Table Of Contents
Category
Artificial Intelligence
FinTech
1. What is AI-Based Credit Scoring?
AI-Based Credit Scoring refers to the use of artificial intelligence technologies to assess the creditworthiness of individuals or businesses. Traditional credit scoring models rely on historical data and predefined criteria, while AI-based systems leverage machine learning algorithms to analyze vast amounts of data, identify patterns, and make predictions about credit risk. This approach aims to provide a more accurate and nuanced understanding of a borrower's financial behavior.
- Utilizes advanced algorithms to evaluate credit risk.
- Incorporates a wider range of data sources beyond traditional credit reports.
- Aims to improve accuracy and reduce bias in credit assessments.
Traditional credit scoring models typically rely on a limited set of factors, often missing up to 70% of potentially relevant data points. According to the Consumer Financial Protection Bureau, about 45 million Americans are "credit invisible" or have insufficient credit histories to generate a traditional credit score.
1.1. Understanding AI in Credit Scoring
AI in credit scoring involves the application of machine learning, natural language processing, and data analytics to enhance the evaluation of creditworthiness. These technologies enable lenders to process and analyze data more efficiently and effectively.
- Machine Learning: Algorithms learn from historical data to predict future behavior.
- Natural Language Processing: Analyzes unstructured data, such as social media activity or transaction descriptions.
- Data Analytics: Combines various data sources, including alternative data, to create a comprehensive profile of the borrower.
AI systems can assess factors such as payment history, income stability, spending habits, and social media behavior. This holistic approach allows lenders to make more informed decisions, especially for individuals with limited credit histories.
1.2. How AI Transforms the Credit Evaluation Process
AI is revolutionizing the credit evaluation process in several key ways:
- Enhanced Data Analysis: AI can process large datasets quickly, identifying trends and correlations that traditional methods might miss.
- Real-Time Decision Making: AI systems can provide instant credit decisions, improving the customer experience and reducing wait times.
- Reduced Bias: AI can help mitigate human biases by relying on data-driven insights rather than subjective judgments.
- Predictive Analytics: AI models can forecast future credit behavior based on historical patterns, allowing lenders to assess risk more accurately.
The transformation includes greater access to credit for underserved populations, more personalized lending options based on individual risk profiles, and improved fraud detection through anomaly detection algorithms.
Overall, AI-based credit scoring is reshaping how lenders evaluate creditworthiness, making the process more efficient, fair, and inclusive.
At Rapid Innovation, we understand the complexities of integrating AI-based credit scoring into your business model. Our expertise in building AI based banking and finance solutions allows us to tailor solutions that not only enhance your credit evaluation processes but also drive greater ROI. By partnering with us, you can expect:
- Increased Efficiency: Our AI solutions streamline data processing, enabling faster decision-making and reducing operational costs.
- Enhanced Accuracy: With advanced algorithms, we help you achieve more precise credit assessments, minimizing risk and maximizing profitability.
- Access to New Markets: Our innovative approaches, including ai credit scoring models and ai credit rating, can help you reach underserved populations, expanding your customer base and increasing revenue potential.
- Data-Driven Insights: We provide actionable analytics that empower you to make informed lending decisions, ultimately improving your bottom line.
Refer to the image for a visual representation of AI-Based Credit Scoring concepts:

2. Differences Between Traditional Credit Scoring and AI-Based Credit Scoring
2.1. Key Distinctions in Data Analysis and Accuracy
Traditional credit scoring relies on a limited set of data points, primarily focusing on credit history, payment history, outstanding debts, length of credit history, and types of credit used. In contrast, AI-based credit scoring utilizes a broader range of data sources, including social media activity, online behavior, utility payment records, employment history, and alternative data from non-traditional sources.
Accuracy in credit scoring is significantly enhanced with AI due to machine learning algorithms that can identify complex patterns in large datasets, continuous learning from new data that allows for real-time updates and adjustments, and improved predictive capabilities that lead to better risk assessment. Traditional models often struggle with accuracy, particularly for individuals with limited credit history (thin files) and new immigrants or young adults without established credit records.
2.2. The Benefits of AI Over Traditional Models
- Enhanced Predictive Power: AI algorithms can analyze vast amounts of data quickly and efficiently, identifying subtle correlations that traditional models may overlook, as seen in ai for credit scoring.
- Real-Time Decision Making: AI systems can process applications and provide credit decisions almost instantaneously, improving customer experience and satisfaction.
- Personalization: AI can tailor credit offers based on individual behavior and preferences, leading to better customer engagement and loyalty.
- Reduced Bias: AI can be designed to minimize human biases that may affect traditional scoring. By focusing on data-driven insights, AI can promote fairer lending practices, including ai credit rating.
- Cost Efficiency: Automating the credit scoring process with AI can reduce operational costs for lenders, which can lead to lower interest rates for consumers.
- Continuous Improvement: AI systems can adapt and improve over time as they learn from new data, ensuring that credit scoring remains relevant in changing economic conditions.
- Broader Access to Credit: AI can assess creditworthiness for individuals who may not qualify under traditional models, helping to expand financial services to a larger segment of the population, as demonstrated by credit scoring using ai.
At Rapid Innovation, we leverage these advancements in AI and blockchain technology to help our clients enhance their credit scoring systems. By integrating AI-based solutions, we enable our clients to achieve greater ROI through improved accuracy, efficiency, and customer satisfaction. Partnering with us means you can expect a transformative approach to credit scoring that not only meets the demands of today's market but also positions you for future growth.
Refer to the image for a visual representation of the differences between traditional credit scoring and AI-based credit scoring.
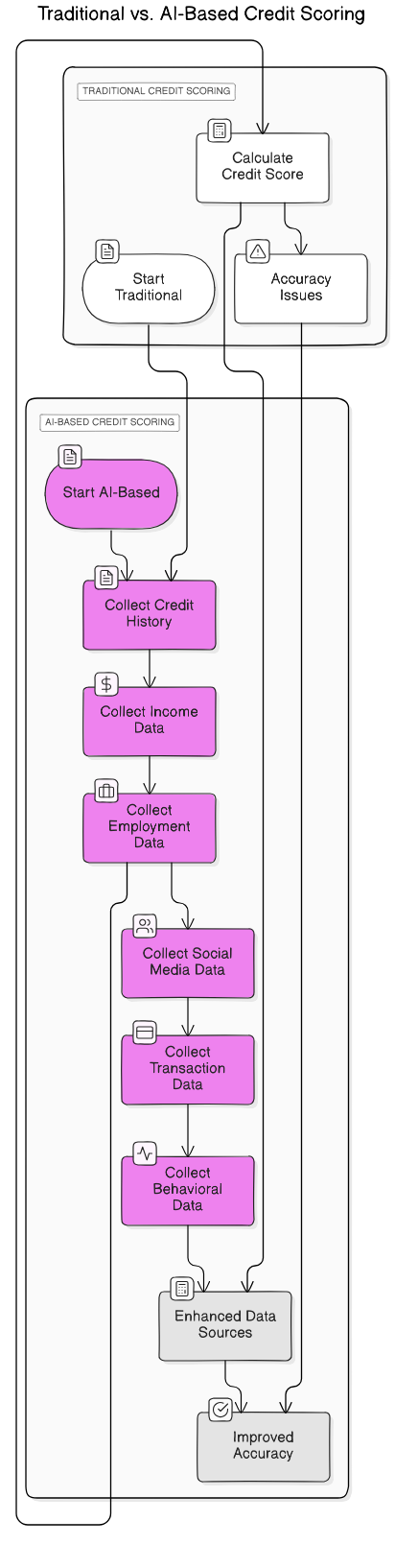
3. How Does AI-Based Credit Scoring Work?
AI-based credit scoring leverages advanced algorithms and machine learning techniques to assess an individual's creditworthiness. This approach enhances traditional credit scoring methods by incorporating a wider range of data and providing more accurate predictions of a borrower's likelihood to repay a loan. Traditional credit scoring often relies on a limited set of factors, such as payment history, credit utilization, and length of credit history. In contrast, AI-based systems can analyze vast amounts of data, including alternative data sources, to create a more comprehensive profile of a borrower. The use of AI can lead to faster decision-making processes and potentially lower costs for lenders.
