with market conditions and investment goals.
- Risk Management: Identifying potential risks associated with investments and implementing strategies to mitigate them, as well as monitoring market trends and economic indicators to adjust strategies accordingly.
- Performance Measurement: Evaluating the performance of investments against benchmarks and industry standards, and providing clients with regular reports and updates on portfolio performance.
- Client Relationship Management: Building and maintaining strong relationships with clients to understand their needs and preferences, while offering personalized advice and support to help clients achieve their financial objectives.
- Financial Regulatory Compliance: Ensuring that all investment activities comply with relevant laws and regulations, and keeping abreast of changes in the regulatory landscape to avoid potential legal issues.
- Goal Achievement: Aiming to meet specific financial goals such as retirement planning, wealth accumulation, or funding education, and aligning investment strategies with clients' long-term objectives to ensure sustainable growth.
At Rapid Innovation, we leverage our expertise in AI banking solutions and blockchain technology to enhance asset management processes. By integrating advanced ai with banking and finance, we help clients achieve greater ROI through improved decision-making, risk assessment, and operational efficiency. Partnering with us means you can expect tailored strategies, enhanced transparency, and a commitment to helping you reach your financial goals effectively and efficiently, whether through managed discretionary accounts or working with firms like JP Morgan wealth management or Pacific Investment Management Company.
Refer to the image for a visual representation of the asset management process.

2. How Generative AI Transforms Asset Management
Generative AI in asset management is revolutionizing the industry by enhancing decision-making processes, improving efficiency, and enabling more personalized investment strategies. This transformation is driven by advanced algorithms that can analyze vast amounts of data and generate insights that were previously unattainable.
2.1. Understanding Generative AI Technology
Generative AI refers to a subset of artificial intelligence that focuses on creating new content or data based on existing information. It utilizes machine learning models, particularly deep learning, to understand patterns and generate outputs that mimic human-like creativity.
- Key components of Generative AI:
- Neural Networks: These are algorithms modeled after the human brain, capable of learning from large datasets.
- Natural Language Processing (NLP): This allows AI to understand and generate human language, making it useful for analyzing financial reports and news.
- Generative Adversarial Networks (GANs): These consist of two neural networks that work against each other to improve the quality of generated data.
- Applications in finance:
- Data Generation: Creating synthetic data for training models when real data is scarce.
- Risk Assessment: Generating scenarios to evaluate potential risks in investment portfolios.
- Market Predictions: Analyzing trends and generating forecasts based on historical data.
2.2. Integration of AI into Asset Management
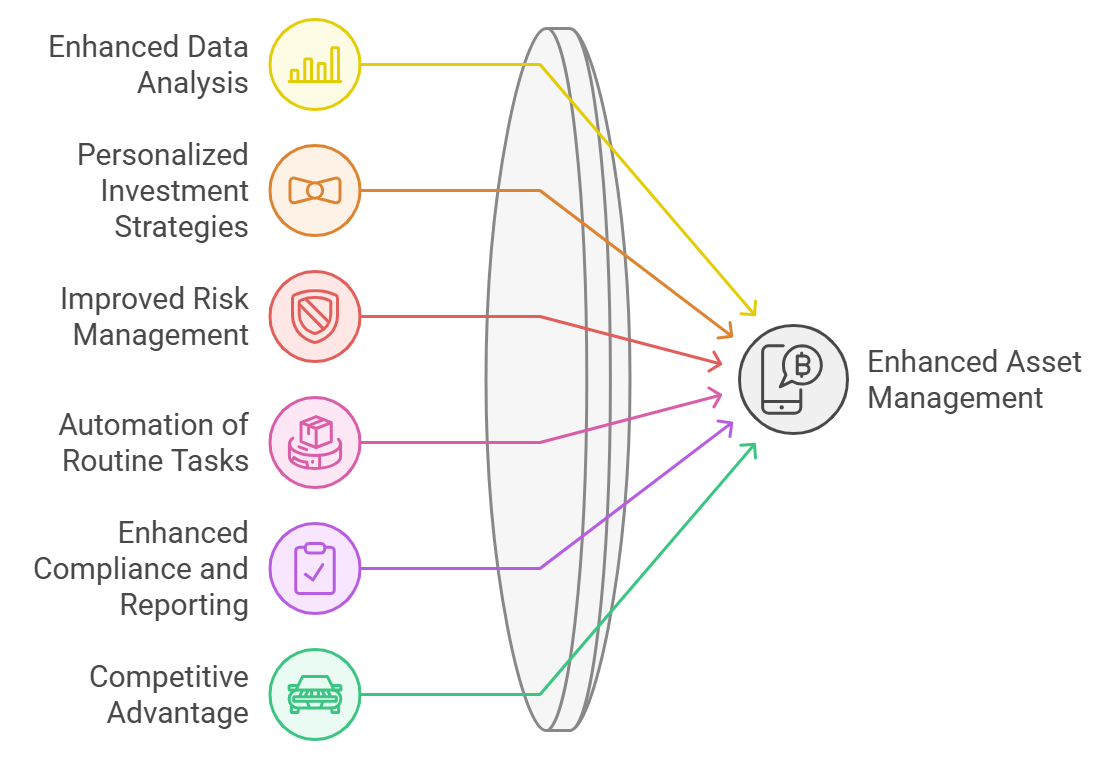
The integration of generative AI in asset management is reshaping how firms operate, enabling them to leverage data-driven insights for better investment strategies.
- Enhanced Data Analysis: Generative AI can process and analyze large datasets much faster than traditional methods. It identifies patterns and correlations that human analysts might overlook, leading to more informed investment decisions based on comprehensive data analysis.
- Personalized Investment Strategies: Generative AI can tailor investment strategies to individual client preferences and risk tolerances. By analyzing client data, it creates customized portfolios that align with specific goals, enhancing client satisfaction and retention.
- Improved Risk Management: AI models can simulate various market conditions and stress-test portfolios. They provide insights into potential vulnerabilities and help in developing mitigation strategies, leading to more resilient investment portfolios.
- Automation of Routine Tasks: Generative AI can automate repetitive tasks such as data entry and report generation, freeing up human analysts to focus on more strategic activities. This increased efficiency can lead to cost savings and improved operational performance.
- Enhanced Compliance and Reporting: AI can assist in monitoring regulatory changes and ensuring compliance. It can generate reports that meet regulatory requirements, reducing the risk of non-compliance, which is crucial in an industry facing constant scrutiny and evolving regulations.
- Competitive Advantage: Firms that adopt generative AI in asset management can gain a significant edge over competitors. The ability to analyze data quickly and accurately allows for faster decision-making, leading to better investment outcomes and increased market share.
In conclusion, the integration of generative AI in asset management is not just a trend; it is a fundamental shift that is reshaping the industry. By harnessing the power of AI, asset managers can enhance their analytical capabilities, improve client services, and navigate the complexities of the financial markets more effectively. At Rapid Innovation, we are committed to helping our clients leverage these advancements to achieve greater ROI and operational excellence.
For more insights on the role of AI in product management, check out AI in Product Management: A Comprehensive Guide.
Refer to the image for a visual representation of how generative AI transforms asset management:

3. Key Use Cases for Generative AI in Asset Management
Generative AI in asset management is transforming the industry by enhancing decision-making processes, improving efficiency, and enabling more personalized investment strategies. Here are two key use cases where generative AI is making a significant impact.
3.1. Optimizing and Generating Portfolios
Generative AI can play a crucial role in portfolio management by optimizing asset allocation and generating new investment strategies.
- Portfolio Optimization: AI algorithms analyze vast amounts of historical data to identify patterns and correlations among different assets. They can simulate various market conditions to determine the best asset allocation that maximizes returns while minimizing risk. This process can lead to more efficient portfolios that are tailored to specific risk tolerances and investment goals.
- Generating New Investment Strategies: Generative AI in asset management can create novel investment strategies by combining different asset classes and investment styles. It can identify underexplored opportunities in the market, such as emerging sectors or geographic regions. By leveraging machine learning, AI can continuously learn from market changes and adapt strategies accordingly.
- Risk Management: AI enhance risk assessment by predicting potential downturns and market volatility. It provides real-time insights into portfolio performance, allowing managers to make informed adjustments. This proactive approach helps in mitigating losses and capitalizing on favorable market conditions.
- Customization: Generative AI enables the creation of personalized portfolios based on individual investor preferences and financial goals. It can analyze client data to recommend tailored investment options that align with their risk appetite and investment horizon.
3.2. Using Predictive Analytics for Investment Decisions
Predictive analytics powered by generative AI is revolutionizing how asset managers make investment decisions.
- Enhanced Forecasting: AI models can analyze historical data and current market trends to forecast future asset performance. These models can incorporate a wide range of variables, including economic indicators, market sentiment, and geopolitical events. This comprehensive analysis leads to more accurate predictions and informed investment choices.
- Sentiment Analysis: Generative AI can process unstructured data from news articles, social media, and financial reports to gauge market sentiment. By understanding public perception and sentiment trends, asset managers can make timely investment decisions. This capability allows for a more nuanced understanding of market dynamics beyond traditional financial metrics.
