Table Of Contents
Category
Artificial Intelligence
Computer Vision
CRM
Security
IoT
AIML
Blockchain
1. Understanding the Challenges of Handling Financial Documents
Managing financial documents is a critical aspect of any business operation. However, it comes with its own set of challenges that can hinder efficiency and accuracy. Financial institutions process an average of 800 million pages of documents annually, according to a study by AIIM (Association for Intelligent Information Management). Manual document processing is not only time-consuming but also error-prone, with an average error rate of 3.6% in data entry tasks, as reported by the Bureau of Labor Statistics.
1.1. What Issues Do Companies Face When Managing Large Volumes of Financial Documents?
Companies often deal with a significant amount of financial documentation, which can lead to various issues, including:
- Data Overload: The sheer volume of documents can overwhelm staff, making it difficult to locate specific information quickly.
- Increased Risk of Errors: Manual data entry and processing can lead to mistakes, which may result in financial discrepancies and compliance issues.
- Inefficient Retrieval Systems: Traditional filing systems can be cumbersome, leading to wasted time searching for documents.
- Compliance Challenges: Keeping up with regulatory requirements can be difficult, especially when documents are not organized or easily accessible.
- Security Concerns: Financial documents often contain sensitive information, making them a target for data breaches if not properly secured.
- Collaboration Difficulties: Sharing documents among teams can be challenging, especially if files are stored in different locations or formats.
- Cost Implications: The costs associated with printing, storing, and managing physical documents can add up significantly over time.
1.2. Why Traditional Methods Fall Short in Processing Financial Data?
Traditional methods of handling financial documents often fail to meet the demands of modern businesses due to several limitations:
- Manual Processes: Relying on manual data entry and processing is time-consuming and prone to human error.
- Lack of Automation: Traditional systems do not leverage automation, which can streamline workflows and reduce processing times.
- Inflexibility: Older systems may not easily adapt to new technologies or changing business needs, leading to inefficiencies in financial document management.
- Poor Integration: Many traditional methods do not integrate well with other software systems, creating silos of information that hinder data analysis, especially in financial services document management.
- Limited Accessibility: Physical documents can only be accessed in specific locations, making remote work and collaboration difficult.
- High Storage Costs: Storing large volumes of paper documents requires significant physical space and resources, which can be costly, especially in the context of financial document storage.
- Inadequate Security Measures: Traditional filing systems may lack the robust security features needed to protect sensitive financial information from unauthorized access, a critical concern in enterprise content management for financial services.
By understanding these challenges, companies can begin to explore more efficient and secure methods for managing their financial documents. At Rapid Innovation, we specialize in leveraging AI technologies for banking and finance to transform the way businesses handle their financial documentation. Our solutions, including document management systems for financial services, not only enhance efficiency but also significantly reduce the risk of errors and compliance issues, ultimately leading to greater ROI for our clients. Partnering with us means you can expect streamlined processes, improved data security, and cost savings that will empower your organization to thrive in a competitive landscape.
Refer to the image for a visual representation of the challenges in handling financial documents.

2. Introducing AI-Powered Financial Document Processing
AI-powered financial document processing refers to the use of artificial intelligence technologies to automate the handling, analysis, and management of financial documents. This innovation is transforming how organizations manage their financial data, leading to increased efficiency, accuracy, and cost savings. McKinsey estimates that AI could potentially save the banking industry $447 billion by 2023, with a significant portion attributed to improved document processing
2.1. What is AI-Powered Document Processing and How Does It Work?
AI-powered financial document processing involves several advanced technologies that work together to streamline the handling of financial documents. These technologies include:
- Optical Character Recognition (OCR): This technology converts different types of documents, such as scanned paper documents or PDFs, into editable and searchable data.
- Natural Language Processing (NLP): NLP enables machines to understand and interpret human language, allowing them to analyze the content of financial documents effectively.
- Machine Learning (ML): ML algorithms learn from data patterns and improve over time, enhancing the accuracy of document classification and data extraction.
The process typically involves the following steps:
- Data Capture: Documents are scanned or uploaded into the system.
- Data Extraction: AI algorithms extract relevant information, such as names, dates, amounts, and other key data points.
- Data Validation: The extracted data is cross-checked against predefined rules or databases to ensure accuracy.
- Data Integration: Validated data is integrated into existing financial systems or databases for further analysis and reporting.
This automated approach reduces manual effort, minimizes errors, and accelerates the processing time for financial documents.
