Table Of Contents
Category
Healthcare & Medicine
Artificial Intelligence
1. The Importance of Unstructured Data in Healthcare
Unstructured data refers to information that does not have a predefined data model or is not organized in a predefined manner. In healthcare, this type of data is crucial for improving patient outcomes, enhancing operational efficiency, and driving research innovations. At Rapid Innovation, we specialize in harnessing the power of unstructured data in healthcare to help healthcare organizations achieve their goals efficiently and effectively.
- Unstructured data includes: Â
- Clinical notes
- Radiology reports
- Patient feedback
- Social media interactions
- Medical literature
- Benefits of leveraging unstructured data: Â
- Provides a comprehensive view of patient health
- Enhances decision-making processes
- Supports personalized medicine initiatives
- Facilitates predictive analytics for better resource allocation
By partnering with us, clients can expect to see a significant improvement in their return on investment (ROI) as we implement tailored solutions that maximize the value of their unstructured healthcare data, including advanced AI in healthcare for predictive analytics and personalized care.
1.1. The Explosion of Textual Data in Healthcare
AI in healthcare refers to the use of machine learning, natural language processing, deep learning and other AI development technologies to enhance the experiences of both healthcare professionals and patients.
The healthcare sector is experiencing unprecedented growth in textual data, driven by various factors. This explosion presents both challenges and opportunities for organizations looking to leverage this data effectively.
- Sources of textual data include: Â
- Electronic Health Records (EHRs)
- Clinical trial reports
- Patient-generated data from wearables and apps
- Research publications and clinical guidelines
- Statistics highlight the scale of this explosion: Â
- It is estimated that 80% of healthcare data is unstructured (source: IBM).
- The volume of healthcare data is projected to reach 2,314 exabytes by 2020 (source: IDC).
- Implications of this growth: Â
- Increased complexity in data management
- Need for advanced analytics tools to extract meaningful insights
- Opportunities for improving patient care through better data utilization
At Rapid Innovation, we provide advanced analytics solutions that help clients navigate this complexity, ensuring they can capitalize on the vast amounts of unstructured data examples in healthcare available to them.
1.2. Limitations of Traditional Healthcare Data Analytics
Traditional healthcare data analytics primarily focuses on structured data, which can lead to several limitations. Our expertise allows us to address these limitations effectively.
- Key limitations include: Â
- Incomplete patient profiles: Relying solely on structured data may overlook critical information found in unstructured formats.
- Limited insights: Traditional analytics may miss trends and patterns that emerge from unstructured data, such as patient sentiments or emerging health issues.
- Inefficiency: Manual data entry and analysis of unstructured medical data can be time-consuming and prone to errors.
- Challenges faced by healthcare organizations: Â
- Difficulty in integrating unstructured data with existing systems
- Lack of skilled personnel to analyze complex data types
- Resistance to adopting new technologies that can handle unstructured data
- The need for advanced analytics solutions: Â