- Scenario Analysis: AI can simulate various economic scenarios to assess potential impacts on investment portfolios. This helps managers prepare for different market conditions and adjust their strategies proactively. Scenario analysis can also aid in stress testing portfolios against extreme market events.
- Automation of Decision-Making: Generative AI can automate routine investment decisions, allowing asset managers to focus on strategic planning. By integrating AI into trading systems, firms can execute trades based on predefined criteria without human intervention. This automation can lead to faster execution and reduced transaction costs.
- Continuous Learning: AI systems can continuously learn from new data, improving their predictive capabilities over time. This adaptability ensures that investment strategies remain relevant in a rapidly changing market environment. As AI models evolve, they can uncover new insights that may not be apparent through traditional analysis methods.
By partnering with Rapid Innovation, clients can leverage these advanced capabilities to achieve greater ROI, streamline their operations, and enhance their investment strategies. Our expertise in AI banking services ensures that we provide tailored solutions that meet the unique needs of each client, ultimately driving efficiency and effectiveness in their asset management processes.
Refer to the image for a visual representation of the key use cases for Generative AI in Asset Management:

3.3. Conducting Scenario Analysis for Risk Management
Scenario analysis is a critical tool in risk management that helps organizations anticipate potential future events and their impacts. It involves creating detailed narratives about different possible futures and assessing how these scenarios could affect an organization’s objectives.
- Definition: Scenario analysis is a process of analyzing possible future events by considering alternative possible outcomes (scenarios).
- Purpose:
- Identify risks and opportunities.
- Evaluate the potential impact of different scenarios on business operations.
- Support strategic planning and decision-making.
- Steps in Scenario Analysis:
- Identify key variables: Determine the factors that could influence outcomes, such as market trends, regulatory changes, or economic conditions.
- Develop scenarios: Create a range of plausible scenarios, including best-case, worst-case, and moderate-case situations. This includes conducting scenario analysis for risk management, operational risk scenario analysis, and worst case scenario risk management.
- Analyze impacts: Assess how each scenario would affect the organization’s financial performance, operational capabilities, and strategic goals. This can involve scenario analysis in risk management examples and risk assessment scenario example.
- Develop response strategies: Formulate action plans to mitigate risks or capitalize on opportunities identified in the scenarios, including the role of scenario analysis in risk management.
- Benefits:
- Enhances understanding of uncertainties and risks.
- Improves strategic planning by considering a wider range of possibilities.
- Facilitates better communication among stakeholders regarding potential risks.
- Applications:
- Financial institutions use scenario analysis to assess credit risk and market risk.
- Corporations apply it to evaluate the impact of economic downturns or regulatory changes on their business models, such as climate risk management and scenario analysis.
4. Advanced Applications of Generative AI
Generative AI refers to algorithms that can generate new content, designs, or solutions based on existing data. Its advanced applications are transforming various industries by enhancing creativity, efficiency, and decision-making.
- Definition: Generative AI uses machine learning models to create new data that resembles existing data, including text, images, and audio.
- Key Technologies:
- Natural Language Processing (NLP): Enables machines to understand and generate human language.
- Generative Adversarial Networks (GANs): A framework where two neural networks compete to create realistic data.
- Variational Autoencoders (VAEs): Used for generating new data points by learning the underlying distribution of the input data.
- Applications:
- Content Creation: Automating the generation of articles, marketing materials, and social media posts.
- Design: Assisting in product design by generating innovative concepts based on user preferences.
- Drug Discovery: Accelerating the identification of new compounds by simulating molecular interactions.
- Benefits:
- Increases productivity by automating repetitive tasks.
- Enhances creativity by providing novel ideas and solutions.
- Reduces time-to-market for products and services.
4.1. Enhancing Asset Allocation Strategies
Asset allocation is a crucial investment strategy that involves distributing investments across various asset classes to optimize risk and return. Generative AI can significantly enhance asset allocation strategies by providing data-driven insights and predictive analytics.
- Definition: Asset allocation is the process of dividing an investment portfolio among different asset categories, such as stocks, bonds, and cash.
- Importance:
- Balances risk and return based on individual investment goals.
- Helps in managing market volatility and economic changes.
- Role of Generative AI in Asset Allocation:
- Data Analysis: AI can analyze vast amounts of historical data to identify patterns and correlations among asset classes.
- Predictive Modeling: Generative AI can create models that predict future asset performance based on various economic indicators and market conditions.
- Scenario Simulation: AI can simulate different market scenarios to assess how various asset allocations would perform under different conditions, including scenario analysis in operational risk management and stress testing and scenario analysis in risk management.
- Benefits:
- Improved decision-making through data-driven insights.
- Enhanced risk management by identifying potential vulnerabilities in asset allocation.
- Increased efficiency in portfolio management by automating analysis and reporting.
- Practical Applications:
- Investment firms use AI to optimize portfolios by continuously analyzing market data and adjusting allocations in real-time.
- Robo-advisors leverage generative AI to provide personalized investment strategies based on individual risk tolerance and financial goals.
At Rapid Innovation, we leverage these advanced methodologies and technologies to help our clients achieve greater ROI. We even have ai solutions for merger and acquisition. By integrating scenario analysis and generative AI into your strategic planning and investment processes, including bnm climate risk management and scenario analysis, we empower you to make informed decisions, mitigate risks, and capitalize on emerging opportunities. Partnering with us means gaining access to cutting-edge solutions that enhance your operational efficiency and drive sustainable growth.
4.2. Leveraging AI for Economic Forecasting
- AI technologies are transforming the way economic forecasting is conducted.
- Machine learning algorithms can analyze vast datasets to identify patterns and trends that human analysts might miss.
- Predictive analytics can enhance the accuracy of economic models by incorporating real-time data.
- AI can process unstructured data, such as news articles and social media, to gauge public sentiment and its potential impact on the economy.
- By utilizing AI economic forecasting, organizations can:
- Improve the speed of data analysis.
- Enhance the precision of forecasts.
- Adapt to changing economic conditions more swiftly.
- AI-driven models can simulate various economic scenarios, helping policymakers and businesses prepare for potential outcomes.
- The integration of AI in economic forecasting can lead to more informed decision-making and strategic planning.
At Rapid Innovation, we empower our clients to harness these AI capabilities, enabling them to make data-driven decisions that enhance their operational efficiency and ultimately lead to greater ROI. For instance, a financial institution we partnered with utilized our AI-driven forecasting models to better predict market trends, resulting in a 20% increase in their investment returns over a fiscal year.
4.3. Developing Customized Investment Strategies
- AI enables the creation of tailored investment strategies that align with individual investor goals and risk tolerance.
- Algorithms can analyze historical performance data and current market conditions to recommend personalized investment portfolios.
- Key benefits of AI in investment strategy development include:
- Enhanced data analysis capabilities, allowing for the evaluation of multiple investment options simultaneously.
- Real-time adjustments to portfolios based on market fluctuations and emerging trends.
- The ability to incorporate alternative data sources, such as satellite imagery or social media sentiment, into investment decisions.
- AI can also facilitate robo-advisory services, providing automated investment advice at a lower cost.
- Investors can benefit from:
- Increased transparency in investment processes.
- Improved risk management through predictive analytics.
- Access to sophisticated tools that were previously available only to institutional investors.
By collaborating with Rapid Innovation, clients can leverage our expertise in AI to develop investment strategies that not only align with their financial goals but also adapt to market dynamics. For example, one of our clients saw a 30% improvement in their portfolio performance after implementing our customized AI-driven investment solutions.
5. Boosting Operational Efficiency with Generative AI
- Generative AI is revolutionizing operational efficiency across various industries by automating complex tasks.
- This technology can create content, design products, and even generate code, significantly reducing the time and resources required for these processes.
- Key applications of generative AI in enhancing operational efficiency include:
- Automating customer service through chatbots that can handle inquiries and provide support 24/7.
- Streamlining content creation for marketing and communications, allowing teams to focus on strategy rather than execution.
- Enhancing product design by generating multiple prototypes based on user specifications and preferences.
- Optimizing supply chain management by predicting demand and adjusting inventory levels accordingly.
- Benefits of implementing generative AI in operations include:
- Increased productivity as repetitive tasks are automated.
- Cost savings through reduced labor and operational expenses.
- Improved innovation by freeing up human resources to focus on creative and strategic initiatives.
- Organizations that leverage generative AI can gain a competitive edge by responding more quickly to market changes and customer needs.
At Rapid Innovation, we guide our clients in integrating generative AI into their operations, leading to significant cost reductions and enhanced productivity. For instance, a retail client we worked with reported a 40% decrease in operational costs after automating their customer service processes with our generative AI solutions.
Partnering with Rapid Innovation means not only adopting cutting-edge technology but also achieving measurable results that drive your business forward.
For further insights, you can explore our articles on AI agent societies and simulations.
5.1. Detecting and Preventing Fraud
Fraud detection and prevention are critical components of financial management and organizational integrity. Effective strategies can help organizations safeguard their assets and maintain trust with stakeholders.