3.1. AI Algorithms Used in Credit Scoring
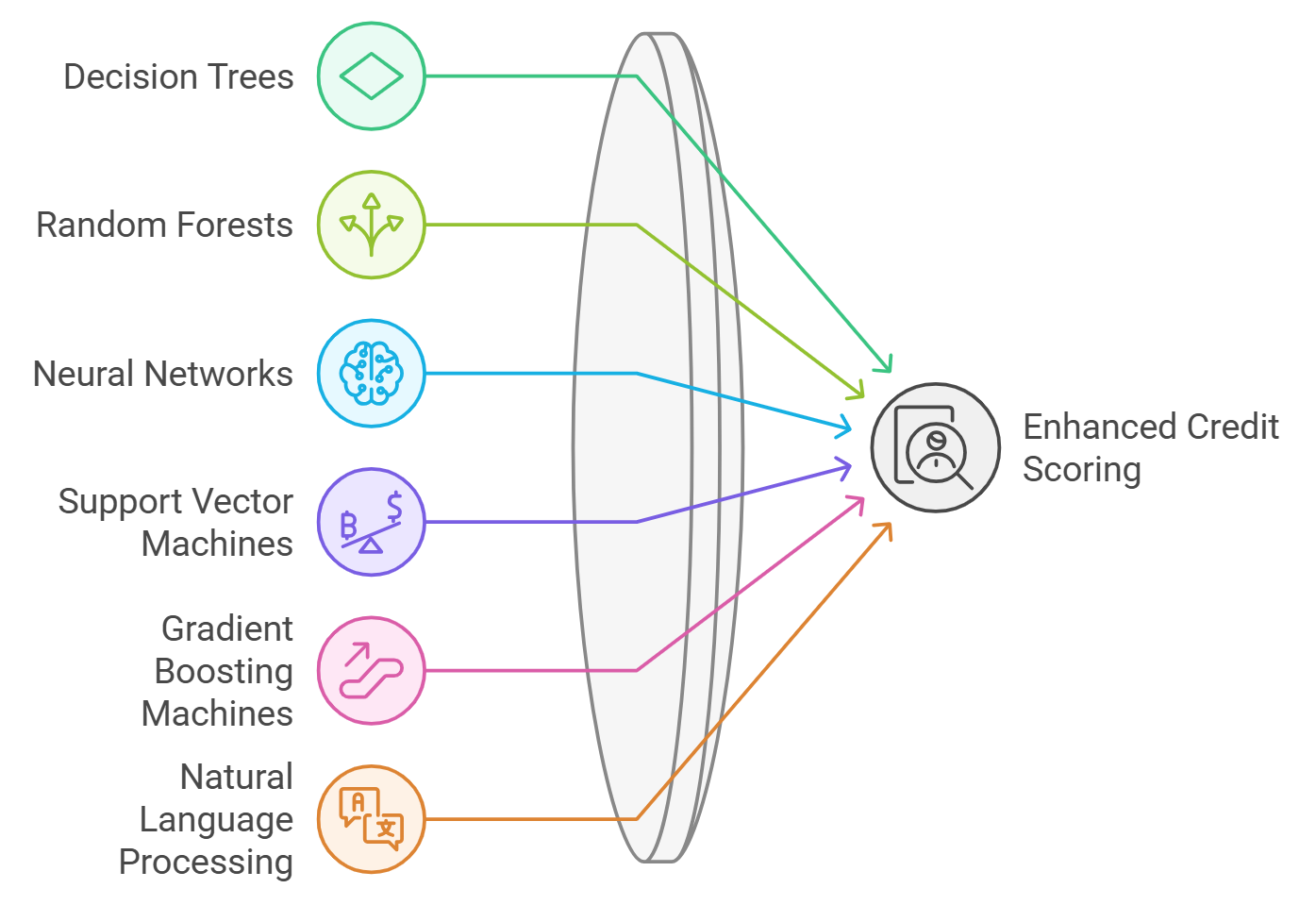
Various AI algorithms are employed in credit scoring to improve accuracy and efficiency. Some of the most common algorithms include:
- Decision Trees: These algorithms break down data into branches based on decision rules, making it easier to visualize and understand the factors influencing creditworthiness.
- Random Forests: An extension of decision trees, random forests use multiple trees to improve prediction accuracy by averaging the results, reducing the risk of overfitting.
- Neural Networks: These algorithms mimic the human brain's structure and function, allowing for complex pattern recognition in large datasets. They are particularly effective in identifying non-linear relationships between variables.
- Support Vector Machines (SVM): SVMs are used to classify data points into different categories, helping to distinguish between high-risk and low-risk borrowers.
- Gradient Boosting Machines (GBM): GBMs build models in a sequential manner, where each new model corrects the errors of the previous ones, leading to improved predictive performance.
- Natural Language Processing (NLP): NLP techniques can analyze unstructured data, such as social media activity or customer reviews, to gain insights into a borrower's behavior and reliability.
3.2. The Process of Analyzing Creditworthiness with AI
The process of analyzing creditworthiness using AI involves several key steps:
- Data Collection: AI systems gather data from various sources, including traditional credit reports, bank statements, social media, and even utility payment histories. This comprehensive data collection helps create a more accurate borrower profile, which is essential for AI credit scoring.
- Data Preprocessing: The collected data is cleaned and transformed to ensure consistency and accuracy. This step may involve handling missing values, normalizing data, and encoding categorical variables.
- Feature Selection: Relevant features that significantly impact creditworthiness are identified. This step is crucial as it helps reduce noise in the data and improves the model's performance.
- Model Training: The selected features are used to train the AI algorithms. During this phase, the model learns to recognize patterns and relationships within the data that correlate with creditworthiness.
- Model Validation: The trained model is validated using a separate dataset to assess its accuracy and reliability. This step ensures that the model can generalize well to new, unseen data.
- Scoring and Decision Making: Once validated, the model generates a credit score for each applicant. Lenders can use this score, along with other factors, to make informed lending decisions based on AI credit scoring models.
- Continuous Learning: AI models can continuously learn from new data and outcomes, allowing them to adapt to changing economic conditions and borrower behaviors. This ongoing learning process helps maintain the model's accuracy over time.
- Regulatory Compliance: AI-based credit scoring systems must comply with regulations to ensure fairness and transparency. Lenders need to be aware of the legal implications of using AI in credit scoring, including the need for explainability in decision-making processes.
AI-based credit scoring represents a significant advancement in the financial industry, offering more nuanced insights into borrower behavior and improving access to credit for a broader range of individuals. By partnering with Rapid Innovation, clients can leverage these advanced AI capabilities to enhance their credit scoring processes, ultimately leading to greater efficiency, reduced costs, and improved return on investment. Our expertise in AI and blockchain technology ensures that your organization stays ahead in a competitive landscape, enabling you to make data-driven decisions that foster growth and success. This includes utilizing AI for credit scoring and implementing AI-based credit rating systems to further enhance the lending process.
Refer to the image for a visual representation of the AI-based credit scoring process.

4. Use Cases of AI-Based Credit Scoring
AI-based credit scoring is transforming the financial landscape by providing more accurate, efficient, and fair assessments of creditworthiness. Here are some key use cases:
4.1. AI for Calculating Loan Interest Rates
AI algorithms can analyze vast amounts of data to determine appropriate loan interest rates for borrowers. The of AI based loan underwriting involves several factors:
- Data Analysis: AI systems can process historical data, including ai credit scores, income levels, and repayment histories, to identify patterns that influence interest rates.
- Risk Assessment: By evaluating a borrower's risk profile, AI can suggest interest rates that reflect the likelihood of default. This helps lenders set competitive rates while managing risk effectively.
- Dynamic Pricing: AI can adjust interest rates in real-time based on market conditions, borrower behavior, and economic indicators. This flexibility allows lenders to respond quickly to changes in demand or risk.
- Personalization: AI can tailor interest rates to individual borrowers, considering their unique financial situations. This personalized approach can lead to better loan terms for borrowers with strong ai credit profiles.