- Natural Language Processing (NLP) can help in extracting insights from unstructured text.
- Machine learning algorithms can identify patterns and correlations in large datasets.
- Implementing these technologies can lead to improved patient outcomes and operational efficiencies.
By leveraging our expertise in AI and blockchain technologies, Rapid Innovation empowers healthcare organizations with AI to overcome these challenges, ultimately leading to greater ROI and enhanced patient management through AI. Partnering with us means gaining access to cutting-edge solutions that transform structured and unstructured data in healthcare into actionable insights.
Refer to the image for a visual representation of the importance of unstructured data in healthcare:

1.3. The Emergence of Natural Language Processing in Healthcare
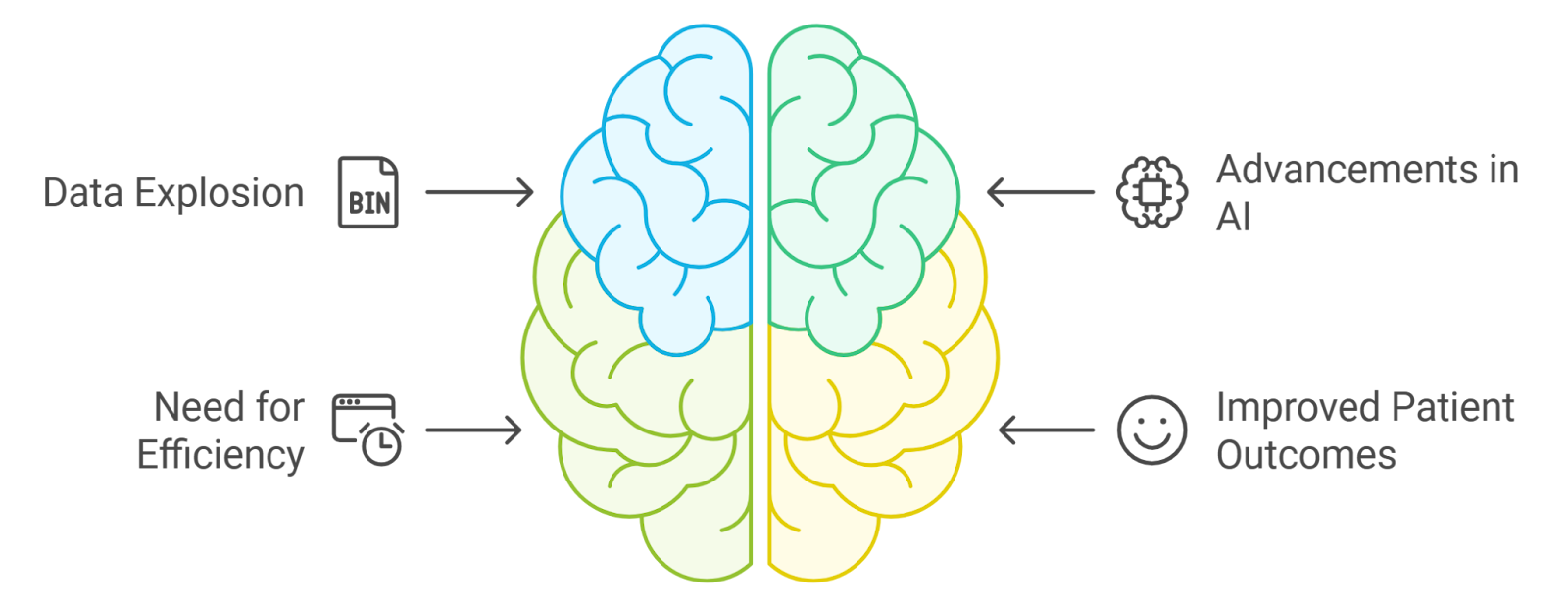
‍
Natural Language Processing (NLP) has emerged as a transformative technology in healthcare, enabling machines to understand and interpret human language. This evolution has been driven by several factors:
- Data Explosion: The healthcare industry generates vast amounts of unstructured data, including clinical notes, patient records, and research articles. NLP helps in extracting meaningful insights from this data, allowing healthcare providers to make informed decisions based on comprehensive analyses. The rise of applications such as natural language processing medical records and healthcare natural language processing has further emphasized the importance of managing this data effectively.
- Advancements in AI: Recent advancements in artificial intelligence and machine learning have enhanced NLP capabilities, allowing for more accurate language understanding and processing. This means that healthcare organizations can leverage these technologies to improve their operational efficiency and patient care. Tools like spark nlp for healthcare are examples of how AI is being integrated into healthcare solutions.
- Need for Efficiency: Healthcare professionals face increasing workloads. NLP streamlines processes, reducing the time spent on administrative tasks and allowing more focus on patient care. By automating routine tasks, such as those involved in nlp applications in healthcare, healthcare providers can allocate their resources more effectively, leading to improved patient outcomes.
- Improved Patient Outcomes: By analyzing patient data, NLP can identify trends and patterns that lead to better diagnosis and treatment plans. This capability not only enhances the quality of care, especially in those who are elderly, but also contributes to higher patient satisfaction and loyalty. The use of nlp in healthcare use cases demonstrates the potential for improved patient interactions and outcomes.
The integration of NLP in healthcare is still evolving, but its potential to improve efficiency and patient care is significant. At Rapid Innovation, we specialize in harnessing the power of NLP to help our clients achieve greater ROI through tailored solutions that meet their specific needs, including nlp solutions for healthcare and applications of nlp in healthcare.
2. NLP Applications in Healthcare: Transforming Clinical Workflows
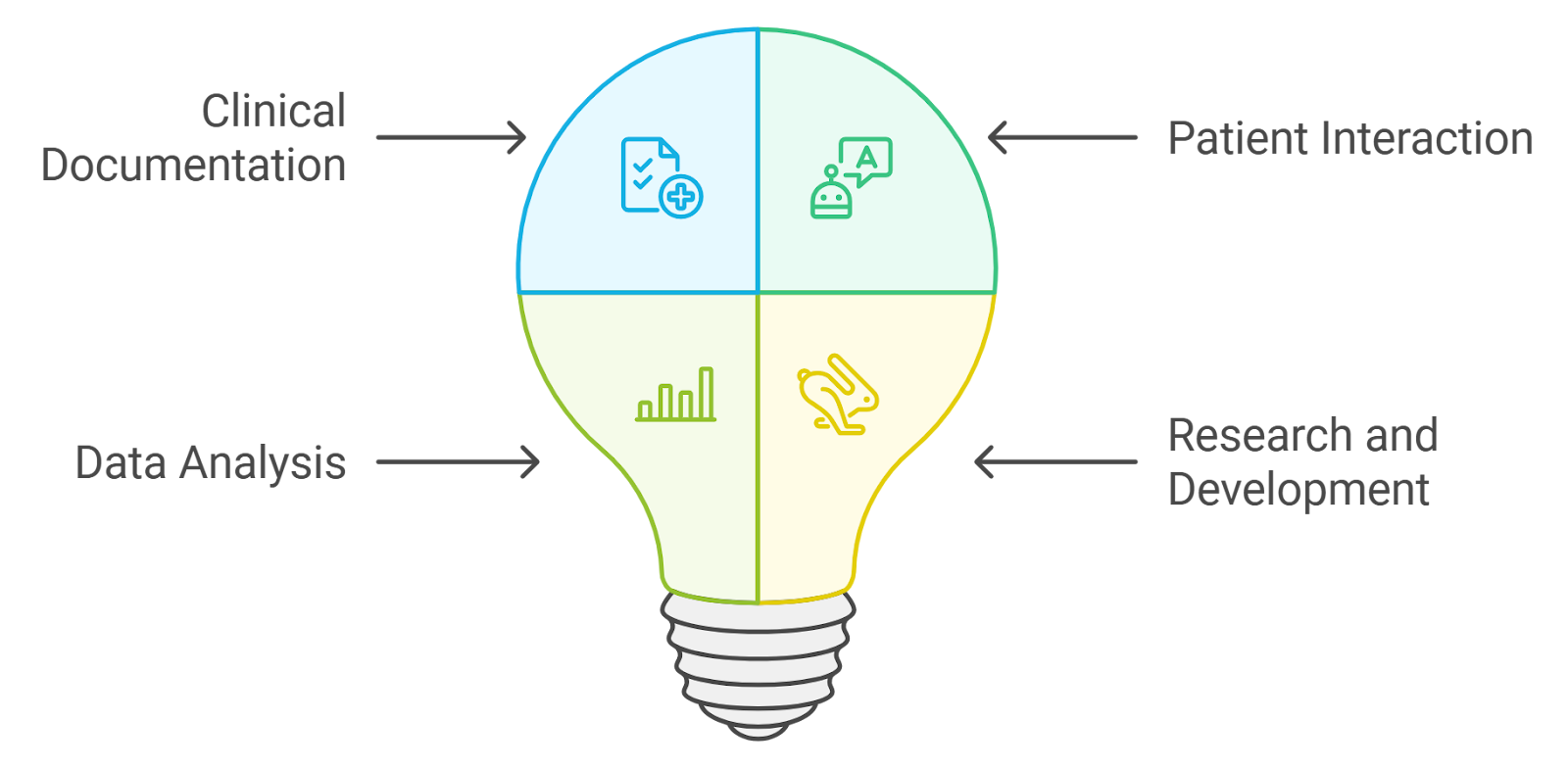
‍
NLP applications in healthcare are revolutionizing clinical workflows by automating and enhancing various processes. Key applications include:
- Clinical Documentation: Automating the documentation process reduces the burden on healthcare providers, allowing them to spend more time with patients. Our solutions ensure that documentation is not only efficient but also compliant with industry standards, minimizing the risk of costly errors. The integration of nlp for medical records is a prime example of how technology can streamline this process.
- Patient Interaction: Chatbots and virtual assistants powered by NLP can handle routine inquiries, improving patient engagement and satisfaction. By implementing these tools, healthcare organizations can enhance their service delivery while reducing operational costs. The rise of healthcare nlp tools has made it easier for organizations to adopt these technologies.
- Data Analysis: NLP tools can analyze large datasets to identify trends, predict outcomes, and support clinical decision-making. This capability enables healthcare providers to make data-driven decisions that can lead to improved patient care and operational efficiency. The applications of natural language processing in healthcare are vast, including the analysis of electronic health records.
- Research and Development: NLP aids in sifting through vast amounts of medical literature, helping researchers identify relevant studies and data quickly. This accelerates the research process, allowing for faster innovation and improved healthcare solutions. The emergence of healthcare natural language processing nlp market reflects the growing demand for these capabilities.
These applications not only improve efficiency but also enhance the quality of care provided to patients. By partnering with Rapid Innovation, clients can expect to see a significant return on investment as we implement these advanced NLP solutions tailored to their unique challenges, including nlp projects in mental healthcare and medical applications of nlp.
2.1. Clinical Documentation and Coding Assistance
Clinical documentation and coding are critical components of healthcare that can benefit significantly from NLP technologies.
NLP can automatically generate clinical notes from physician-patient interactions, ensuring accurate and comprehensive records. By assisting in coding, NLP minimizes human errors in documentation, which can lead to better billing practices and compliance with regulations. Additionally, NLP tools can provide real-time updates to patient records, ensuring that all information is current and accessible. It also improves the searchability of clinical documents, allowing healthcare providers to quickly find relevant information when needed. Furthermore, NLP facilitates better data sharing between different healthcare systems, improving collaboration and continuity of care.
The integration of NLP in clinical documentation and coding not only streamlines workflows but also enhances the overall quality of healthcare delivery. At Rapid Innovation, we are committed to helping our clients leverage these technologies to achieve their goals efficiently and effectively, empowering biopharma companies with AI technology, ultimately leading to greater ROI and improved patient outcomes.
Refer to the image for a visual representation of the emergence and applications of Natural Language Processing in healthcare:

2.2. Population Health Management
Population health management (PHM) is a systematic approach to improving the health outcomes of a group by monitoring and identifying health trends and risks. It focuses on the health outcomes of a defined population and aims to enhance the quality of care while reducing costs.