- Implementing Internal Controls: Establishing robust internal controls can help prevent unauthorized access and fraudulent activities. This includes segregation of duties, regular audits, and access controls.
- Utilizing Technology: Advanced technologies such as machine learning and artificial intelligence can analyze patterns in data to identify anomalies that may indicate fraudulent behavior. These tools can flag unusual transactions for further investigation, allowing organizations to act swiftly and minimize losses. This is particularly relevant in areas like card fraud detection and credit fraud detection.
- Employee Training: Regular training sessions for employees on recognizing and reporting suspicious activities can enhance an organization’s fraud prevention efforts. Employees should be aware of the signs of fraud and the procedures for reporting them, fostering a proactive approach to fraud management. This is essential for effective fraud prevention solutions.
- Data Analytics: Analyzing transaction data can help identify trends and outliers. Organizations can use data analytics to monitor transactions in real-time, allowing for quicker responses to potential fraud and reducing the risk of financial loss. This is a key aspect of financial fraud detection and ecommerce fraud prevention.
- Whistleblower Policies: Encouraging a culture of transparency where employees feel safe to report suspicious activities can be effective. Whistleblower policies should protect individuals who report fraud from retaliation, creating an environment where integrity is prioritized. This is crucial in preventing and detecting fraud.
5.2. Modeling Volatility and Managing Risk
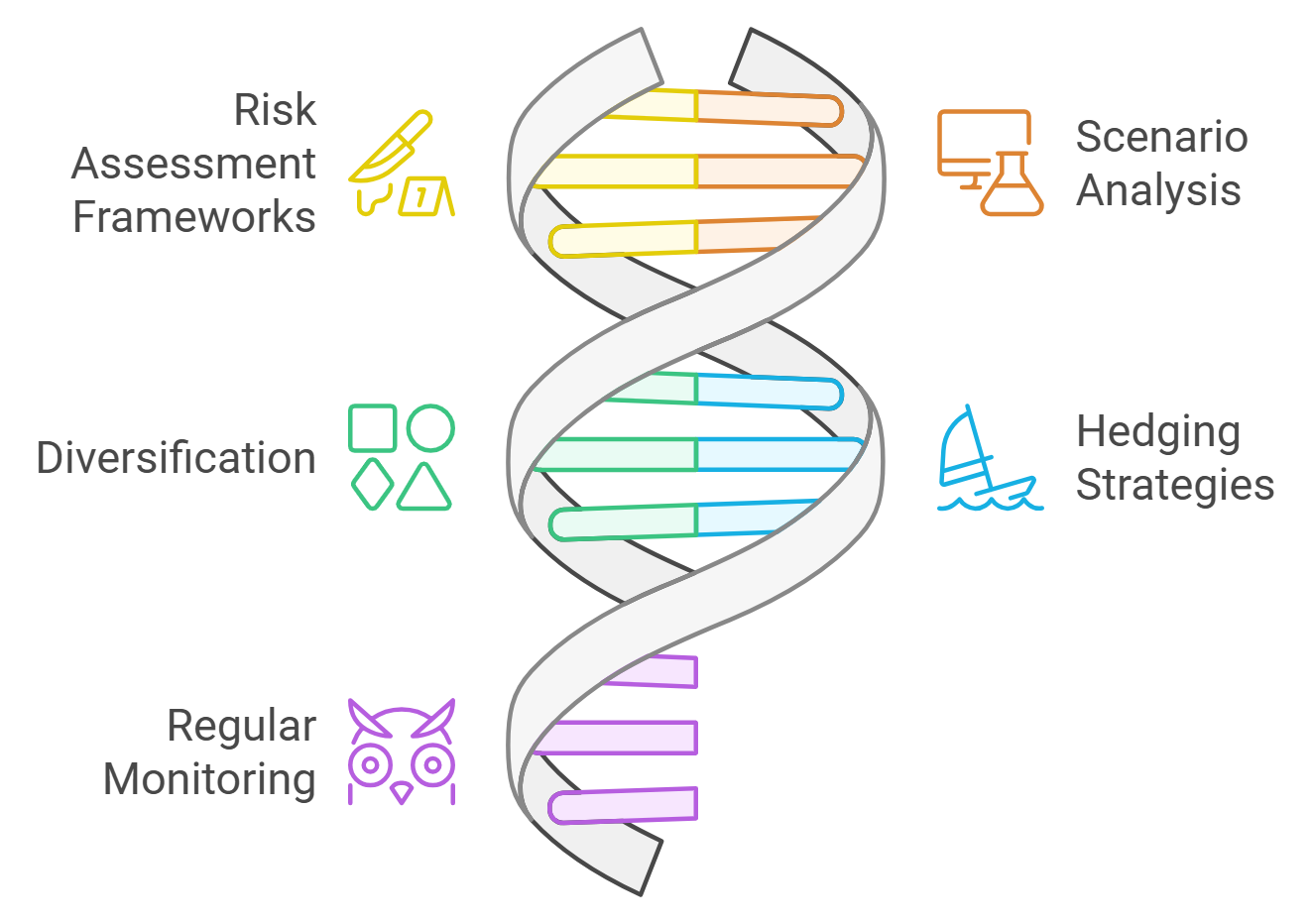
Understanding and managing volatility is essential for organizations to navigate uncertainties in the market. Effective risk management strategies can help mitigate potential losses.
- Risk Assessment Frameworks: Organizations should develop a comprehensive risk assessment framework that identifies, analyzes, and prioritizes risks. This framework should include both qualitative and quantitative assessments, ensuring a thorough understanding of potential threats.
- Scenario Analysis: Conducting scenario analysis allows organizations to model different market conditions and their potential impacts. This helps in understanding how various factors can affect volatility and overall risk, enabling informed decision-making.
- Diversification: Diversifying investments across different asset classes can reduce exposure to volatility. A well-diversified portfolio can help cushion against market fluctuations, enhancing financial stability.
- Hedging Strategies: Utilizing financial instruments such as options and futures can help manage risk. Hedging allows organizations to offset potential losses in one area with gains in another, providing a safety net during turbulent times.
- Regular Monitoring: Continuous monitoring of market conditions and performance metrics is crucial. Organizations should regularly review their risk management strategies and adjust them as necessary based on changing market dynamics, ensuring they remain agile and responsive.
5.3. Monitoring Performance and Conducting Market Research
Monitoring performance and conducting market research are vital for informed decision-making and strategic planning. These activities help organizations stay competitive and responsive to market changes.
- Key Performance Indicators (KPIs): Establishing KPIs allows organizations to measure performance against specific goals. KPIs should be relevant, measurable, and aligned with the organization’s objectives, providing clear benchmarks for success.
- Benchmarking: Comparing performance against industry standards or competitors can provide insights into areas for improvement. Benchmarking helps organizations identify best practices and set realistic performance targets, driving continuous improvement.
- Customer Feedback: Gathering and analyzing customer feedback can provide valuable insights into market trends and consumer preferences. Organizations should use surveys, focus groups, and GenAI can use for social media monitoring to collect this data, ensuring they remain attuned to customer needs.
- Market Trends Analysis: Regularly analyzing market trends helps organizations anticipate changes in consumer behavior and industry dynamics. This can involve studying economic indicators, competitor activities, and technological advancements, allowing for proactive strategy adjustments.
- Data-Driven Decision Making: Leveraging data analytics to inform strategic decisions can enhance performance monitoring. Organizations should utilize data visualization tools to present performance metrics clearly and effectively, facilitating informed discussions and actions.
By partnering with Rapid Innovation, clients can leverage our expertise in AI and blockchain technologies to implement these strategies effectively, ultimately achieving greater ROI and operational efficiency. Our tailored solutions not only enhance fraud detection and prevention but also empower organizations to make data-driven decisions that drive growth and innovation, including ecommerce fraud prevention companies and fraud detection tools.
6. Improving Client Interaction through AI
AI technologies are transforming how businesses interact with their clients. By leveraging AI, companies can enhance customer experiences, streamline communication, and provide timely support. Here are two key areas where AI is making a significant impact.