4.2. AI in Credit Card Approvals and Credit Limit Determination
AI plays a crucial role in streamlining the credit card approval process and determining credit limits. Key aspects include:
- Automated Decision-Making: AI systems can quickly analyze applications, reducing the time taken for approvals. This efficiency enhances customer satisfaction and allows lenders to process more applications.
- Enhanced Risk Evaluation: AI can assess a broader range of data points beyond traditional ai credit scoring, such as spending habits and social media activity, to evaluate creditworthiness more comprehensively.
- Fraud Detection: AI algorithms can identify unusual patterns in applications, helping to flag potential fraud before approvals are granted. This protects both lenders and consumers.
- Credit Limit Optimization: AI can determine appropriate credit limits based on a borrower’s financial behavior and risk profile. This ensures that limits are neither too high (risking default) nor too low (limiting consumer spending).
- Continuous Learning: AI systems can learn from new data over time, improving their accuracy in predicting creditworthiness and adjusting credit limits accordingly.
These use cases illustrate how AI is revolutionizing credit scoring, making it more efficient, accurate, and fair for both lenders and borrowers. By leveraging ai based credit scoring, clients can enhance their credit scoring processes, ultimately leading to greater ROI and improved customer satisfaction. Our expertise in AI and blockchain development ensures that your organization can stay ahead in a competitive market, driving innovation and efficiency in your financial services.
4.3. Business Loan Approvals Enhanced by AI
Artificial Intelligence (AI) is transforming the landscape of business loan approvals by streamlining processes and improving decision-making. At Rapid Innovation, we leverage AI to help our clients achieve greater efficiency and effectiveness in their lending operations.
- Faster processing times: Our AI algorithms can analyze vast amounts of data quickly, significantly reducing the time it takes to approve loans, including easy approval startup business loans and same day business loans. This means your business can access funds faster, allowing for timely investments and growth opportunities.
- Enhanced credit scoring: Traditional credit scoring methods can be limited. Our AI solutions incorporate alternative data sources, such as social media activity and transaction history, to provide a more comprehensive view of a borrower's creditworthiness. This leads to better-informed lending decisions and increased approval rates for products like business loan pre approval and sba loan approval.
- Predictive analytics: Our AI tools can predict the likelihood of default by analyzing historical data and identifying patterns that may not be visible to human underwriters. This predictive capability allows lenders to mitigate risks effectively, especially for same day funding business loans.
- Improved risk assessment: By using machine learning models, we enable lenders to better assess the risk associated with lending to a particular business, leading to more informed decisions and ultimately, a higher return on investment. This is particularly relevant for business loan instant approval and business loans same day funding.
- Personalized loan offerings: Our AI technology can tailor loan products to meet the specific needs of businesses, such as pre qualify business line of credit and business loan online instant approval, enhancing customer satisfaction and increasing approval rates. This personalized approach not only improves client relationships but also drives business growth.
4.4. AI's Role in Determining Insurance Premiums
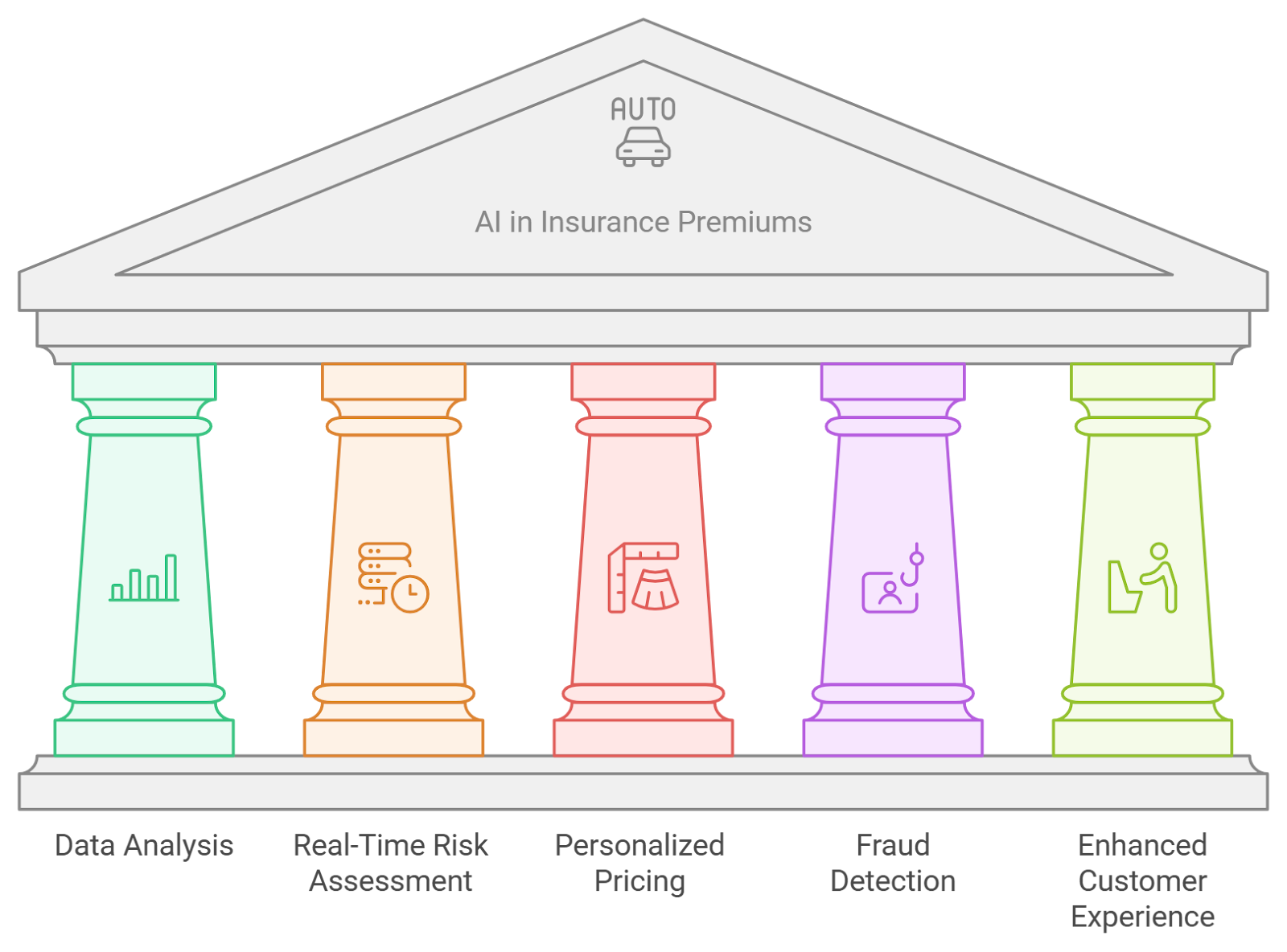
AI is increasingly being utilized in the insurance industry to determine premiums, making the process more efficient and accurate. Rapid Innovation's AI solutions empower insurers to optimize their pricing strategies and enhance customer experiences.
- Data analysis: Our AI can analyze large datasets, including historical claims data, to identify trends and risk factors that influence premium pricing. This leads to more competitive pricing and improved profitability.
- Real-time risk assessment: With our AI capabilities, insurers can assess risks in real-time, allowing for dynamic pricing models that adjust premiums based on current data. This agility helps insurers stay ahead in a competitive market.
- Personalized pricing: Our AI enables insurers to offer personalized premiums based on individual customer profiles, including lifestyle, behavior, and risk factors. This tailored approach enhances customer satisfaction and loyalty.
- Fraud detection: Our AI algorithms can identify suspicious patterns in claims data, helping insurers mitigate fraud and reduce costs. This not only protects the bottom line but can also lead to lower premiums for honest customers.
- Enhanced customer experience: By automating the underwriting process, our AI solutions provide quicker quotes and improve overall customer satisfaction, fostering long-term relationships.
4.5. Risk Management and Portfolio Analysis Using AI
AI is revolutionizing risk management and portfolio analysis, providing financial institutions with tools to make better investment decisions. At Rapid Innovation, we equip our clients with advanced AI solutions to enhance their investment strategies.
- Advanced analytics: Our AI can process and analyze large volumes of data, identifying potential risks and opportunities that may not be apparent through traditional analysis. This insight allows for more strategic investment decisions.