2.2. Key Features That Make AI a Game-Changer in Financial Document Management
AI-powered financial document processing offers several key features that significantly enhance document management capabilities:
- Increased Accuracy: AI systems can achieve higher accuracy rates in data extraction compared to manual processes, reducing the risk of human error.
- Speed and Efficiency: Automation allows for rapid processing of large volumes of documents, enabling organizations to respond quickly to financial inquiries and reporting needs.
- Cost Savings: By reducing the need for manual labor and minimizing errors, organizations can save on operational costs associated with document management.
- Scalability: AI solutions can easily scale to handle increasing volumes of documents as a business grows, without a proportional increase in resources.
- Enhanced Compliance: AI can help ensure that financial documents adhere to regulatory requirements by automating compliance checks and maintaining accurate records.
- Improved Data Insights: Advanced analytics capabilities allow organizations to derive valuable insights from financial documents, aiding in decision-making and strategic planning.
- User-Friendly Interfaces: Many AI-powered solutions come with intuitive interfaces that make it easy for users to interact with the system and access information quickly.
These features collectively make AI-powered financial document processing a transformative force in financial document management, enabling organizations to operate more efficiently and effectively in a competitive landscape. By partnering with Rapid Innovation, clients can leverage these advanced capabilities to achieve greater ROI, streamline their operations, and enhance their overall financial management processes.
Refer to the image below for a visual representation of AI-powered financial document processing.
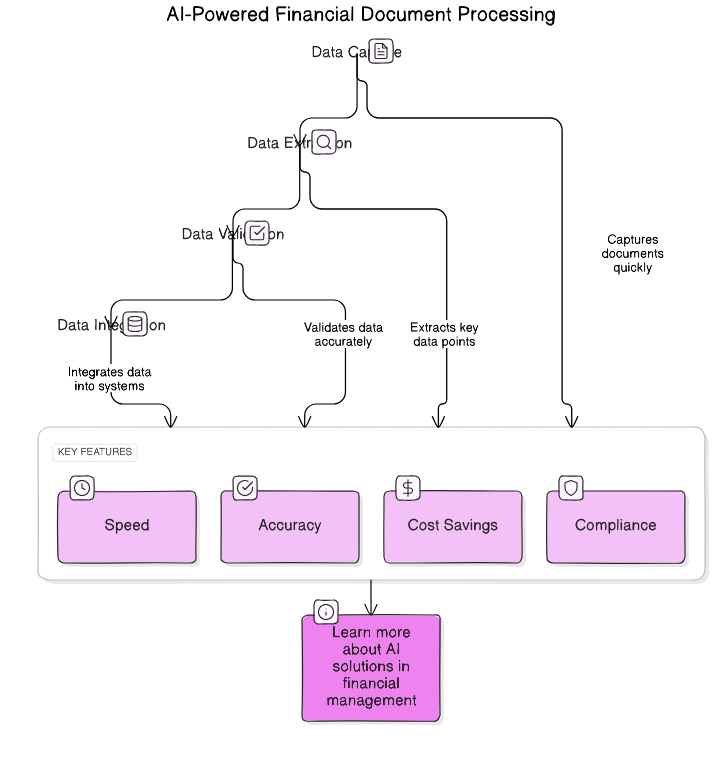
3. Exploring the Types of Financial Documents AI Can Handle
Artificial Intelligence (AI) is revolutionizing the financial sector by automating the processing of various financial documents. This not only enhances efficiency but also reduces human error and operational costs. Understanding the types of financial documents that AI can handle is crucial for organizations looking to leverage this technology.