6.1. Automated Solutions for Customer Support
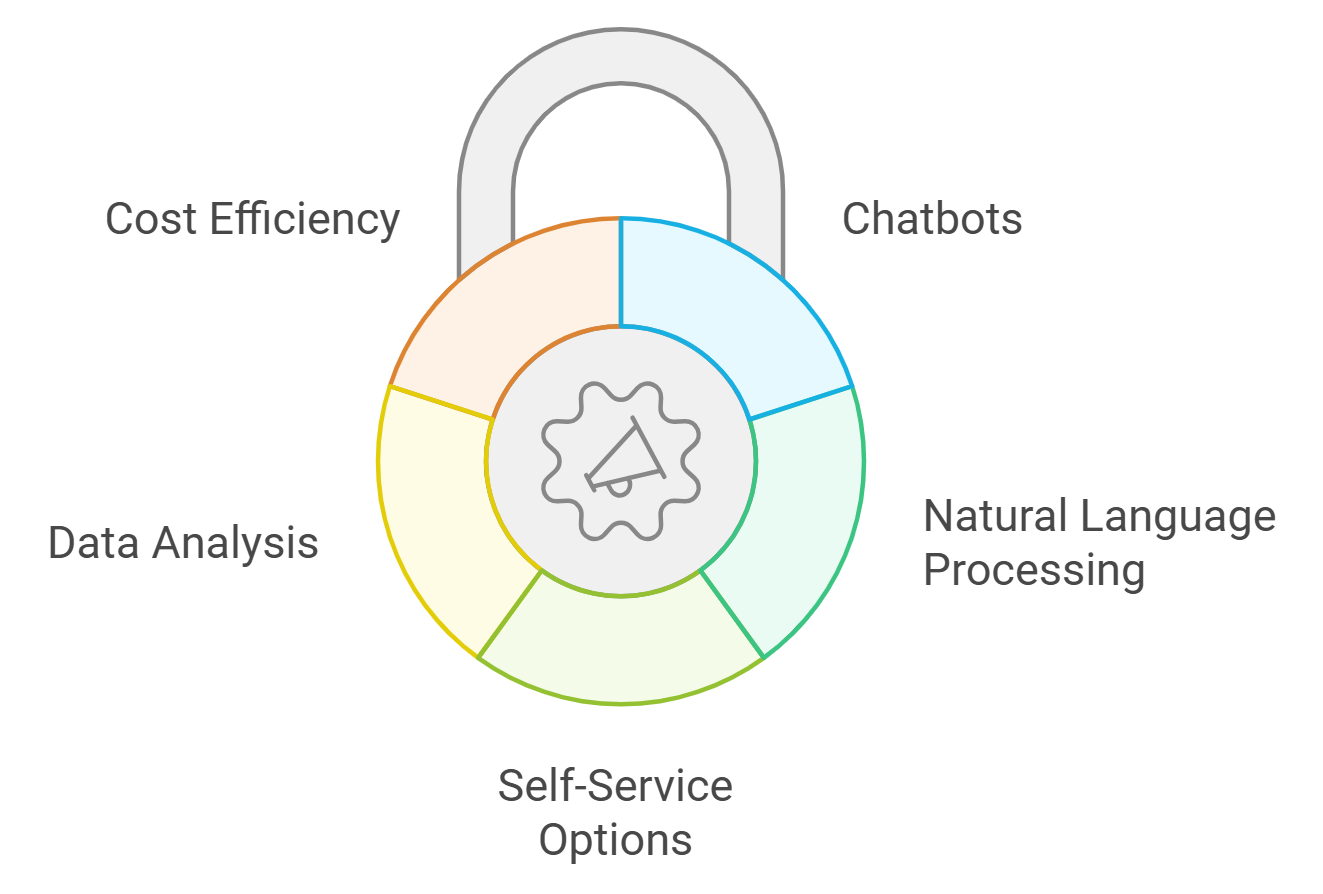
Automated solutions powered by AI are revolutionizing customer support by providing quick and efficient responses to client inquiries.
- Chatbots: AI-driven chatbots can handle multiple customer queries simultaneously, providing instant responses that reduce wait times for clients. They can operate 24/7, ensuring support is available at all times. This includes applications in ai customer service and ai customer support.
- Natural Language Processing (NLP): NLP allows chatbots to understand and respond to customer inquiries in a conversational manner. This technology helps in interpreting customer emotions and intent, leading to more personalized interactions, particularly in the context of conversational ai for customer service.
- Self-Service Options: AI can facilitate self-service portals where clients can find answers to common questions. These portals can include FAQs, video tutorials, and troubleshooting guides, empowering clients to resolve issues independently. This is especially relevant for ai customer care and artificial intelligence customer care.
- Data Analysis: AI systems can analyze customer interactions to identify common issues and trends. This data can help businesses improve their products and services based on customer feedback, enhancing the overall ai and customer service experience.
- Cost Efficiency: Automating customer support can significantly reduce operational costs. Businesses can allocate resources more effectively, focusing on complex issues that require human intervention, which is a key benefit of ai customer service software.
6.2. Enhancing Client Communication and Engagement
AI is also enhancing client communication and engagement, making interactions more meaningful and effective.
- Personalized Communication: AI can analyze customer data to tailor messages and offers to individual preferences. Personalized emails and recommendations can increase engagement and conversion rates, particularly in the realm of artificial intelligence and customer service.
- Predictive Analytics: AI tools can predict customer behavior based on historical data, allowing businesses to proactively reach out to clients with relevant offers or support before issues arise. This is crucial for ai in customer support.
- Omnichannel Support: AI enables seamless communication across various channels, including social media, email, and live chat. Clients can switch between channels without losing context, enhancing their overall experience, which is vital for customer care ai.
- Sentiment Analysis: AI can assess customer sentiment through social media and feedback forms. Understanding client emotions helps businesses adjust their strategies and improve satisfaction, a key aspect of ai helpdesk and helpdesk ai.
- Engagement Metrics: AI tools can track engagement metrics, such as response times and customer satisfaction scores. This data helps businesses refine their communication strategies and improve client relationships.
By integrating AI into customer support and communication strategies, businesses can create a more efficient, responsive, and personalized experience for their clients. At Rapid Innovation, we specialize in implementing these AI solutions to help our clients achieve greater ROI, streamline operations, and enhance customer satisfaction. Partnering with us means you can expect improved efficiency, reduced costs, and a more engaged customer base, ultimately driving your business success.
7. Managing ESG Factors with AI
Artificial Intelligence (AI) is transforming how organizations manage Environmental, Social, and Governance (ESG) factors. By leveraging AI technologies, companies can enhance their ESG strategies, ensuring compliance and integrating these criteria into their investment decisions.
7.1. Monitoring ESG Compliance
AI tools can significantly improve the monitoring of ESG compliance by automating data collection and analysis. This leads to more accurate and timely assessments of a company's adherence to ESG standards.
- Automated Data Collection: AI can gather data from various sources, including social media, news articles, and regulatory filings, to provide a comprehensive view of a company's ESG performance.
- Real-time Monitoring: AI systems can continuously track ESG metrics, allowing organizations to respond quickly to any compliance issues or changes in regulations.
- Predictive Analytics: By analyzing historical data, AI can predict potential ESG risks, enabling companies to take proactive measures to mitigate them.
- Enhanced Reporting: AI can streamline the reporting process by generating insights and visualizations that make it easier for stakeholders to understand ESG performance.
- Benchmarking: AI can compare a company's ESG performance against industry standards or competitors, helping identify areas for improvement.
7.2. Integrating ESG Criteria into Investment Strategies
AI can also play a crucial role in integrating ESG criteria into investment strategies, allowing investors to make more informed decisions that align with their values. AI algorithms can analyze vast amounts of data to identify companies that meet specific ESG criteria, helping investors select sustainable investment options. Additionally, AI can evaluate the potential risks associated with ESG factors, providing investors with a clearer understanding of how these elements may impact their portfolios.
- Data-Driven Insights: AI algorithms can analyze vast amounts of data to identify companies that meet specific ESG criteria, helping investors select sustainable investment options.
- Risk Assessment: AI can evaluate the potential risks associated with ESG factors, providing investors with a clearer understanding of how these elements may impact their portfolios.
- Portfolio Optimization: AI can assist in constructing portfolios that balance financial returns with ESG considerations, ensuring that investments align with sustainability goals.
- Scenario Analysis: AI can simulate various market conditions and their impact on ESG investments, helping investors understand potential outcomes and make better decisions.
- Engagement Strategies: AI can help investors develop engagement strategies with companies to encourage better ESG practices, fostering a culture of sustainability within the investment community.
By partnering with Rapid Innovation, clients can expect to achieve greater ROI through enhanced AI esg management. Our expertise in AI and blockchain development allows us to provide tailored solutions that streamline compliance, optimize investment strategies, and ultimately drive sustainable growth. With our support, organizations can not only meet regulatory requirements but also position themselves as leaders in responsible investing, ensuring long-term success in an increasingly conscientious market.
8. Supporting Decision-Making and Knowledge Management
8.1. AI-Driven Decision Support Systems

AI-driven decision support systems (DSS) leverage artificial intelligence to enhance the decision-making process across various sectors. These systems analyze vast amounts of data, providing insights that help organizations make informed choices.
- Data Analysis: AI algorithms can process and analyze large datasets quickly, identifying patterns and trends that may not be visible to human analysts. This capability allows organizations to make data-driven decisions that can lead to increased efficiency and profitability.
- Predictive Analytics: By utilizing historical data, AI can forecast future outcomes, enabling organizations to anticipate challenges and opportunities. For instance, a retail company can predict inventory needs, reducing excess stock and improving cash flow. Learn more about this in our article on predictive analytics in finance.
- Real-Time Insights: AI-driven DSS can provide real-time data analysis, allowing decision-makers to respond promptly to changing circumstances. This agility can be crucial in fast-paced industries, such as finance or e-commerce.