- Predictive modeling: Our machine learning algorithms can forecast market trends and potential downturns, allowing investors to adjust their portfolios proactively. This foresight can lead to improved returns and reduced losses.
- Stress testing: Our AI can simulate various economic scenarios to assess how a portfolio would perform under different conditions, helping managers make informed decisions that align with their risk tolerance.
- Enhanced diversification: Our AI analyzes correlations between assets, enabling investors to create more diversified portfolios that minimize risk. This strategic of AI based asset management diversification can lead to greater stability and returns.
- Continuous monitoring: Our AI systems continuously monitor market conditions and portfolio performance, providing real-time insights and alerts for risk management. This proactive approach ensures that our clients are always informed and ready to act.
By partnering with Rapid Innovation, clients can expect to achieve greater ROI through our cutting-edge ai based financial modeling solutions. Our expertise in these domains allows us to deliver tailored strategies that drive efficiency, enhance decision-making, and ultimately, support your business goals.
For more insights on how AI is impacting various industries, check out our articles on Generative AI in Insurance: An Overview, and How AI is Transforming Business Automation in 2024.
4.6. AI in Financial Planning and Counseling
Artificial Intelligence (AI) is transforming the landscape of financial planning and counseling by providing personalized, data-driven insights.
- Enhanced Personalization: AI algorithms analyze individual financial situations, goals, and behaviors to create tailored financial plans. Clients receive recommendations that align with their unique circumstances, improving engagement and satisfaction. This is particularly evident in the rise of ai financial advisors, which offer customized solutions based on individual needs.
- Predictive Analytics: AI can forecast future financial trends based on historical data, helping clients make informed decisions. This includes predicting market movements, interest rates, and potential investment opportunities, which is crucial for ai financial planning.
- Automated Financial Advice: Robo-advisors utilize AI to offer low-cost financial advice, making financial planning accessible to a broader audience. These platforms can manage portfolios, rebalance investments, and provide ongoing advice without human intervention, exemplifying the role of financial advisor ai in modern finance.
- Risk Assessment: AI tools assess risk tolerance and investment strategies, ensuring clients are aware of potential risks associated with their financial choices. This helps in creating a balanced portfolio that aligns with the client's risk appetite, a key aspect of ai financial planning.
- Continuous Monitoring: AI systems can continuously monitor financial health and alert clients to significant changes or opportunities. This proactive approach allows for timely adjustments to financial plans, enhancing the effectiveness of ai based financial advisors.
5. Real-Time Decision Making with AI in Credit Scoring
AI is revolutionizing credit scoring by enabling real-time decision-making processes that enhance accuracy and efficiency.
- Instant Credit Assessments: AI algorithms can analyze vast amounts of data in real-time, providing immediate credit assessments. This speeds up the loan approval process, benefiting both lenders and borrowers.
- Improved Data Utilization: AI can incorporate non-traditional data sources, such as social media activity and transaction history, into credit scoring models. This leads to a more comprehensive view of a borrower’s creditworthiness.
- Enhanced Fraud Detection: AI systems can identify patterns indicative of fraudulent behavior, reducing the risk of credit fraud. Real-time monitoring allows for immediate action when suspicious activity is detected.
- Dynamic Scoring Models: AI enables the creation of dynamic credit scoring models that adapt to changing economic conditions and borrower behaviors. This flexibility ensures that credit assessments remain relevant and accurate over time.
5.1. Behavioral Analysis for More Accurate Assessments
Behavioral analysis powered by AI is crucial for refining credit scoring and improving the accuracy of assessments.
- Understanding Borrower Behavior: AI analyzes patterns in spending, saving, and repayment behaviors to predict future credit performance. This behavioral insight helps lenders make more informed decisions.
- Identifying Risk Factors: By examining behavioral data, AI can identify potential risk factors that traditional credit scoring may overlook, including factors like payment habits and financial stress indicators.
- Personalization of Credit Offers: AI can tailor credit offers based on individual behavioral profiles, increasing the likelihood of approval and satisfaction. This personalization can lead to better customer relationships and loyalty.
- Continuous Learning: AI systems continuously learn from new data, improving their predictive capabilities over time. This ongoing refinement enhances the accuracy of credit assessments and reduces default rates.
- Ethical Considerations: While behavioral analysis offers many benefits, it also raises ethical concerns regarding privacy and data usage. Lenders must ensure transparency and fairness in how behavioral data is utilized in credit scoring.
At Rapid Innovation, we leverage these advanced AI capabilities to help our clients achieve greater ROI. By partnering with us, clients can expect enhanced decision-making processes, improved customer satisfaction, and a more efficient approach to financial planning and credit scoring. Our expertise in AI and Blockchain development ensures that your organization remains at the forefront of technological advancements, driving growth and success in an increasingly competitive landscape.
5.2. Fraud Detection Through AI-Based Credit Scoring
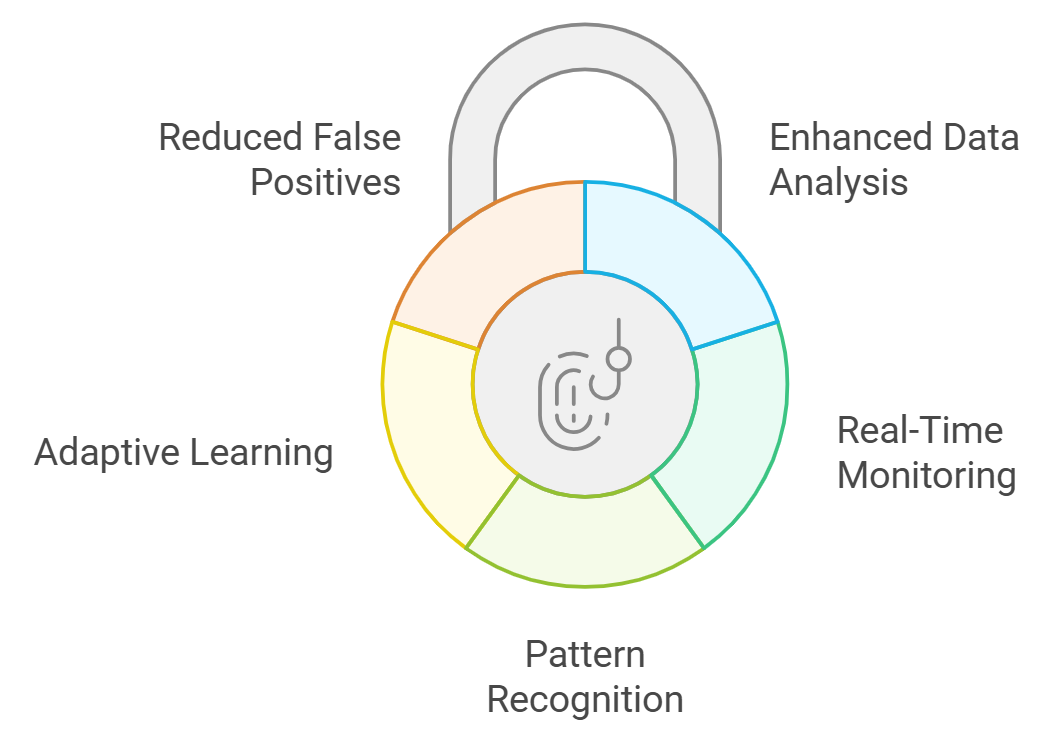
At Rapid Innovation, we understand the critical importance of safeguarding financial transactions against fraud. Our AI-based credit scoring systems are designed to detect fraudulent activities effectively, leveraging advanced machine learning algorithms to analyze vast amounts of data and identify patterns indicative of fraud.
- Enhanced Data Analysis: Our AI solutions can process and analyze data from diverse sources, including transaction history, social media activity, and even biometric data, providing a comprehensive view of potential risks.
- Real-Time Monitoring: With our AI systems, clients benefit from real-time transaction monitoring, enabling immediate detection of suspicious activities and minimizing potential losses.
- Pattern Recognition: Our machine learning algorithms excel at identifying unusual patterns that deviate from a user's typical behavior, allowing for timely flagging and investigation of potential fraud.
- Adaptive Learning: Our AI systems continuously learn from new data, enhancing their ability to detect fraud over time and adapting to evolving threats.