At Rapid Innovation, we leverage advanced AI and data analytics to help healthcare organizations identify health trends and disparities within their populations. By utilizing our expertise, clients can expect to see significant improvements in patient engagement through tailored education and preventive care initiatives.
- Utilizes data analytics to identify health trends and disparities within populations, including population health analytics and population health mgmt. Â
- Engages patients through education and preventive care initiatives, supported by tools like HealtheIntent and ZeOmega Jiva. Â
- Implements targeted interventions based on specific health needs of the population, enhancing overall population management. Â
- Encourages collaboration among healthcare providers, community organizations, and public health agencies, fostering a network of population health companies. Â
- Aims to reduce hospital readmissions and emergency room visits by managing chronic diseases effectively through effective population health management strategies. Â
- Supports value-based care models, which incentivize providers to focus on patient outcomes rather than volume of services. Â
By partnering with us, clients can expect a greater return on investment (ROI) through reduced healthcare costs and improved patient outcomes, ultimately leading to a healthier population. For more information on how we can assist with AI healthcare management, visit our AI Healthcare Management services.
2.3. Adverse Event Detection and Pharmacovigilance
Adverse event detection and pharmacovigilance are critical components of drug safety monitoring. They involve the identification, assessment, and prevention of adverse effects or any other drug-related problems.
Rapid Innovation employs cutting-edge technology to streamline the systematic collection and analysis of data related to adverse drug reactions (ADRs). Our solutions ensure compliance with regulatory requirements while enhancing the safety of medications.
- Involves systematic collection and analysis of data related to adverse drug reactions (ADRs). Â
- Utilizes various reporting systems, including spontaneous reporting, electronic health records, and patient registries. Â
- Aims to identify potential safety signals that may indicate a need for further investigation. Â
- Ensures compliance with regulatory requirements set by agencies like the FDA and EMA. Â
- Engages healthcare professionals and patients in reporting adverse events to enhance data collection. Â
- Supports risk management strategies to minimize the occurrence of adverse events and improve patient safety. Â
By collaborating with Rapid Innovation, clients can expect enhanced drug safety monitoring, leading to improved patient trust and satisfaction, which translates into a higher ROI.
2.4. Clinical Decision Support
Clinical decision support (CDS) refers to tools and systems that provide healthcare professionals with knowledge and patient-specific information to enhance decision-making in patient care.
Our innovative CDS solutions integrate seamlessly into existing healthcare workflows, providing timely and relevant information that empowers healthcare professionals to make informed decisions.
- Integrates evidence-based guidelines and clinical pathways into the healthcare workflow. Â
- Offers alerts and reminders for preventive care, medication interactions, and diagnostic tests. Â
- Enhances diagnostic accuracy by providing relevant patient data and clinical evidence. Â
- Supports personalized medicine by tailoring recommendations based on individual patient characteristics. Â
- Aims to reduce errors and improve patient outcomes through timely and relevant information. Â
- Facilitates communication among healthcare teams, ensuring that all members have access to the same information. Â
By choosing Rapid Innovation as your partner, clients can expect to see a reduction in medical errors and improved patient outcomes, ultimately leading to a more efficient healthcare system and a substantial ROI.
In summary, partnering with Rapid Innovation means leveraging our expertise in AI medical image analysis to achieve your healthcare goals efficiently and effectively, ensuring that you stay ahead in a rapidly evolving industry.
Refer to the image based on the Population Health Management concepts outlined above:

2.5. Patient Engagement and Experience
Patient engagement refers to the involvement of patients in their own healthcare processes. It emphasizes the importance of patients being active participants in their treatment and decision-making.
- Enhances communication between patients and healthcare providers, fostering a collaborative environment that leads to improved trust and transparency. Â
- Leads to better health outcomes as patients are more likely to adhere to treatment plans, ultimately resulting in higher patient retention and satisfaction rates. Â
- Involves the use of technology, such as patient portals and mobile apps, to facilitate access to health information, making it easier for patients to manage their health proactively. Digital patient engagement tools are essential in this process. Â
- Encourages shared decision-making, where patients and providers collaborate on treatment options, ensuring that care aligns with patient preferences and values. Increasing patient engagement is crucial for this collaboration. Â
- Improves patient satisfaction, as engaged patients feel more valued and understood, which can translate into positive word-of-mouth referrals and increased patient loyalty. The importance of patient engagement in healthcare cannot be overstated. Â
- Involves feedback mechanisms, such as surveys and focus groups, to gather patient insights and experiences, allowing healthcare providers to continuously refine their services. Patient engagement services can facilitate this feedback process. Â
- Can reduce healthcare costs by preventing complications through better self-management, ultimately leading to a more efficient allocation of resources and improved ROI for healthcare organizations. Improving patient engagement can significantly contribute to this goal.
2.6. Medical Research and Literature Mining
Medical research and literature mining involve the systematic analysis of existing medical literature to extract valuable insights and data that can inform clinical practices and research.