- Scenario Simulation: These systems can simulate different scenarios based on varying inputs, helping organizations evaluate potential outcomes before making decisions. This feature is particularly beneficial for strategic planning and risk management.
- Enhanced Collaboration: AI tools can facilitate collaboration among team members by providing a centralized platform for data sharing and analysis. This fosters a culture of teamwork and innovation, leading to better decision-making.
- Reduced Bias: AI can help minimize human biases in decision-making by relying on data-driven insights rather than subjective opinions. This objectivity can lead to more equitable and effective outcomes.
8.2. Generating and Managing Knowledge Insights
Knowledge management is crucial for organizations to retain and utilize information effectively. AI plays a significant role in generating and managing knowledge insights, ensuring that valuable information is accessible and actionable. AI-driven decision support systems can sift through vast amounts of unstructured data, extracting relevant information and insights that can inform decision-making.
- Knowledge Discovery: AI can sift through vast amounts of unstructured data, extracting relevant information and insights that can inform decision-making. This capability enables organizations to leverage their data assets fully.
- Automated Documentation: AI tools automate the documentation process, ensuring that knowledge is captured and stored systematically for future reference. This efficiency reduces the risk of knowledge loss and enhances organizational learning.
- Intelligent Search: Advanced AI algorithms enhance search capabilities, allowing users to find relevant information quickly and efficiently. This feature saves time and improves productivity across the organization.
- Personalized Learning: AI can tailor knowledge resources to individual users based on their roles and preferences, improving engagement and retention. This personalization fosters a culture of continuous learning and development.
- Collaboration Tools: AI-driven platforms can facilitate knowledge sharing among employees, breaking down silos and fostering a culture of collaboration. This interconnectedness can lead to innovative solutions and improved performance.
- Continuous Improvement: By analyzing feedback and outcomes, AI can help organizations refine their knowledge management processes, ensuring they remain effective and relevant. This commitment to improvement can drive long-term success and adaptability in a changing market.
By partnering with Rapid Innovation, clients can expect to achieve greater ROI through enhanced decision-making capabilities and effective knowledge management. Our expertise in AI-driven decision support systems and AI knowledge management in 2024 ensures that organizations can leverage cutting-edge technology to meet their goals efficiently and effectively.
9. How Rapid Innovation Enhances Asset Management with AI
Rapid innovation in artificial intelligence (AI) is transforming asset management by streamlining processes, improving decision-making, and enhancing overall efficiency. The integration of AI technologies allows asset managers to analyze vast amounts of data quickly, identify trends, and make informed investment decisions. This results in increased efficiency in data processing, enhanced predictive analytics for market trends, and improved risk management through real-time insights.
9.1. Features of Rapid Innovation’s AI Solutions

Rapid Innovation offers a range of AI solutions specifically designed for asset management, including ai inventory management software and digital asset management ai. These features help firms optimize their operations and improve investment outcomes.
- Data Integration: Seamlessly combines data from various sources, including market data, financial reports, and social media, providing a holistic view of asset performance and market conditions.
- Predictive Analytics: Utilizes machine learning algorithms to forecast market trends and asset performance, helping in identifying potential investment opportunities and risks.
- Automated Reporting: AI generates real-time reports and dashboards for stakeholders, reducing manual effort and increasing accuracy in reporting.
- Portfolio Optimization: Analyzes historical data to suggest optimal asset allocations, balancing risk and return based on individual investment goals.
- Sentiment Analysis: Monitors social media and news sentiment to gauge market sentiment, providing insights into public perception of assets and market conditions.
- Risk Assessment: Evaluates potential risks associated with investments using advanced algorithms, enabling proactive risk management strategies.
9.2. Case Studies: Optimizing Asset Management Workflows
Several case studies illustrate how Rapid Innovation's AI solutions, including ai digital asset management and ai asset management solutions, have optimized asset management workflows, leading to improved performance and efficiency.
- Case Study 1: Hedge Fund Performance Enhancement: A hedge fund implemented Rapid Innovation’s predictive analytics tools, resulting in a 20% increase in portfolio returns over a year. The fund was able to identify undervalued assets and adjust its strategy accordingly.
- Case Study 2: Real Estate Investment Optimization: A real estate investment firm used AI for market trend analysis, improving its property acquisition strategy and leading to a 15% reduction in acquisition costs. AI-driven insights helped the firm target high-growth areas more effectively.
- Case Study 3: Risk Management in Equity Investments: An asset management company adopted AI for risk assessment, reducing its exposure to high-risk assets by 30% through real-time risk monitoring. Enhanced decision-making processes led to a more resilient investment portfolio.
These case studies demonstrate the tangible benefits of integrating AI into asset management workflows, showcasing how Rapid Innovation's solutions can lead to significant improvements in performance and efficiency. By partnering with us, clients can expect enhanced operational efficiency, improved investment outcomes, and a greater return on investment, ultimately positioning them for long-term success in a competitive market.
For more insights on how AI is shaping various industries, check out our articles on AI Applications for loan underwriting. Additionally, explore AI in Everyday Use: Success Stories & Industry-Specific AI Case Studies to see real-world applications of AI technology.
10. Overcoming Challenges in Asset Management with AI
Asset management involves the systematic process of developing, operating, maintaining, and selling assets in a cost-effective manner. However, the industry faces several challenges that can hinder efficiency and profitability. At Rapid Innovation, we harness the power of Artificial Intelligence (AI) in investment management to offer innovative solutions to these challenges, transforming how asset management is conducted and helping our clients achieve their goals efficiently and effectively.
10.1. Common Workflow Challenges
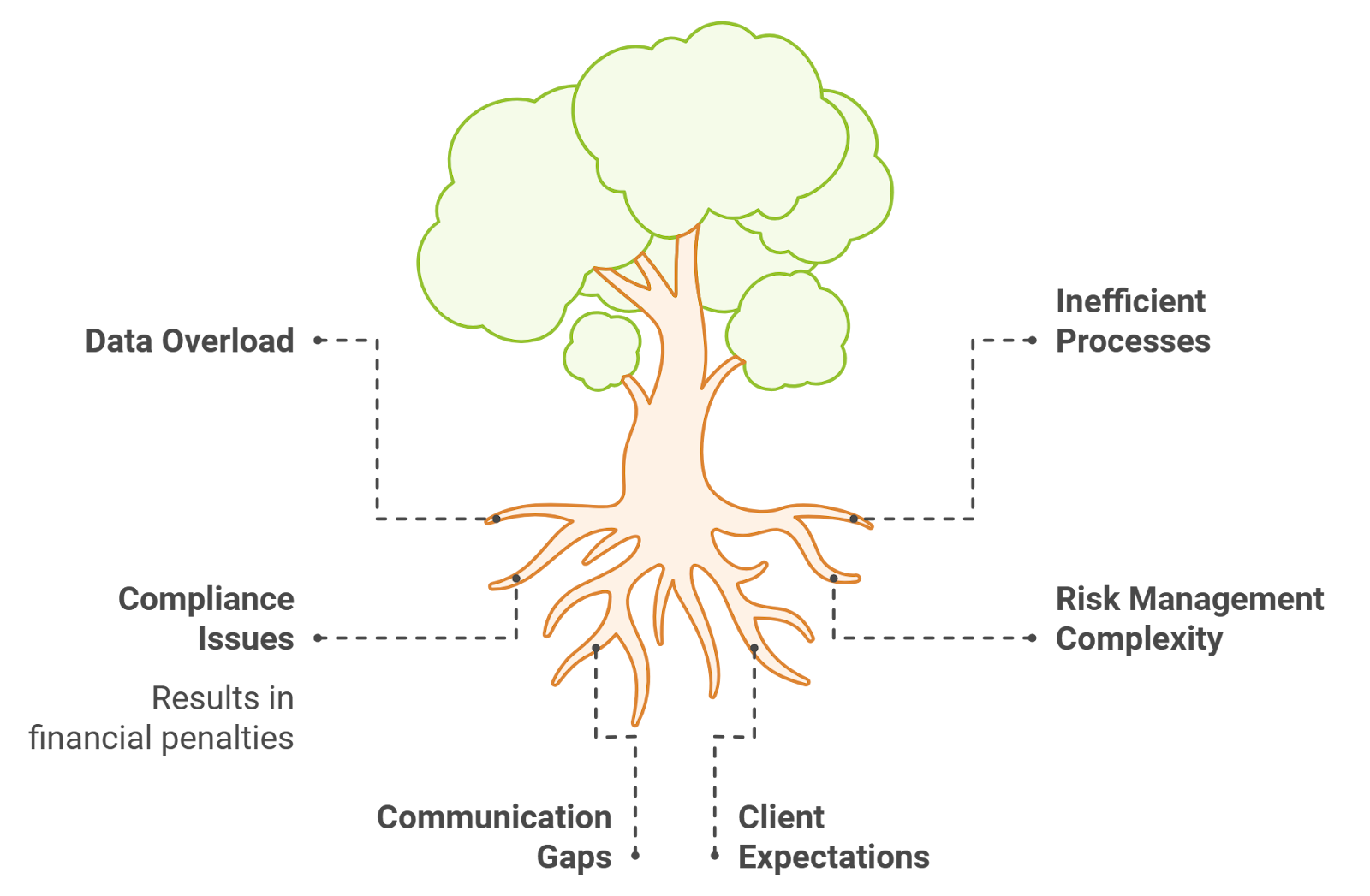
- Data Overload: Asset managers often deal with vast amounts of data from various sources, and the difficulty in filtering relevant information can lead to analysis paralysis.