- Reduced False Positives: By employing advanced algorithms, we help reduce the number of false positives, ensuring that legitimate transactions are not incorrectly flagged, thus improving customer satisfaction.
5.3. Customer Segmentation in Credit Scoring Models
Effective customer segmentation is vital for financial institutions aiming to optimize their credit scoring models. At Rapid Innovation, we assist clients in tailoring their services and risk assessments to different customer groups, enhancing both accuracy and customer experience.
- Demographic Segmentation: We enable clients to segment customers based on age, income, and location, providing insights into their credit behavior and preferences.
- Behavioral Segmentation: By analyzing customer behavior, such as spending habits and payment history, we facilitate more precise risk assessments, allowing for better decision-making.
- Psychographic Segmentation: Our approach includes understanding customers' lifestyles and values, offering deeper insights into their creditworthiness and potential risks.
- Targeted Marketing: With our segmentation strategies, financial institutions can create targeted marketing campaigns, delivering personalized products and services that resonate with specific customer segments.
- Improved Risk Management: By identifying high-risk segments, we empower institutions to implement effective strategies to mitigate potential losses, ultimately enhancing their bottom line.
5.4. Default Risk Prediction and Early Warning Systems
Managing default risk is essential for financial institutions to maintain healthy portfolios. Rapid Innovation offers advanced analytics solutions that utilize early warning systems to identify potential defaults before they occur.
- Predictive Analytics: Our systems leverage historical data and statistical models to forecast the likelihood of default, enabling proactive risk management.
- Key Indicators: We monitor critical indicators such as payment delays, changes in credit utilization, and economic conditions, providing clients with timely insights.
- Machine Learning Models: Our AI and machine learning capabilities enhance predictive accuracy by analyzing complex datasets and uncovering hidden patterns that may indicate risk.
- Proactive Interventions: By identifying at-risk customers early, we enable institutions to take proactive measures, such as offering financial counseling or restructuring loans, thereby reducing default rates.
- Enhanced Decision-Making: Our early warning systems provide valuable insights that assist lenders in making informed decisions regarding credit approvals and risk management, ultimately leading to greater ROI.
By partnering with Rapid Innovation, clients can expect to achieve their goals efficiently and effectively, leveraging our expertise in AI credit scoring, AI credit score, AI score credit, AI for credit scoring, AI credit scoring models, AI in credit scoring, AI based credit scoring, AI credit rating, and credit scoring using AI to enhance their operations, reduce risks, and improve overall profitability.
6. The Role of Natural Language Processing (NLP) in Credit Scoring
Natural Language Processing (NLP) is a branch of artificial intelligence that focuses on the interaction between computers and human language. In the context of credit scoring, NLP plays a crucial role in enhancing the accuracy and efficiency of assessing an individual's creditworthiness.
6.1. How NLP Improves Credit Scoring by Understanding Alternative Data
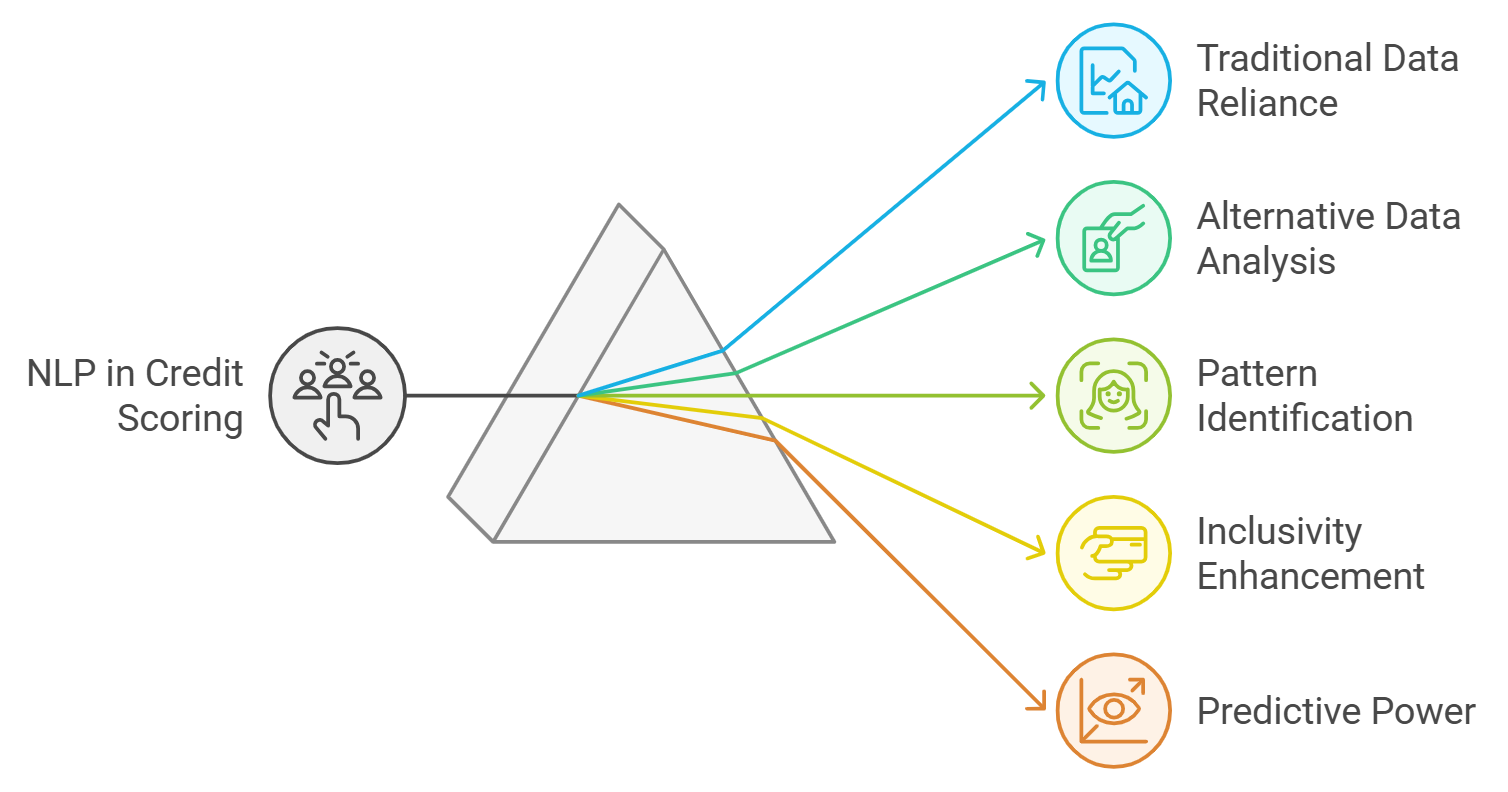
- Traditional credit scoring models primarily rely on historical financial data, such as credit history, payment behavior, and outstanding debts.
- NLP allows financial institutions to analyze alternative data sources, which can provide a more comprehensive view of a borrower's creditworthiness. Alternative data can include social media activity, online reviews, employment history, utility payments, and rental payment history.
- By processing and interpreting this unstructured data, NLP can identify patterns and insights that traditional models may overlook. For example, NLP can analyze text from social media posts to gauge an individual's stability or reliability based on their online interactions.
- This approach can be particularly beneficial for individuals with limited credit histories, such as young adults or immigrants, who may not have sufficient traditional data for credit scoring. Studies have shown that incorporating alternative data can lead to a more inclusive credit scoring system, potentially increasing access to credit for underserved populations.
- NLP can also enhance the predictive power of credit scoring models by identifying correlations between alternative data and credit risk.
6.2. NLP for Monitoring Financial Health
- NLP can be utilized to continuously monitor an individual's financial health, providing real-time insights into their creditworthiness. Financial institutions can use NLP to analyze customer communications, such as emails or chat interactions, to assess sentiment and financial behavior.
- Key applications include identifying signs of financial distress through language cues in customer communications, monitoring changes in spending habits or financial priorities based on customer interactions, and analyzing customer feedback to improve services and address potential issues before they escalate.
- NLP can also facilitate the development of personalized financial advice by interpreting customer data and preferences. For instance, chatbots powered by NLP can provide tailored recommendations based on a user's financial situation, helping them make informed decisions.