- Utilizes advanced algorithms and artificial intelligence to sift through vast amounts of medical literature, significantly reducing the time and effort required for researchers to find relevant information. Â
- Helps identify trends, gaps, and emerging areas of research that require further exploration, enabling organizations to stay ahead of the curve in medical advancements. Â
- Facilitates the discovery of new treatments and therapies by analyzing existing studies and clinical trials, which can lead to innovative solutions and improved patient outcomes. Â
- Supports evidence-based medicine by providing healthcare professionals with up-to-date information, ensuring that clinical decisions are grounded in the latest research. Â
- Enhances collaboration among researchers by making relevant studies more accessible, fostering a culture of shared knowledge and innovation. Â
- Can lead to the development of clinical guidelines based on comprehensive literature reviews, which can standardize care and improve overall healthcare quality. Â
- Involves the use of databases like PubMed and Cochrane Library for comprehensive searches, ensuring that healthcare providers have access to the most relevant and reliable information. Â
2.7. Healthcare Quality Improvement
Healthcare quality improvement focuses on enhancing the effectiveness, safety, and efficiency of healthcare services. It aims to provide better patient care and outcomes through systematic changes.
- Involves continuous monitoring and evaluation of healthcare processes and outcomes, allowing organizations to identify areas for improvement and implement necessary changes. Â
- Utilizes data-driven approaches to identify areas needing improvement, ensuring that decisions are based on solid evidence rather than assumptions. Â
- Encourages the implementation of best practices and evidence-based interventions, which can lead to more consistent and reliable patient care. Â
- Engages multidisciplinary teams to foster collaboration and innovation in care delivery, leveraging diverse expertise to enhance service quality. Â
- Incorporates patient feedback to ensure that services meet their needs and expectations, ultimately leading to higher patient satisfaction and loyalty. Â
- Uses frameworks like Plan-Do-Study-Act (PDSA) cycles to test and implement changes, allowing for iterative improvements that can adapt to evolving healthcare landscapes. Â
- Aims to reduce medical errors and improve patient safety through standardized protocols, which can significantly enhance the overall quality of care and reduce liability risks for healthcare organizations. Â
By partnering with Rapid Innovation, clients can expect to achieve greater ROI through enhanced patient engagement, informed decision-making in medical research, and continuous quality improvement in healthcare services. Our expertise in AI and blockchain technology empowers organizations to streamline processes, reduce costs, and ultimately deliver superior patient care, including through platforms like Twistle Health Catalyst and Luma patient engagement solutions.
Refer to the image for a visual representation of patient engagement and experience in healthcare.
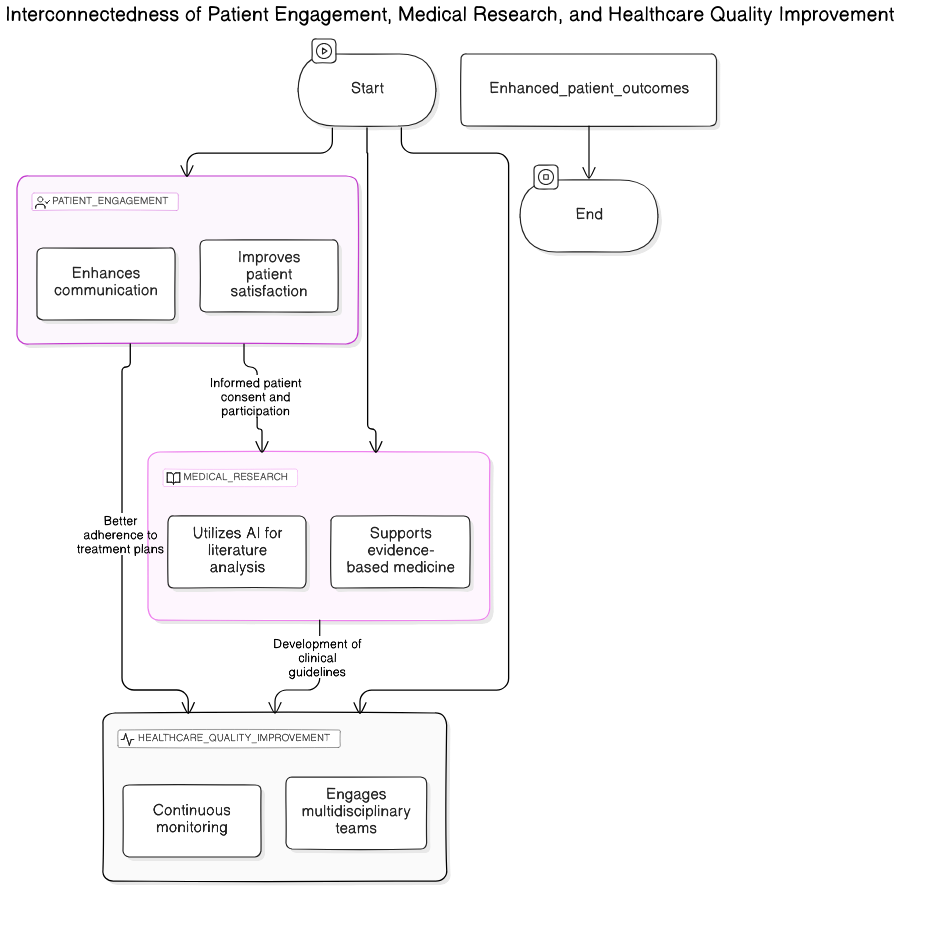
3. Overcoming Challenges in Healthcare NLP
Natural Language Processing (NLP) in healthcare presents unique challenges that need to be addressed to harness its full potential. Two significant challenges are healthcare NLP challenges related to data privacy and security, as well as the heterogeneity of unstructured data. At Rapid Innovation, we specialize in providing tailored solutions that help healthcare organizations navigate these challenges effectively, ensuring they achieve greater ROI through our expertise.