3.1. Which Financial Documents Benefit Most from AI Automation?
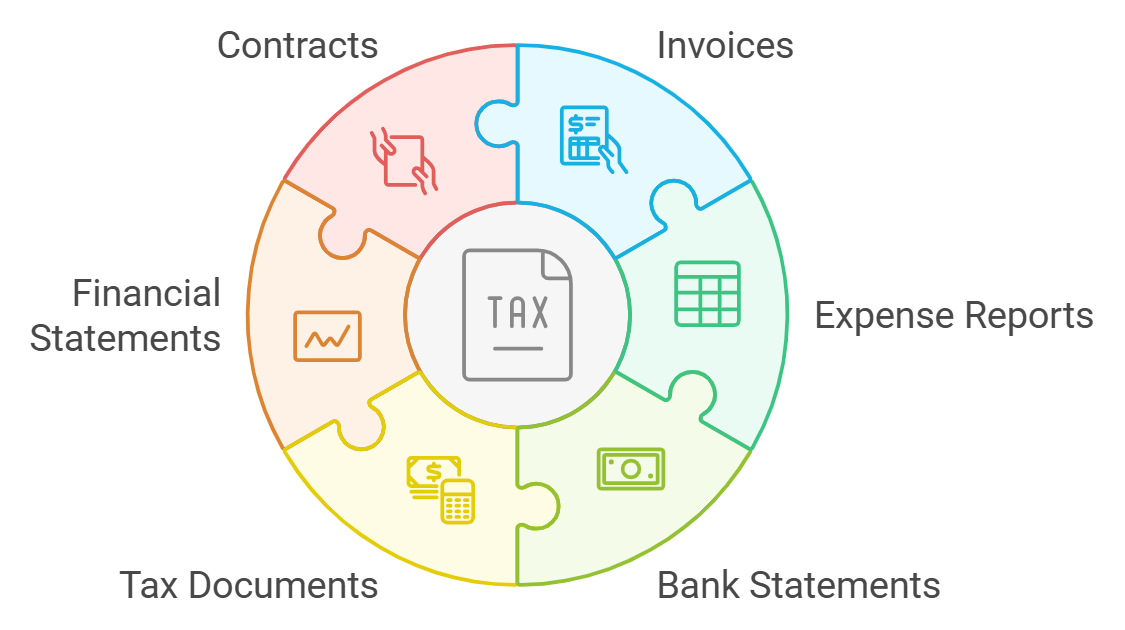
‍
AI automation can significantly improve the handling of several types of financial documents. The following categories benefit the most:
- Invoices: AI can extract data from invoices, such as vendor names, amounts, and due dates. Automation reduces the time spent on manual data entry and minimizes errors.
- Expense Reports: AI can analyze receipts and categorize expenses automatically, streamlining the reimbursement process for employees.
- Bank Statements: AI can reconcile bank statements with internal records, helping to identify discrepancies and ensuring accurate financial reporting.
- Tax Documents: AI can assist in organizing and preparing tax documents, as well as analyze tax codes and regulations to ensure compliance.
- Financial Statements: AI can generate and analyze balance sheets, income statements, and cash flow statements, aiding in real-time financial analysis and decision-making.
- Contracts: AI can review contracts for compliance and risk assessment, extracting key terms and conditions for easier management.
These documents benefit from AI automation due to their repetitive nature and the need for accuracy in financial operations. By automating these processes, organizations can save time and resources while improving accuracy.
3.2. From Invoices to Contracts: Documents That AI Can Process?
AI technology is capable of processing a wide range of financial documents, including ai financial document processing, each with unique characteristics and requirements. Here are some key documents that AI can effectively handle:
- Invoices: AI can read and interpret various invoice formats, automating approval workflows and payment processing.
- Purchase Orders: AI can match purchase orders with invoices to ensure accuracy, helping to prevent fraud and overpayments.
- Receipts: AI can digitize and categorize receipts for expense tracking, extracting relevant data for accounting purposes.
- Contracts: AI can analyze contract language for compliance and risk factors, flagging unusual terms or conditions for further review.
- Financial Reports: AI can generate reports from raw data, providing insights into financial performance and automating the distribution of these reports to stakeholders.
- Loan Applications: AI can process loan applications by analyzing financial documents submitted by applicants, assessing creditworthiness and streamlining the approval process.
- Tax Returns: AI can assist in preparing and filing tax returns by organizing necessary documents and analyzing tax implications for various financial decisions.
- Audit Reports: AI can analyze audit reports for compliance and risk management, identifying areas that require further investigation.
The ability of AI to process these documents efficiently leads to improved accuracy, faster turnaround times, and enhanced compliance with regulations. Organizations that adopt AI for document processing can expect to see significant improvements in their financial operations. By partnering with Rapid Innovation, clients can leverage our expertise in AI and Blockchain to achieve greater ROI, streamline their processes, and enhance their overall operational efficiency.
For more insights on how AI is changing the industry, check out how AI in accounting is changing the industry, and learn about AI indue diligence
Refer to the image for a visual representation of the types of financial documents AI can handle.

4. The Role of AI in Streamlining Financial Document Workflows
Artificial Intelligence (AI) is transforming how financial institutions manage their document workflows, offering a powerful solution for enhancing efficiency and reducing costs. Through targeted AI development for financial compliance, repetitive tasks are automated, and data processing capabilities are optimized, allowing organizations to streamline operations while adhering to regulatory standards. Machine learning and natural language processing are integrated into financial document management systems to analyze large data volumes swiftly, enabling faster and more accurate decision-making. Additionally, AI continuously learns from historical data, improving its accuracy and compliance efficacy over time.