- Inefficient Processes: Manual processes can be time-consuming and prone to human error, resulting in inefficiencies that delay decision-making and reduce responsiveness.
- Compliance and Regulatory Issues: Keeping up with changing regulations can be challenging, and non-compliance can result in significant financial penalties and reputational damage.
- Risk Management: Identifying and mitigating risks in asset portfolios is complex, as traditional methods may not adequately predict market fluctuations or asset performance.
- Communication Gaps: Poor communication between teams can lead to misalignment in goals and strategies, while a lack of real-time information sharing can hinder collaboration.
- Client Expectations: There are increasing demands for personalized services and transparency from clients, and difficulty in meeting these expectations can lead to client dissatisfaction.
10.2. AI Solutions to Address These Challenges
- Data Analytics and Management: AI can process and analyze large datasets quickly, identifying trends and insights. Machine learning for asset management can filter out noise, allowing managers to focus on relevant data.
- Automation of Processes: Robotic Process Automation (RPA) can streamline repetitive tasks, reducing human error. Automated reporting and compliance checks can save time and ensure accuracy.
- Enhanced Compliance Monitoring: AI tools can continuously monitor regulatory changes and assess compliance. Natural Language Processing (NLP) can analyze legal documents for compliance risks.
- Advanced Risk Assessment: AI models can predict market trends and assess risks more accurately. Predictive analytics can help in making informed investment decisions.
- Improved Communication Tools: AI-driven platforms can facilitate real-time communication and collaboration among teams. Chatbots and virtual assistants can provide instant information and support.
- Personalized Client Engagement: AI can analyze client data to offer tailored investment strategies. Predictive analytics can anticipate client needs and enhance service delivery.
By leveraging AI in asset management, firms can overcome these challenges, leading to improved efficiency, better risk management, and enhanced client satisfaction. Partnering with Rapid Innovation means you can expect greater ROI through streamlined operations, reduced costs, and enhanced decision-making capabilities. Our expertise in AI portfolio management and blockchain development ensures that you are equipped with the tools necessary to thrive in a competitive landscape. Let us help you navigate the complexities of asset management and achieve your business objectives with confidence.
For more insights on how AI is transforming the industry, check out the future of personalized risk evaluation in insurance with AI agents.
11. Requirements for Integrating AI into Asset Management
Integrating AI into asset management involves a comprehensive understanding of both technical and operational needs, as well as the development of effective strategies for successful implementation.
11.1. Technical and Operational Needs
- Data Infrastructure: Robust data management systems are essential for collecting, storing, and processing large volumes of data. The integration of various data sources, including market data, financial reports, and alternative data, is crucial.
- Advanced Analytics Tools: AI algorithms require sophisticated analytics tools for ai based financial data analysis and predictive modeling. These tools should support machine learning, natural language processing, and other AI techniques, including machine learning for asset management and AI in investment management.
- Skilled Workforce: A team with expertise in data science, machine learning, and finance is necessary to develop and maintain AI systems. Continuous training and upskilling of existing staff are important to keep pace with technological advancements, particularly in areas like AI portfolio management and AI fund manager roles.
- Regulatory Compliance: Understanding and adhering to financial regulations is critical when implementing AI solutions. Compliance frameworks should be established to ensure that AI applications, such as artificial intelligence asset management, meet legal and ethical standards.
- Cybersecurity Measures: Protecting sensitive financial data from cyber threats is paramount. Implementing robust cybersecurity protocols and conducting regular audits can help safeguard AI systems.
- Integration with Existing Systems: AI solutions must be compatible with existing asset management platforms and tools. Seamless integration minimizes disruption and enhances operational efficiency, especially for AI in asset management and asset management machine learning.
11.2. Strategies for Successful AI Integration
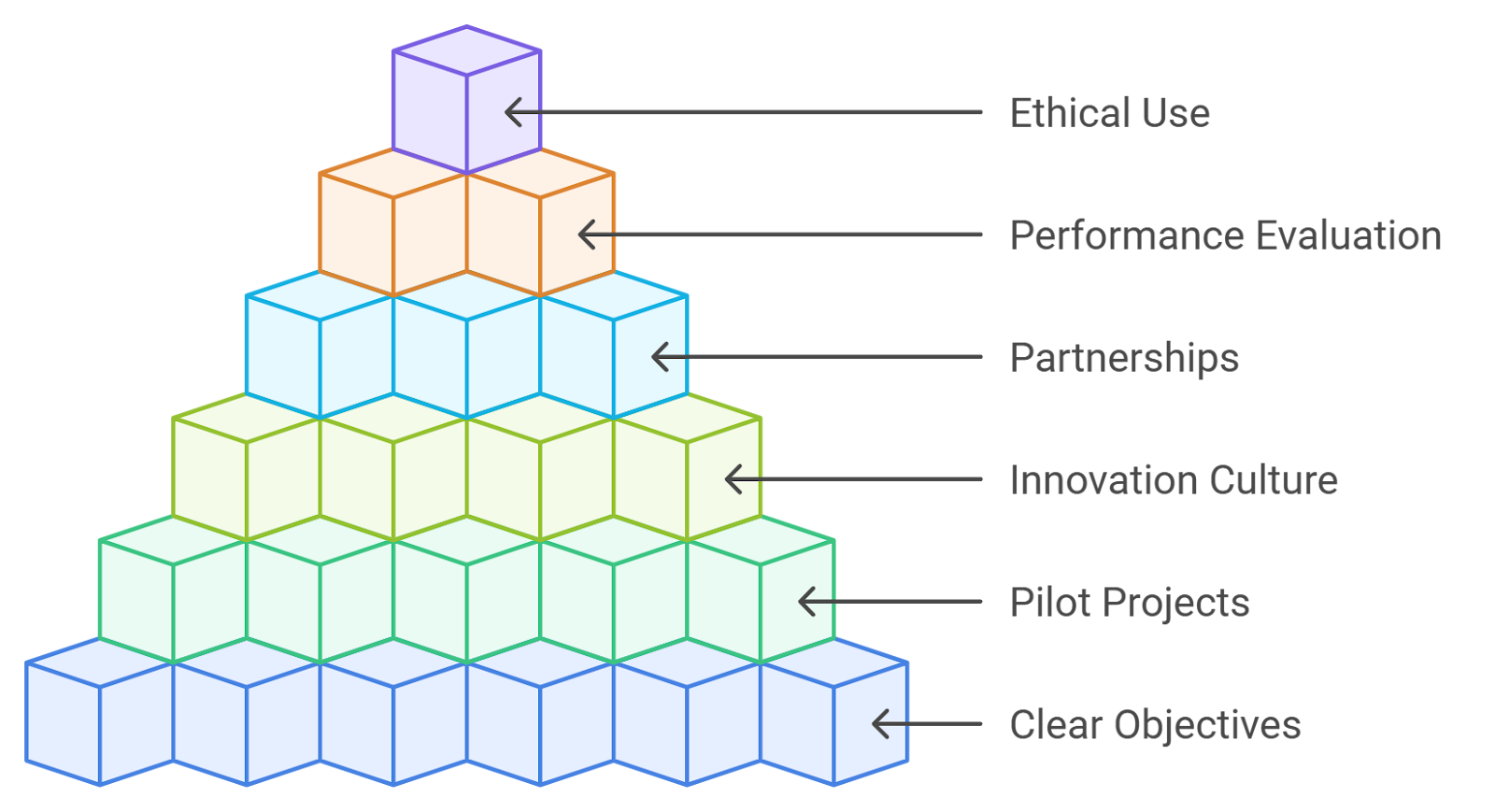
- Define Clear Objectives: Establish specific goals for AI implementation, such as improving investment performance or enhancing risk management. Clear objectives guide the development and deployment of AI solutions, including AI for investment management and AI for portfolio management.
- Start with Pilot Projects: Implementing AI through pilot projects allows for testing and refinement before full-scale deployment. These projects can help identify potential challenges and areas for improvement, particularly in AI based portfolio management.
- Foster a Culture of Innovation: Encourage a mindset that embraces change and innovation within the organization. Promoting collaboration between IT, data science, and investment teams can drive AI initiatives, including digital asset management AI and AI digital asset management.
- Leverage Partnerships: Collaborating with technology providers, data vendors, and academic institutions can enhance AI capabilities. Partnerships can provide access to cutting-edge technology and expertise, particularly in machine learning asset management.
- Monitor and Evaluate Performance: Establish metrics to assess the effectiveness of AI solutions in achieving business objectives. Regularly reviewing and adjusting strategies based on performance data and market changes is essential.