3.1. Data Privacy and Security

‍
Data privacy and security are paramount in healthcare due to the sensitive nature of patient information. The following points highlight the challenges and considerations in this area, along with how Rapid Innovation can assist:
- Regulatory Compliance: Healthcare organizations must comply with regulations such as HIPAA (Health Insurance Portability and Accountability Act) in the U.S. Our team ensures that NLP systems we develop adhere to these guidelines, minimizing the risk of non-compliance and potential fines.
- Data Anonymization: To protect patient identities, data used in NLP applications often needs to be anonymized. Rapid Innovation employs advanced techniques to anonymize data effectively, allowing organizations to utilize valuable insights without compromising patient confidentiality.
- Cybersecurity Threats: Healthcare data is a prime target for cyberattacks. We implement robust security measures, including encryption and access controls, to safeguard sensitive patient information, thereby enhancing trust and reliability in your NLP systems.
- Informed Consent: Patients should be informed about how their data will be used in NLP applications. Our solutions include user-friendly consent management systems that ensure compliance with ethical standards while maintaining patient trust.
- Data Sharing Limitations: Collaborations between healthcare institutions can be hindered by concerns over data privacy. Rapid Innovation facilitates secure data-sharing frameworks that allow organizations to collaborate effectively while adhering to privacy regulations, thus enhancing the quality of NLP models.
3.2. Unstructured Data Heterogeneity
Unstructured data heterogeneity refers to the diverse formats and sources of unstructured data in healthcare, which can complicate NLP efforts. Key points include how we can help overcome these challenges:
- Variety of Data Sources: Healthcare data comes from various sources, including clinical notes, discharge summaries, lab reports, and patient feedback. Our NLP solutions are designed to integrate and standardize data from these diverse sources, creating a unified model that enhances analysis and decision-making.
- Inconsistent Terminology: Medical terminology can vary widely between institutions and even among individual practitioners. Rapid Innovation develops customizable NLP models that can adapt to specific terminologies, reducing misunderstandings and inaccuracies.
- Data Quality Issues: Unstructured data often contains errors, such as typos or incomplete information. We employ advanced data cleaning and validation techniques to ensure high-quality data, which is essential for effective NLP and ultimately leads to better patient outcomes.
- Contextual Understanding: NLP systems must be able to understand the context in which terms are used. Our solutions incorporate sophisticated algorithms that enhance contextual understanding, minimizing misinterpretation and improving the accuracy of insights derived from the data.
- Integration with Structured Data: Healthcare data is often a mix of structured (e.g., databases) and unstructured formats. Rapid Innovation specializes in creating seamless integration solutions that allow for comprehensive analysis across both data types, providing a holistic view of patient information.
- Scalability Issues: As healthcare data continues to grow, NLP systems must be scalable to handle increasing volumes of unstructured data. Our scalable infrastructure and advanced algorithms ensure that your NLP systems can grow alongside your data needs, maximizing efficiency and ROI.
By partnering with Rapid Innovation, healthcare organizations can expect not only to overcome these healthcare NLP challenges but also to achieve greater efficiency, improved patient care, and enhanced return on investment. Our expertise in AI and blockchain development positions us as a trusted advisor in navigating the complexities of healthcare NLP.
3.3. Model Interpretability and Clinical Adoption
- Model interpretability refers to the ability to understand how a machine learning model makes its predictions. In healthcare, interpretability is crucial because clinicians need to trust the model's recommendations, and understanding model decisions can help in diagnosing and treating patients effectively. At Rapid Innovation, we prioritize model interpretability in healthcare to ensure that our clients can leverage AI solutions that are not only effective but also trustworthy.
- Key aspects of model interpretability include: Â
- Transparency: Clear explanations of how inputs affect outputs, which we facilitate through user-friendly dashboards.
- Justifiability: The ability to provide reasons for specific predictions, ensuring clinicians can make informed decisions.
- Trustworthiness: Clinicians are more likely to adopt models they understand and trust, which we achieve by incorporating interpretability techniques in our development process.
- Techniques to enhance interpretability: Â
- Feature importance analysis: Identifying which features most influence predictions, allowing clients to focus on critical data points.
- Local interpretable model-agnostic explanations (LIME): Providing insights into individual predictions, enhancing user confidence in AI recommendations.