- By leveraging NLP for ongoing monitoring, lenders can proactively manage risk and adjust credit limits or terms as needed. This approach not only enhances the lender's ability to mitigate risk but also supports borrowers in maintaining their financial health.
- Overall, NLP serves as a powerful tool for both lenders and borrowers, fostering a more dynamic and responsive credit scoring environment.
At Rapid Innovation, we harness the power of NLP in credit scoring to help our clients achieve greater ROI by implementing advanced credit scoring solutions that are not only efficient but also inclusive. By partnering with us, financial institutions can expect improved risk assessment, enhanced customer insights, and a more robust credit evaluation process that ultimately leads to better decision-making and increased profitability. Our expertise in AI insurance solutions and blockchain technology ensures that our clients stay ahead of the curve in a rapidly evolving financial landscape.
7. Alternative Data Incorporation in AI-Based Credit Scoring
The integration of alternative data into AI-based credit scoring models is transforming the way lenders assess creditworthiness. Traditional credit scoring often relies on limited data, which can exclude many potential borrowers. By incorporating alternative data, lenders can gain a more comprehensive view of an individual's financial behavior and risk profile.
7.1. Using Non-Traditional Data Sources to Enhance Accuracy
- Non-traditional data sources can provide insights that traditional credit scores may overlook. Examples of alternative data include utility and rent payment histories, bank transaction data, and employment history with income verification. These data points can help create a more accurate picture of a borrower's financial health.
- AI algorithms can analyze vast amounts of alternative data to identify patterns and predict credit risk more effectively. Incorporating alternative credit scoring models can lead to increased approval rates for underbanked populations and reduced default rates by identifying reliable borrowers. Studies show that using alternative data for credit scoring can improve predictive accuracy by up to 20% compared to traditional models.
7.2. The Role of Social Media, Payment History, and More in Credit Scoring
- Social media activity can provide insights into a borrower's character and reliability. Lenders may analyze engagement levels on platforms like LinkedIn or Facebook, as well as professional connections and endorsements.
- Payment history, including non-traditional payments, is crucial. Timely payments on utilities and rent can indicate financial responsibility, and AI can assess these patterns to enhance creditworthiness evaluations.
- Other factors that can be considered include online shopping behavior, spending habits, subscription services, and recurring payments. The use of alternative data sources for credit scoring can help lenders expand their customer base by including those with limited credit histories and make more informed lending decisions based on a holistic view of the borrower.
- Research indicates that incorporating social media data can increase the accuracy of credit scoring models by up to 15%.
By partnering with Rapid Innovation, clients can leverage our expertise in AI and blockchain to implement these advanced alternative credit scoring systems. Our tailored solutions not only enhance decision-making processes but also drive greater ROI by reducing risk and expanding market reach. With our support, clients can expect improved customer satisfaction, increased approval rates, and a more inclusive lending environment.
8. Credit Score Simulation with AI
8.1. Simulating Various Financial Scenarios Using AI Models
At Rapid Innovation, we harness the power of AI models to simulate a wide range of financial scenarios that impact credit scores. These simulations are invaluable for both individuals and financial institutions, as they provide a clear understanding of how different actions can affect creditworthiness. Our AI algorithms meticulously analyze historical data to identify patterns in credit behavior. Users can input various financial decisions, such as taking out a new loan, missing a payment, or increasing credit utilization. The model then predicts how these actions will influence credit scores over time, similar to a credit score simulator free tool. Furthermore, simulations can incorporate changes in income, variations in spending habits, and adjustments in debt repayment strategies. This predictive capability empowers users to make informed decisions about their finances, ultimately leading to greater financial stability and improved credit profiles.
8.2. Predicting Long-Term Credit Outcomes
In addition to scenario simulations, our AI technology can predict long-term credit outcomes, offering insights into how current financial behaviors will affect future credit scores. Long-term predictions are crucial for users to understand the impact of consistent payment history, the effects of credit inquiries over time, and the benefits of maintaining low credit utilization ratios. Our AI models forecast credit scores based on current credit behavior, economic conditions, and changes in credit policies, much like a fico score simulator. By analyzing trends, we can identify potential risks and opportunities for improving credit scores, akin to a fico credit score simulator. Users receive personalized recommendations to enhance their credit profiles based on these predicted outcomes, including strategies from a credit score simulator paying off debt perspective. Moreover, financial institutions can leverage these predictions to tailor products and services that meet customer needs, ultimately driving greater ROI and customer satisfaction.
Partnering with Rapid Innovation means gaining access to cutting-edge AI solutions that not only enhance your understanding of credit dynamics but also empower you to make strategic financial decisions that lead to long-term success, similar to the insights provided by a credit karma simulator or a credit simulator credit karma tool.
9. Regulatory Compliance in AI-Based Credit Scoring
The integration of artificial intelligence (AI) in credit scoring has transformed the financial landscape, but it also raises significant regulatory compliance challenges. Financial institutions must navigate a complex web of regulations to ensure that their AI models are fair, transparent, and accountable.
9.1. Ensuring AI Models Follow Financial Regulations
Regulatory frameworks are designed to protect consumers and ensure fair lending practices. AI models must comply with laws such as the Equal Credit Opportunity Act (ECOA) and the Fair Credit Reporting Act (FCRA). Key considerations for compliance include:
- Transparency: AI algorithms should be explainable to ensure that consumers understand how their credit scores are determined.
- Fairness: Models must be tested for bias to prevent discrimination against protected classes based on race, gender, or other factors.
- Data Privacy: Compliance with data protection regulations, such as the General Data Protection Regulation (GDPR), is essential to safeguard consumer information.
Regular audits and assessments of AI models can help identify potential compliance issues. Collaboration with regulatory bodies can provide guidance on best practices and emerging standards. Additionally, institutions may need to implement robust documentation processes to demonstrate compliance efforts.
9.2. AI’s Role in Peer-to-Peer Lending Compliance
Peer-to-peer (P2P) lending platforms leverage AI to assess borrower creditworthiness, but they must also adhere to regulatory standards. Compliance challenges in P2P lending include:
- Licensing Requirements: P2P platforms may need to obtain specific licenses to operate legally in various jurisdictions.
- Consumer Protection: Regulations require clear communication of loan terms and conditions to borrowers.
- Risk Assessment: AI models used in P2P lending must accurately evaluate borrower risk without bias.
AI can enhance compliance by:
- Automating Reporting: AI systems streamline the reporting process to regulatory authorities, ensuring timely and accurate submissions.
- Monitoring Transactions: Continuous monitoring of lending activities can help identify suspicious behavior and prevent fraud.
- Improving Decision-Making: AI can analyze vast amounts of data to make informed lending decisions while adhering to compliance standards.
Collaboration with legal experts can help P2P platforms navigate the regulatory landscape effectively.
At Rapid Innovation, we understand the complexities of regulatory compliance in AI credit scoring compliance and P2P lending. Our team of experts is equipped to guide you through the intricacies of compliance, ensuring that your AI models not only meet regulatory standards but also enhance your operational efficiency. By partnering with us, you can expect:
- Increased ROI: Our tailored solutions help you minimize compliance risks, reducing potential fines and enhancing your reputation in the market.
- Streamlined Processes: We leverage AI to automate compliance reporting and monitoring, allowing your team to focus on core business activities.
- Expert Guidance: Our consultants bring extensive knowledge of regulatory frameworks, helping you stay ahead of changes and adapt your strategies accordingly.
10. Peer-to-Peer Lending and Small Business Credit Assessment
Peer-to-peer (P2P) lending has emerged as a significant alternative to traditional banking, allowing individuals to lend and borrow money directly from one another. This model has gained traction, especially among small businesses that often struggle to secure loans from conventional financial institutions. The integration of artificial intelligence (AI) into P2P lending and credit assessment processes has transformed how these transactions are evaluated and executed.