4.1. How AI Automates and Enhances Document Processing?

‍
AI automates various aspects of document processing, making it more efficient and less prone to errors.
- Data Extraction: AI can automatically extract relevant information from financial documents, such as invoices, contracts, and tax forms, significantly reducing the need for manual data entry.
- Classification: AI algorithms can classify documents based on their content, simplifying the organization and retrieval of information.
- Workflow Automation: AI can streamline workflows by routing documents to the appropriate departments or individuals based on predefined rules, ensuring a smoother operational flow.
- Integration with Existing Systems: AI can be seamlessly integrated with existing financial software, enhancing their capabilities without necessitating a complete overhaul.
- Continuous Learning: Machine learning models can improve over time by learning from new data, leading to enhanced performance in document processing tasks.
4.2. The Impact of AI on Accuracy, Speed, and Reducing Human Error?
The implementation of AI in financial document workflows has a significant impact on accuracy, speed, and the reduction of human error.
- Increased Accuracy: AI systems can analyze data with a high degree of precision, minimizing the chances of errors that often occur with manual processing. Studies indicate that AI can achieve accuracy rates exceeding 90% in data extraction tasks.
- Faster Processing Times: AI can process documents in a fraction of the time it takes a human. For example, while a human might take hours to review and input data from a set of documents, AI can complete the same task in mere minutes.
- Reduction of Human Error: By automating repetitive tasks, AI significantly reduces the likelihood of human mistakes, such as typos or misinterpretations of data. This is particularly crucial in financial settings where accuracy is paramount.
- Cost Efficiency: With faster processing and fewer errors, organizations can save on labor costs and mitigate the financial impact of mistakes.
- Enhanced Compliance: AI can help ensure that documents meet regulatory requirements by flagging inconsistencies or missing information, thereby reducing the risk of compliance issues.
In conclusion, AI is playing a pivotal role in streamlining financial document workflows by automating processes, enhancing accuracy, and significantly reducing human error. As technology continues to evolve, its impact on the financial sector is expected to grow, leading to even more efficient and effective document management solutions. By partnering with Rapid Innovation, clients can leverage these advancements to achieve greater ROI, enhance operational efficiency, and stay ahead in a competitive landscape. AI in financial document workflows is set to become an essential component of modern financial operations.
5. How AI-Powered Financial Document Processing Works
AI-powered financial document processing leverages advanced technologies to automate the handling of financial documents. This process enhances efficiency, accuracy, and speed in managing large volumes of data. By utilizing machine learning, natural language processing, and optical character recognition, AI systems can interpret and process financial documents with minimal human intervention.
5.1. What Are the Core Steps in AI Document Processing?
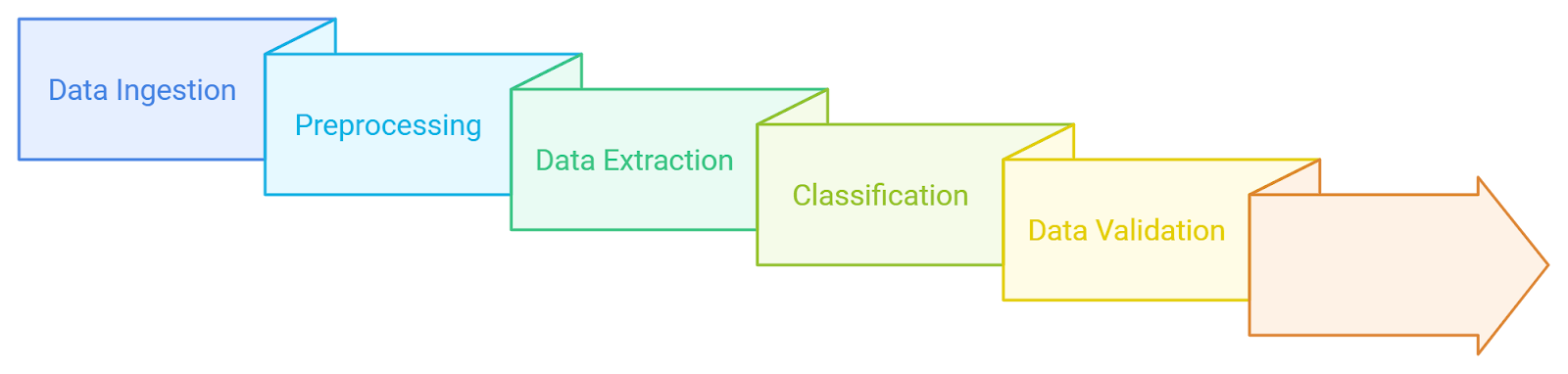
‍
The core steps in AI document processing typically include:
- Data Ingestion: Collecting documents from various sources such as emails, cloud storage, or physical scans, and ensuring documents are in a format suitable for processing, like PDFs or images.