- SHAP (SHapley Additive exPlanations): A method to explain the output of any machine learning model, which we integrate into our solutions to boost transparency.
- Challenges in achieving interpretability: Â
- Complex models (e.g., deep learning) often act as "black boxes." Our team at Rapid Innovation specializes in demystifying these models to ensure clarity.
- Balancing accuracy and interpretability can be difficult, but we work closely with clients to find the right equilibrium.
- Clinical adoption is influenced by: Â
- Regulatory approval: Models must meet standards set by health authorities, and we guide clients through this process.
- Integration into existing workflows: Solutions must fit seamlessly into clinical practices, which we prioritize in our development.
- Training and education: Clinicians need to be trained to use these models effectively, and we provide comprehensive training programs.
- Successful examples of interpretability leading to adoption include: Â
- Models that provide clear visualizations of risk factors for diseases, which we have successfully implemented for several healthcare clients.
- Tools that explain treatment recommendations based on patient data, enhancing clinician confidence and improving patient outcomes. Additionally, our expertise in transformer model development ensures that we can create advanced models that are both interpretable and clinically relevant. Furthermore, our insights on clinical decision support software benefits and implementation strategies can guide effective adoption in clinical settings.
3.4. Integration with Clinical Workflows
- Integration of NLP tools into clinical workflows is essential for maximizing their utility. Key considerations for effective integration include: Â
- Compatibility with existing electronic health record (EHR) systems: Our solutions are designed to work seamlessly with popular EHR platforms.
- User-friendly interfaces that require minimal training, ensuring quick adoption by clinical staff.
- Real-time data processing to provide immediate insights, which is critical for timely decision-making.
- Benefits of integration: Â
- Streamlined documentation: NLP can automate note-taking and data entry, significantly reducing administrative burdens.
- Enhanced decision support: Clinicians receive timely alerts and recommendations, improving the quality of care.
- Improved patient outcomes: Faster access to relevant information can lead to better care, ultimately driving higher ROI for healthcare providers.
- Challenges faced during integration: Â
- Resistance to change: Clinicians may be hesitant to adopt new technologies, but our change management strategies help ease this transition.
- Data privacy concerns: Ensuring patient data is secure and compliant with regulations is a top priority for us.
- Technical barriers: Integrating NLP tools with legacy systems can be complex, but our experienced team navigates these challenges effectively.
- Strategies for successful integration: Â
- Involve clinicians in the development process to ensure tools meet their needs, fostering a sense of ownership.
- Provide ongoing support and training to ease the transition, ensuring that staff feel confident using new tools.
- Pilot programs to test tools in real-world settings before full-scale implementation, allowing for adjustments based on feedback.
- Examples of successful integration include: Â
- NLP systems that assist in coding for billing and insurance claims, streamlining revenue cycles for healthcare organizations.
- Tools that extract relevant clinical information from unstructured data in EHRs, enhancing data usability and decision-making.
4. The Future of NLP in Healthcare
- The future of NLP in healthcare is promising, with advancements expected in several areas. Key trends shaping the future include: Â
- Increased use of AI and machine learning: More sophisticated algorithms will enhance NLP capabilities, which we are at the forefront of developing.
- Expansion of telehealth: NLP can improve virtual consultations by analyzing patient interactions, a service we are actively enhancing for our clients.
- Personalized medicine: NLP can help tailor treatments based on individual patient data, driving better health outcomes.
- Potential applications of NLP in healthcare: Â
- Clinical decision support: Providing evidence-based recommendations to clinicians, which we integrate into our solutions for enhanced patient care.
- Patient engagement: Chatbots and virtual assistants can enhance communication with patients, improving satisfaction and adherence.
- Research and drug discovery: AI-based literature and clinical data identification, a service we offer to pharmaceutical clients.
- Challenges that may arise: Â
- Ethical considerations: Ensuring fairness and avoiding bias in NLP models is a priority for us as we develop solutions.
- Data quality: The effectiveness of NLP depends on the quality of the input data, and we work with clients to ensure high standards.
- Regulatory hurdles: Navigating the complex landscape of healthcare regulations is a challenge we help clients overcome.
- Future innovations to watch for: Â
- Development of more robust multilingual NLP systems to cater to diverse populations, which we are actively pursuing.
- Enhanced capabilities for understanding context and sentiment in patient communications, improving engagement strategies.
- Integration of NLP with other technologies, such as wearables and IoT devices, for comprehensive patient monitoring, a key area of our research and development.
- Overall, the evolution of NLP in healthcare holds the potential to transform patient care, improve operational efficiency, and drive research advancements. By partnering with Rapid Innovation, clients can expect to achieve greater ROI through our cutting-edge solutions and expert guidance.
4.1. Emerging Trends and Innovations
The healthcare landscape is rapidly evolving, driven by technological advancements and innovative practices. At Rapid Innovation, we understand these healthcare technology trends and leverage them to help our clients achieve their goals efficiently and effectively. Key trends include:
- Telemedicine: The rise of telehealth services has made healthcare more accessible. Patients can consult with healthcare providers remotely, reducing travel time and increasing convenience. By integrating telemedicine solutions, we help healthcare organizations expand their reach and improve patient satisfaction, ultimately leading to greater ROI.