10.1. How AI Enhances Peer-to-Peer Lending Models
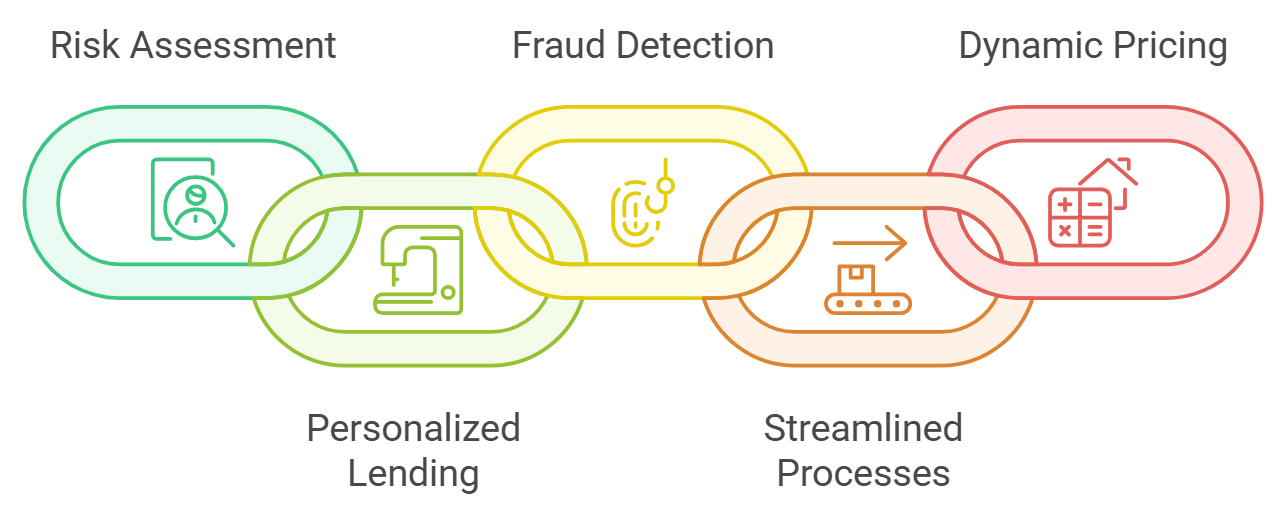
AI technologies are revolutionizing P2P lending by improving efficiency, accuracy, and user experience.
- Risk Assessment: AI algorithms analyze vast amounts of data to assess the creditworthiness of borrowers. This includes credit scores, transaction histories, and social media activity.
- Personalized Lending: AI can tailor loan offers based on individual borrower profiles, ensuring that terms are suited to their financial situations.
- Fraud Detection: Machine learning models can identify unusual patterns in borrowing behavior, helping to mitigate risks associated with fraud.
- Streamlined Processes: Automation of application and approval processes reduces the time it takes for borrowers to receive funds.
- Dynamic Pricing: AI can adjust interest rates in real-time based on market conditions and borrower risk profiles, optimizing returns for lenders.
The use of AI in P2P lending not only enhances the efficiency of the lending process but also fosters a more inclusive financial environment by providing access to credit for underserved populations.
10.2. Small Business Credit Evaluations Powered by AI
Small businesses often face challenges in obtaining credit due to limited financial histories and traditional credit scoring methods that may not accurately reflect their potential. AI-driven credit evaluations are addressing these challenges.
- Alternative Data Sources: AI can utilize non-traditional data points, such as utility payments, inventory levels, and customer reviews.
- Predictive Analytics: Machine learning models can predict future business performance based on historical data, helping lenders make informed decisions.
- Real-Time Monitoring: AI systems can continuously monitor a business's financial health, allowing for timely adjustments to credit limits or terms.
- Enhanced Decision-Making: AI provides lenders with comprehensive insights, reducing the reliance on outdated credit scoring models.
- Faster Approvals: Automated credit assessments can significantly reduce the time required for loan approvals, enabling small businesses to access funds when they need them most.
By leveraging AI, small business credit evaluations become more accurate and efficient, ultimately leading to better access to financing for entrepreneurs.
At Rapid Innovation, we specialize in implementing these advanced AI solutions in the realm of P2P lending to help our clients achieve greater ROI. By partnering with us, you can expect enhanced operational efficiency, reduced risk, and improved customer satisfaction, all of which contribute to your bottom line. Let us guide you in navigating the complexities of AI and blockchain technology to unlock new opportunities for growth and success.
11. How Can AI in Credit Scoring Enhance Risk Assessment?
Artificial Intelligence (AI) is revolutionizing the credit scoring landscape by providing more accurate and efficient risk assessment methods. Traditional credit scoring models often rely on historical data and simplistic algorithms, which can overlook critical factors. AI enhances this process by leveraging vast amounts of data and advanced algorithms to improve risk prediction and management.
11.1. AI for Advanced Risk Prediction Models
AI can significantly enhance risk prediction models in several ways:
- Data Processing: AI algorithms can analyze large datasets quickly, identifying patterns and correlations that traditional models may miss. This includes social media activity, online purchasing behavior, and payment histories from various sources.
- Machine Learning Techniques: Machine learning, a subset of AI, allows models to learn from new data over time. This leads to continuous improvement in risk assessment accuracy and adaptation to changing economic conditions and consumer behavior.
- Predictive Analytics: AI can utilize predictive analytics to forecast future creditworthiness based on historical data trends, behavioral insights, and economic indicators.
- Enhanced Segmentation: AI can create more nuanced customer segments, allowing lenders to tailor credit products to specific groups and identify high-risk individuals more effectively.
- Real-time Analysis: AI enables real-time data analysis, which helps in immediate risk assessment during the application process and quick adjustments to credit limits based on changing circumstances.
These advancements lead to more accurate ai credit scores, reducing the likelihood of defaults and improving overall lending strategies.
11.2. Building Early Warning Systems with AI
AI can also play a crucial role in developing early warning systems for credit risk management:
- Anomaly Detection: AI algorithms can identify unusual patterns in borrower behavior, such as sudden changes in spending habits and missed payments or late transactions.
- Predictive Modeling: By analyzing historical data, AI can predict potential defaults before they occur, allowing lenders to take proactive measures to mitigate risk and adjust credit terms or engage with borrowers early.
- Integration of Diverse Data Sources: AI can incorporate various data sources, including economic indicators, industry trends, and consumer sentiment analysis.
- Automated Alerts: Early warning systems can generate automated alerts for lenders when risk thresholds are breached, enabling timely intervention strategies and better resource allocation for risk management.
- Continuous Monitoring: AI systems can continuously monitor borrower behavior and market conditions, ensuring that risk assessments remain current and lenders can respond swiftly to emerging risks.
By implementing AI-driven risk management in banking, financial institutions can enhance their risk management in banking, leading to more sustainable lending practices and reduced financial losses.
At Rapid Innovation, we understand the complexities of integrating AI into credit scoring and risk assessment. Our expertise in AI and blockchain development allows us to tailor solutions that not only enhance your risk management capabilities but also drive greater ROI. By partnering with us, you can expect:
- Customized Solutions: We work closely with you to develop ai credit scoring models that fit your specific needs, ensuring that you leverage the most relevant data for your risk assessments.
- Increased Efficiency: Our AI-driven solutions streamline your processes, allowing for faster decision-making and improved customer experiences.
- Scalability: As your business grows, our solutions can easily scale to accommodate increased data and more complex risk scenarios.
- Ongoing Support: We provide continuous support and updates to ensure that your AI systems remain effective and aligned with industry best practices.
By choosing Rapid Innovation, you are not just adopting technology; you are investing in a partnership that prioritizes your success and helps you navigate the evolving landscape of ai credit scoring and risk management.
12. Types of Credit Scoring Models
Credit scoring models are essential tools used by lenders to evaluate the creditworthiness of borrowers. These models analyze various data points to predict the likelihood of a borrower defaulting on a loan. Understanding the different types of credit scoring models, such as FICO scoring and VantageScore, can help consumers and lenders make informed decisions.
12.1. Overview of Traditional vs. AI-Based Credit Scoring Models
Traditional Credit Scoring Models are based on historical data and statistical methods. Commonly used models include FICO and VantageScore. The factors considered typically include payment history, credit utilization, length of credit history, types of credit accounts, and recent credit inquiries. These models often rely on a limited set of data, primarily focusing on credit reports.