- Ensure Ethical AI Use: Develop guidelines for the ethical use of AI in asset management. Transparency in AI decision-making processes can build trust with clients and stakeholders.
At Rapid Innovation, we understand that the integration of AI into asset management is not just about technology; it's about creating value and achieving greater ROI for our clients. By partnering with us, you can expect a tailored approach that aligns with your specific goals, ensuring that your investment in AI translates into measurable success. Our expertise in both AI and blockchain technologies positions us uniquely to help you navigate this complex landscape, ultimately driving efficiency and effectiveness in your operations. For more information on how we can assist you, visit our AI Strategy Consulting page.
12. Implementing Generative AI in Asset Management
Generative AI in asset management is transforming the industry by enhancing decision-making, optimizing portfolios, and improving operational efficiency. Its implementation requires careful planning and execution to maximize benefits.
12.1. Effective Implementation Steps
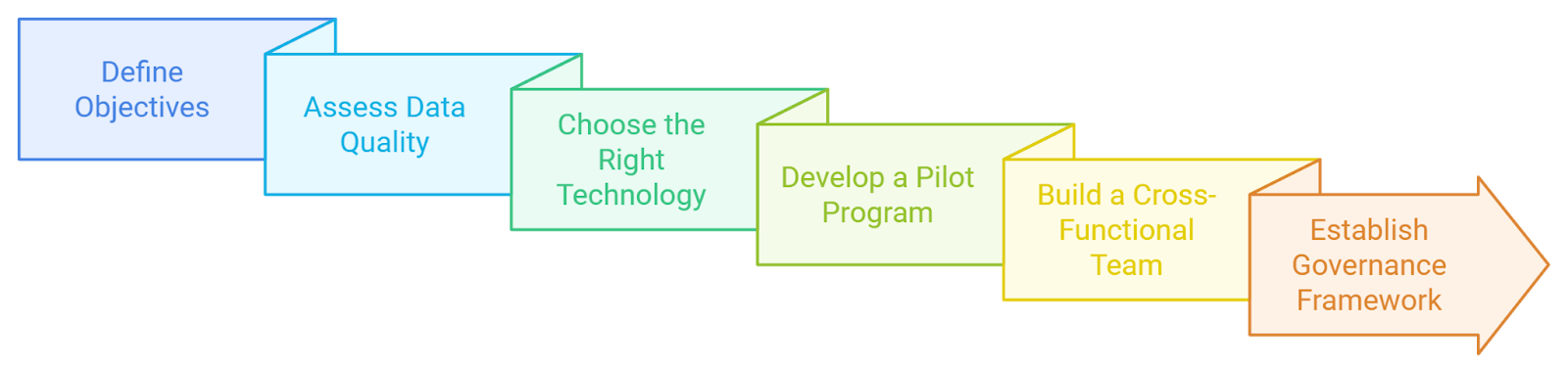
- Define Objectives: Clearly outline what you aim to achieve with generative AI in asset management, considering goals such as improving risk assessment, enhancing customer service, or automating reporting.
- Assess Data Quality: Evaluate the quality and availability of data needed for AI models. Ensure data is clean, relevant, and comprehensive to support accurate predictions.
- Choose the Right Technology: Select appropriate AI tools and platforms that align with your objectives. Consider factors like scalability, integration capabilities, and user-friendliness.
- Develop a Pilot Program: Start with a small-scale pilot to test the AI model in a controlled environment. Monitor performance and gather feedback to refine the approach before full-scale implementation.
- Build a Cross-Functional Team: Assemble a team with diverse skills, including data scientists, financial analysts, and IT professionals. Encourage collaboration to ensure all perspectives are considered in the implementation process.
- Establish Governance Framework: Create guidelines for ethical AI use, data privacy, and compliance with regulations. Ensure accountability and transparency in AI decision-making processes.
- Train and Educate Staff: Provide training for employees on how to use AI tools effectively. Foster a culture of continuous learning to keep pace with technological advancements.
- Monitor and Evaluate Performance: Regularly assess the performance of AI models against predefined metrics. Use insights gained to make necessary adjustments and improvements.
12.2. Best Practices for AI Integration
- Start Small and Scale Gradually: Begin with specific use cases that can demonstrate quick wins and gradually expand AI applications as confidence and expertise grow.
- Focus on User Experience: Design AI interfaces that are intuitive and user-friendly, ensuring that end-users can easily interact with AI tools without extensive technical knowledge.
- Leverage Existing Infrastructure: Integrate AI solutions with existing systems to minimize disruption and ensure compatibility with current data management and analytics platforms.
- Encourage Collaboration: Foster a collaborative environment between IT and business units, encouraging the sharing of insights and best practices across departments.
- Prioritize Security and Compliance: Implement robust security measures to protect sensitive data and stay updated on regulatory requirements to ensure compliance in AI applications.
- Utilize Feedback Loops: Establish mechanisms for continuous feedback from users and stakeholders, using this feedback to refine AI models and improve overall effectiveness.
- Stay Informed on AI Trends: Keep abreast of the latest developments in AI technology and methodologies by attending industry conferences, webinars, and training sessions to enhance knowledge.
- Measure ROI: Regularly assess the return on investment from AI initiatives, using metrics such as cost savings, increased efficiency, and improved decision-making to evaluate success.
At Rapid Innovation, we understand the complexities involved in implementing AI in asset management development. Our expertise in AI and blockchain development allows us to guide clients through each step of the process, ensuring that they achieve their objectives efficiently and effectively. By partnering with us, clients can expect enhanced decision-making capabilities, optimized portfolio management, and improved operational efficiency, ultimately leading to greater ROI. Our tailored solutions and commitment to excellence empower organizations to harness the full potential of generative AI in asset management, driving innovation and success in the competitive landscape.
13. Benefits of Generative AI in Asset Management
Generative AI in asset management is transforming the industry by providing innovative solutions that enhance operational efficiency, improve risk management, and support better decision-making. Here are the key benefits:
13.1. Enhancing Efficiency and Accuracy
Generative AI can significantly streamline processes in asset management, leading to increased efficiency and accuracy.
- Automation of Routine Tasks: Generative AI can automate repetitive tasks such as data entry, report generation, and compliance checks, allowing asset managers to focus on more strategic activities and improving overall productivity.
- Data Analysis and Insights: AI algorithms can analyze vast amounts of data quickly and accurately, identifying trends and patterns that may not be visible to human analysts. This capability enhances the quality of insights derived from data, leading to more informed investment decisions.
- Enhanced Portfolio Management: Generative AI can optimize portfolio allocations by simulating various market scenarios and predicting potential outcomes. This results in more precise asset allocation strategies, reducing the likelihood of human error.
- Real-Time Monitoring: AI systems can continuously monitor market conditions and asset performance, providing real-time updates and alerts. This enables asset managers to react swiftly to market changes, ensuring that investment strategies remain aligned with current conditions.
- Cost Reduction: By automating processes and improving accuracy, generative AI can lead to significant cost savings in operational expenses. Reduced reliance on manual processes minimizes the risk of costly errors and inefficiencies.
13.2. Improving Risk Management and Decision-Making
Generative AI plays a crucial role in enhancing risk management practices and supporting better decision-making in asset management.
- Advanced Risk Assessment: AI models can evaluate a wide range of risk factors, including market volatility, credit score risk, and liquidity risk. This comprehensive risk assessment allows asset managers to identify potential threats and take proactive measures.
- Scenario Analysis and Stress Testing: Generative AI can simulate various economic scenarios to assess how different factors might impact asset performance. This helps in understanding potential vulnerabilities and preparing for adverse market conditions.
- Predictive Analytics: AI-driven predictive analytics can forecast market trends and asset performance based on historical data and current indicators. This foresight aids in making timely investment decisions and adjusting strategies accordingly.
- Enhanced Decision-Making Framework: Generative AI can provide data-driven recommendations, helping asset managers make more informed choices. By integrating AI insights into the decision-making process, firms can reduce biases and improve the quality of their investment strategies.
- Regulatory Compliance: AI can assist in ensuring compliance with regulatory requirements by monitoring transactions and flagging any anomalies. This reduces the risk of regulatory breaches and associated penalties, enhancing the overall governance of asset management practices.
- Improved Client Engagement: Generative AI can analyze client preferences and behaviors, allowing asset managers to tailor their services more effectively. This personalized approach can lead to better client satisfaction and retention, ultimately benefiting the firm’s bottom line.
By partnering with Rapid Innovation, clients can leverage these benefits of generative AI in asset management to achieve greater ROI, streamline their operations, and enhance their decision-making capabilities. Our expertise in AI and blockchain development ensures that we provide tailored solutions that align with your specific goals, driving efficiency and effectiveness in your asset management practices.
14. Generative AI Models for Asset Management
Generative AI models for asset management are increasingly being utilized to enhance decision-making, optimize portfolios, and improve risk management. These models leverage vast amounts of data to generate insights and predictions that can significantly impact investment strategies.