- Artificial Intelligence (AI): AI is being utilized for diagnostics, predictive analytics, and personalized medicine. Our expertise in AI development for elderly patient care allows us to create machine learning algorithms that analyze vast amounts of data to identify patterns and improve treatment plans. This not only enhances clinical outcomes but also optimizes resource allocation, driving down costs. The healthcare AI trends indicate a growing reliance on these technologies for better patient care.
- Wearable Technology: Devices like smartwatches and fitness trackers monitor health metrics in real-time. By developing applications that utilize this data, we empower healthcare providers to promote proactive health management, leading to early detection of health issues and reduced hospital admissions. These health tech trends are reshaping how patients engage with their health.
- Blockchain: This technology is being explored for secure patient data management. Our blockchain solutions enhance data integrity and privacy, ensuring that patient information is protected. This not only builds trust with patients but also reduces the risk of costly data breaches, aligning with current technology trends in healthcare.
- 3D Printing: Innovations in 3D printing are enabling the creation of customized prosthetics and implants, tailored to individual patient needs. We assist clients in implementing 3D printing technologies, which can significantly reduce production costs and improve patient outcomes. This is part of the emerging technologies in the healthcare industry.
- Genomics and Personalized Medicine: AIÂ Advances in genomics allow for treatments tailored to an individual's genetic makeup, improving efficacy and reducing side effects. Our consulting services help healthcare organizations integrate genomic data into their treatment protocols, enhancing patient care and satisfaction. These healthcare innovation trends are crucial for the future of personalized treatment.
4.2. Transforming Clinical Outcomes and Patient Experience
Innovations in healthcare are significantly improving clinical outcomes and enhancing the patient experience. At Rapid Innovation, we focus on delivering solutions that drive these changes, including:
- Patient-Centered Care: Healthcare systems are increasingly focusing on patient engagement and satisfaction. Our solutions encourage shared decision-making and personalized treatment plans, leading to improved patient loyalty and retention. The trends in healthcare information technology support this shift towards more personalized care.
- Data-Driven Decision Making: The use of electronic health records (EHRs) and data analytics helps healthcare providers make informed decisions, leading to better patient outcomes. We provide analytics tools that empower clients to harness their data effectively, resulting in enhanced operational efficiency.
- Integrated Care Models: Coordinated care among various healthcare providers ensures that patients receive comprehensive treatment, reducing the risk of complications and hospital readmissions. Our consulting services help organizations develop integrated care strategies that improve patient outcomes and reduce costs.
- Enhanced Communication: Digital platforms facilitate better communication between patients and providers, allowing for timely updates and follow-ups. We develop user-friendly communication tools that enhance patient engagement and satisfaction.
- Remote Monitoring: Continuous monitoring of patients with chronic conditions through telehealth and wearable devices helps in early intervention, reducing hospital visits. Our solutions enable healthcare providers to implement effective remote patient monitoring trends, leading to better health outcomes.
- Patient Education: Providing patients with access to educational resources empowers them to take charge of their health, leading to improved adherence to treatment plans. We create educational platforms that enhance patient knowledge and engagement, ultimately improving clinical results.
4.3. Empowering Healthcare Professionals and Researchers
The integration of technology and innovative practices is also empowering healthcare professionals and researchers. Key aspects include:
- Access to Information: Digital tools and databases provide healthcare professionals with instant access to the latest research and clinical guidelines, enhancing their knowledge and decision-making capabilities. Our solutions ensure that healthcare teams are always informed, leading to better patient care.
- Collaboration Tools: Platforms that facilitate collaboration among healthcare teams improve communication and teamwork, leading to better patient care. We develop collaborative tools that enhance workflow efficiency and foster a culture of teamwork.
- Training and Development: Online training programs and simulations allow healthcare professionals to enhance their skills and stay updated with the latest advancements in their field. Our training solutions ensure that staff are well-equipped to deliver high-quality care, improving overall service delivery.
- Research Opportunities: Big data analytics and AI are enabling researchers to conduct more comprehensive studies, leading to breakthroughs in treatment and care. We assist research institutions in leveraging data analytics to uncover insights that drive innovation.
- Workforce Efficiency: Automation of administrative tasks allows healthcare professionals to focus more on patient care rather than paperwork, improving job satisfaction and productivity. Our automation solutions streamline operations, leading to cost savings and improved service delivery.
- Interdisciplinary Approaches: Collaboration between different specialties fosters innovation and leads to more holistic treatment approaches, benefiting both patients and healthcare providers. We facilitate interdisciplinary collaboration, ensuring that our clients can deliver comprehensive care that meets diverse patient needs.
By partnering with Rapid Innovation, healthcare organizations can expect to achieve greater ROI through enhanced operational efficiency, improved patient outcomes, and increased patient satisfaction. Our expertise in AI and blockchain development positions us as a valuable ally in navigating the complexities of the evolving healthcare landscape, including the current healthcare tech trends and future trends in healthcare technology.