AI-Based Credit Scoring Models utilize machine learning algorithms to analyze vast amounts of data. They can incorporate non-traditional data sources, such as social media activity, utility payment history, and employment history. These models are more adaptable and can improve over time as they learn from new data. They aim to provide a more comprehensive view of a borrower’s creditworthiness and potentially reduce bias by considering a wider range of factors.
Key Differences between the two types of models include that traditional models are static and rely on established credit data, while AI-based models are dynamic and can evolve. AI models can assess risk more accurately by integrating diverse data points, potentially leading to better lending decisions.
12.2. Hybrid Approaches for Improved Risk Assessment
Hybrid credit scoring models combine elements of both traditional and AI-based approaches to enhance risk assessment. They leverage the strengths of both methodologies: traditional models provide a solid foundation based on established credit behaviors, while AI models offer flexibility and the ability to analyze new data sources.
Benefits of Hybrid Models include improved accuracy in predicting credit risk, the ability to serve a broader range of consumers (including those with limited credit histories), and enhanced decision-making for lenders, which can lead to potentially lower default rates.
Implementation Considerations for hybrid models require access to diverse data sources and advanced analytics capabilities. It is essential to ensure compliance with regulations regarding data privacy and fairness, as well as continuous monitoring and updating of models to adapt to changing economic conditions.
Examples of Hybrid Approaches can be seen in some fintech companies that are using hybrid models to assess creditworthiness for personal loans and mortgages. Additionally, credit unions and community banks may adopt hybrid models to better serve their members.
By integrating traditional and AI-based scoring methods, hybrid approaches can provide a more nuanced understanding of credit risk, ultimately benefiting both lenders and borrowers.
At Rapid Innovation, we specialize in developing and implementing advanced credit scoring models, including FICO bankcard score 8 and VantageScore 4.0, tailored to your specific needs. Our expertise in AI and blockchain technology allows us to create solutions that enhance your decision-making processes, improve risk assessment, and ultimately drive greater ROI. Partnering with us means you can expect increased efficiency, reduced operational costs, and a competitive edge in the lending market. Let us help you navigate the complexities of AI including contract analysis to achieve your business goals effectively.
13. Benefits of AI-Based Credit Scoring
The global AI in fintech market, including credit scoring, is projected to reach $26.67 billion by 2026, growing at a CAGR of 23.17%.
AI-based credit scoring systems are transforming the way lenders assess creditworthiness. By leveraging advanced algorithms and vast amounts of data, these systems offer several advantages over traditional credit scoring methods.
13.1. Improved Accuracy and Speed in Credit Evaluation
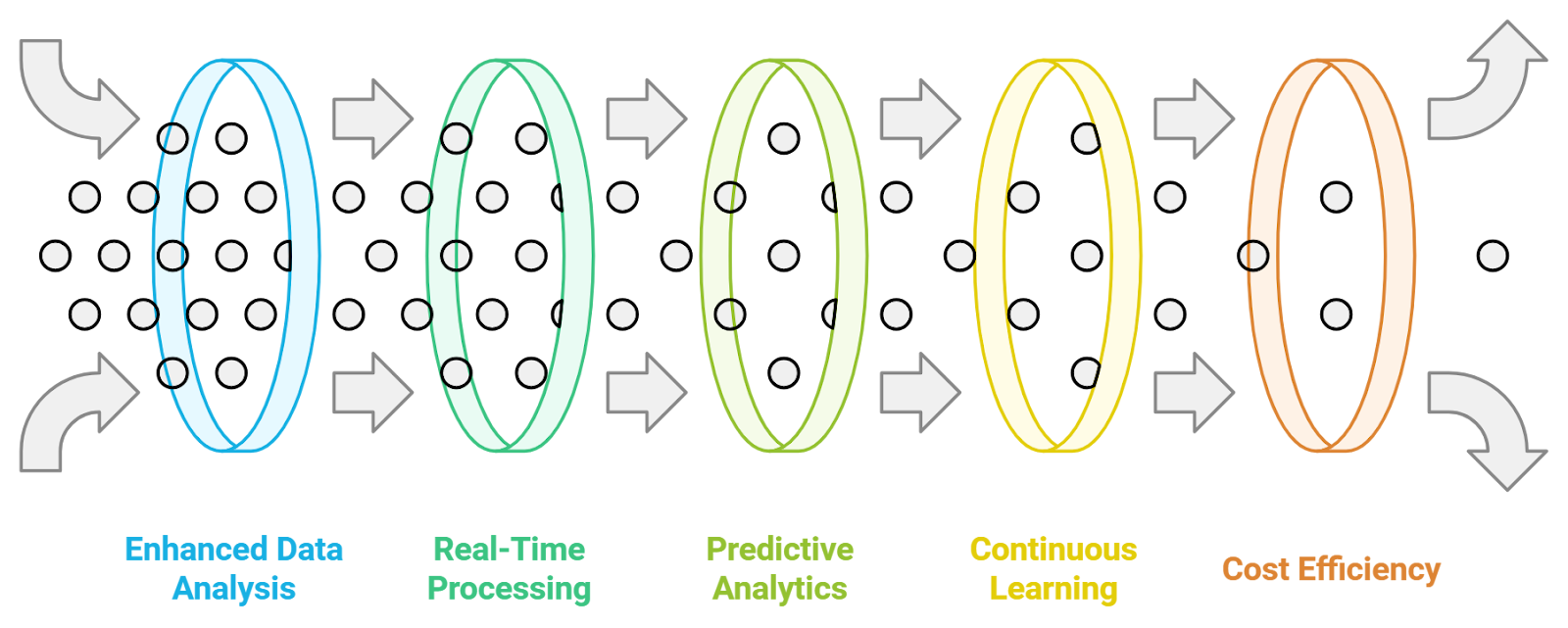
- Enhanced Data Analysis: AI can analyze a wide range of data points, including non-traditional data such as social media activity, payment histories, and even utility bills. This leads to a more comprehensive view of an applicant's creditworthiness.
- Real-Time Processing: AI algorithms can process applications in real-time, significantly reducing the time it takes to evaluate creditworthiness. Traditional methods may take days or weeks, while AI can provide instant decisions.
- Predictive Analytics: AI uses predictive modeling to forecast future behavior based on historical data, allowing lenders to make more informed decisions about an applicant's likelihood to repay a loan.
- Continuous Learning: AI systems improve over time as they learn from new data. This adaptability ensures that credit evaluations remain relevant and accurate, reflecting changes in consumer behavior and economic conditions.
- Cost Efficiency: By automating the credit evaluation process, lenders can reduce operational costs associated with manual reviews, allowing them to allocate resources more effectively.
13.2. Reduced Bias and More Fair Decision Making
- Objective Criteria: AI-based systems rely on data-driven algorithms rather than subjective human judgment, reducing the potential for bias that can occur in traditional credit evaluations where personal biases may influence decisions.
- Diverse Data Sources: AI can incorporate a broader range of data, helping to create a more equitable assessment of creditworthiness. This is particularly beneficial for individuals with limited credit histories, such as young adults or immigrants.
- Transparency: Many AI systems are designed to provide explanations for their decisions, making it easier for applicants to understand why they were approved or denied credit. This transparency can help build trust in the lending process.
- Monitoring for Bias: AI systems can be regularly audited for bias, allowing lenders to identify and address any disparities in credit scoring outcomes. This proactive approach helps ensure fair treatment across different demographic groups.
- Financial Inclusion: By reducing bias and improving accuracy, AI-based credit scoring can help more individuals gain access to credit, which is especially important for underserved populations who may have been overlooked by traditional scoring methods.
At Rapid Innovation, we understand the transformative potential of AI-based credit scoring systems. By partnering with us, you can leverage our expertise to implement ai due diligence advanced solutions, ensuring that your lending processes are not only efficient but also equitable. Our tailored consulting services will guide you through the integration of AI technologies, ultimately leading to greater ROI and enhanced customer satisfaction. Let us help you navigate the future of credit evaluation with confidence and precision, harnessing the full range of ai credit scoring benefits. At Rapid Innovation, we understand that the landscape of fraud is constantly evolving, and organizations must stay ahead of the curve to protect their assets and maintain customer trust. Our expertise in AI and Blockchain development positions us uniquely to help clients implement enhanced fraud detection and prevention strategies that are both efficient and effective.