14.1. Overview of Popular AI Models
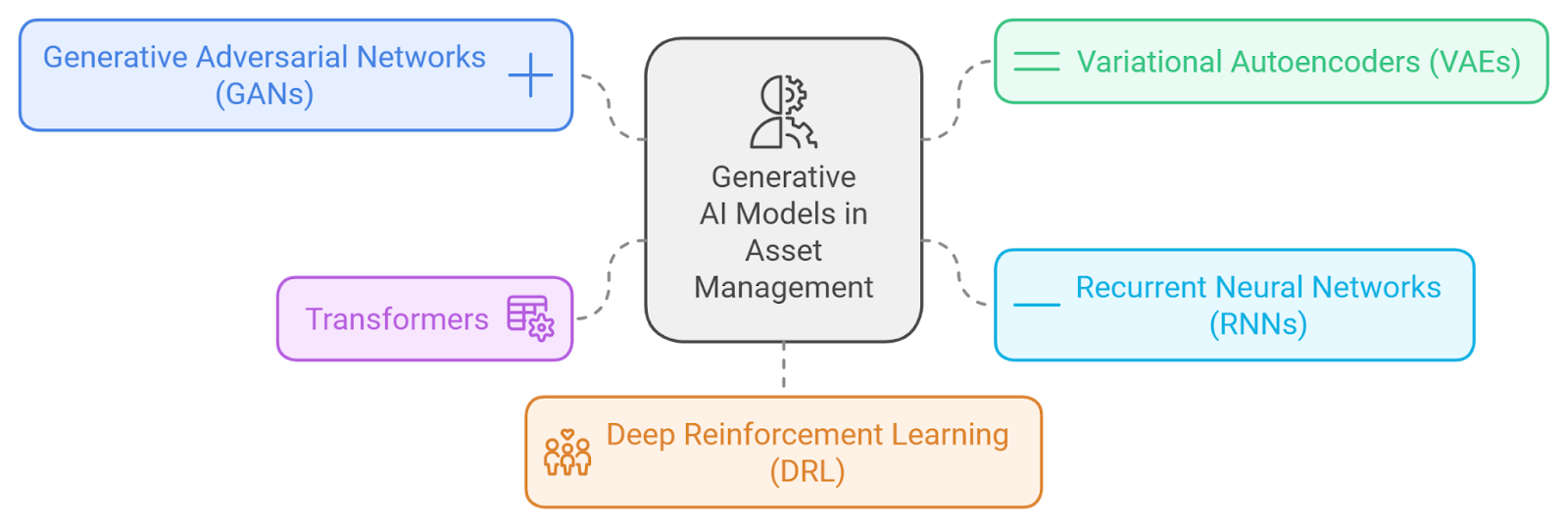
Several generative AI models are gaining traction in the asset management sector. Here are some of the most notable:
- Generative Adversarial Networks (GANs): These models comprise two neural networks, a generator and a discriminator, that work against each other. They are useful for generating synthetic financial data, which can help in stress testing and scenario analysis. Learn more about Generative Adversarial Networks (GANs).
- Variational Autoencoders (VAEs): This type of neural network learns to encode data into a lower-dimensional space and then reconstructs it. VAEs can be used for anomaly detection in financial transactions and portfolio optimization.
- Recurrent Neural Networks (RNNs): Designed for sequential data, RNNs are ideal for time-series forecasting in financial markets. They are effective in predicting stock prices and market trends based on historical data.
- Transformers: Originally developed for natural language processing, Transformers are now being adapted for financial data analysis. They are capable of handling large datasets and capturing complex relationships, making them suitable for risk assessment and portfolio management. To understand more about Transformers, check out what a transformer model is.
- Deep Reinforcement Learning (DRL): This approach combines deep learning with reinforcement learning principles to make decisions based on rewards. DRL is used for developing trading strategies that adapt to changing market conditions.
14.2. Comparative Analysis of AI Models
When comparing generative AI models for asset management, several factors come into play:
- Data Requirements: GANs and VAEs require large datasets for training, which can be a limitation in niche markets. In contrast, RNNs and Transformers can handle smaller datasets more effectively, making them more versatile.
- Complexity: GANs are complex and can be challenging to train due to issues like mode collapse. VAEs, on the other hand, are generally easier to train but may not capture the full complexity of the data.
- Interpretability: RNNs and Transformers can be less interpretable, making it difficult for asset managers to understand model decisions. VAEs offer better interpretability, which is crucial for regulatory compliance and risk management.
- Performance: GANs excel in generating high-quality synthetic data, which can enhance model training. RNNs are particularly strong in time-series predictions, while Transformers have shown superior performance in capturing long-range dependencies in data.
- Use Cases: GANs are often used for generating synthetic market scenarios, while VAEs are more suited for anomaly detection. RNNs are ideal for forecasting, and Transformers are increasingly being used for comprehensive risk assessments.
- Scalability: Transformers are highly scalable and can be deployed across various asset classes. However, GANs and VAEs may face challenges in scaling due to their complexity and data requirements.
In conclusion, the choice of generative AI model in asset management depends on specific use cases, data availability, and the desired balance between complexity and interpretability. Each model has its strengths and weaknesses, making it essential for asset managers to carefully evaluate their options based on their unique needs and objectives.
At Rapid Innovation, we understand the intricacies of generative AI models for asset management and their applications. By partnering with us, clients can leverage our expertise to implement the most suitable AI solutions tailored to their specific investment strategies. Our commitment to delivering efficient and effective solutions ensures that clients can achieve greater ROI while navigating the complexities of the financial landscape. With our support, clients can expect enhanced decision-making capabilities, optimized portfolio performance, and improved risk management, ultimately driving their success in the competitive asset management arena. Additionally, the critical role of data quality in AI implementations cannot be overlooked; for more information, visit the critical role of data quality in AI implementations.
15. Emerging Trends in Generative AI for Asset Management
Generative AI is transforming various sectors, and asset management is no exception. The integration of this technology is leading to innovative solutions that enhance decision-making, risk management, and operational efficiency. By 2025, it's estimated that 95% of all customer interactions in banking and asset management will be AI-powered. Generative AI is predicted to reduce operational costs in asset management by up to 40% by 2030, leading to more competitive pricing for investors.
15.1. Innovations on the Horizon
- Enhanced Data Analysis: Generative AI can process vast amounts of data quickly, identifying patterns and trends that human analysts might miss. This capability allows for more accurate forecasting and investment strategies, ultimately leading to greater returns on investment.
- Personalized Investment Strategies: AI can create tailored investment portfolios based on individual client preferences and risk tolerance. By analyzing client data, generative AI can suggest optimal asset allocations that align with personal financial goals, ensuring that clients achieve their desired outcomes.
- Automated Reporting: Generative AI can automate the generation of reports, saving time and reducing human error. This innovation allows asset managers to focus on strategic decision-making rather than administrative tasks, enhancing overall productivity.
- Risk Management Solutions: AI can simulate various market scenarios to assess potential risks and returns. This predictive capability helps asset managers make informed decisions and mitigate risks effectively, safeguarding client investments.
- Natural Language Processing (NLP): NLP can analyze news articles, social media, and other text data to gauge market sentiment. This insight can inform investment strategies and enhance market understanding, providing a competitive edge.
- Regulatory Compliance: Generative AI can assist in monitoring compliance with regulations by analyzing transactions and flagging anomalies. This reduces the burden on compliance teams and enhances overall governance, ensuring that firms operate within legal frameworks.
15.2. Future Impact on the Asset Management Industry
- Increased Efficiency: The automation of routine tasks will lead to significant time savings for asset managers. This efficiency allows firms to allocate resources more effectively and focus on high-value activities, ultimately driving better results.
- Enhanced Decision-Making: With access to advanced analytics and predictive modeling, asset managers can make more informed decisions, leading to better investment outcomes and improved client satisfaction.
- Competitive Advantage: Firms that adopt generative AI early will likely gain a competitive edge in the market. The ability to leverage generative AI in asset management for insights and automation can differentiate firms in a crowded landscape, attracting more clients.
- Cost Reduction: By automating processes and improving efficiency, generative AI can help reduce operational costs. This cost-effectiveness can lead to better pricing for clients and improved profit margins for firms, enhancing overall profitability.
- Talent Transformation: The role of asset managers will evolve, requiring new skills in data analysis and AI technology. Firms will need to invest in training and development to equip their teams for this shift, ensuring they remain competitive.
- Ethical Considerations: As AI becomes more prevalent, ethical considerations around data usage and decision-making will come to the forefront. Asset management firms will need to establish guidelines to ensure responsible AI use, fostering trust with clients.
- Market Dynamics: The integration of generative AI may lead to changes in market dynamics, influencing how assets are valued and traded. This could result in new investment opportunities and challenges for traditional asset management practices, necessitating adaptability and innovation.
By partnering with Rapid Innovation, clients can leverage these emerging trends in generative AI in asset management to enhance their asset management strategies, achieve greater ROI, and stay ahead in a rapidly evolving market. Our expertise in AI and blockchain development ensures that we provide tailored solutions that meet the unique needs of each client, driving efficiency and effectiveness in their operations.
For more insights, check out Generative AI in Insurance: An Overview, Transforming Industries: The Impact of Generative.