- Preprocessing: Cleaning and preparing the data for analysis, which includes converting scanned documents into machine-readable formats using Optical Character Recognition (OCR).
- Data Extraction: Identifying and extracting relevant information from documents by using AI algorithms to locate key data points like names, dates, amounts, and account numbers.
- Classification: Categorizing documents based on their type (e.g., invoices, contracts, bank statements) and employing machine learning models to improve classification accuracy over time.
- Data Validation: Verifying the accuracy of extracted data against predefined rules or databases and implementing checks to ensure data integrity and compliance with regulations.
- Data Analysis: Analyzing the extracted data for insights and trends, utilizing AI tools to generate reports or dashboards for decision-making.
- Integration: Integrating processed data into existing financial systems or databases to ensure seamless data flow for further processing or analysis.
5.2. How AI Extracts, Classifies, and Analyzes Financial Data?
AI employs various techniques to extract, classify, and analyze financial data effectively:
- Natural Language Processing (NLP): Enables the system to understand and interpret human language in documents, helping in extracting contextually relevant information from unstructured text. For more on NLP, check out Natural Language Processing: From Fundamentals to Advanced Applications.
- Machine Learning Algorithms: These algorithms are trained on historical data to recognize patterns and improve classification accuracy, continuously learning from new data to adapt to changing document formats and structures.
- Optical Character Recognition (OCR): Converts images of text into machine-readable text, which is essential for processing scanned documents and images.
- Data Enrichment: Enhances extracted data by cross-referencing with external databases, providing additional context or validation for the extracted information.
- Sentiment Analysis: Analyzes the tone and sentiment of textual data, which is useful for understanding customer feedback or market trends, and helps in making informed decisions based on qualitative data.
- Predictive Analytics: Uses historical data to forecast future trends and behaviors, assisting in risk assessment and financial planning.
- Automated Reporting: Generates reports based on analyzed data, saving time and reducing manual effort, while providing stakeholders with actionable insights in real-time.
By employing these techniques, AI-powered financial document processing not only streamlines operations but also enhances the accuracy and reliability of financial data management.
At Rapid Innovation, we understand that the efficient management of financial documents is crucial for your business's success. By partnering with us, you can expect significant improvements in operational efficiency, reduced processing times, and enhanced data accuracy, ultimately leading to greater ROI. Our expertise in AI financial document processing and blockchain technology ensures that you receive tailored solutions that align with your specific business goals, allowing you to focus on strategic initiatives while we handle the complexities of financial document processing.
6. Real-World Applications of AI in Financial Document Processing
Artificial Intelligence (AI) is transforming the financial sector by streamlining processes, enhancing accuracy, and improving decision-making. In financial document processing, AI applications such as AI in financial document processing are particularly impactful, leading to increased efficiency and reduced operational costs.
6.1. How AI Helps in Invoice Automation?

‍
AI plays a crucial role in automating the invoice processing workflow, which traditionally involves manual data entry and verification. The integration of AI technologies can significantly enhance this process.
- Data Extraction: AI algorithms can automatically extract relevant information from invoices, such as vendor names, invoice numbers, dates, and amounts. This reduces the need for manual input and minimizes errors.
- Natural Language Processing (NLP): NLP techniques enable AI systems to understand and interpret the text within invoices, allowing for better categorization and organization of financial data.
- Machine Learning: Machine learning models can learn from historical invoice data to improve accuracy over time. They can identify patterns and anomalies, which helps in predicting future invoice trends.
- Integration with ERP Systems: AI can seamlessly integrate with existing Enterprise Resource Planning (ERP) systems, ensuring that invoice data is automatically updated in real-time, facilitating better financial management.
- Cost Reduction: By automating invoice processing, organizations can significantly reduce labor costs associated with manual data entry and processing, leading to faster payment cycles and improved cash flow.
- Improved Compliance: AI can help ensure compliance with financial regulations by maintaining accurate records and providing audit trails for all transactions.
6.2. The Role of AI in Fraud Detection, Risk Management, and Compliance?
AI is increasingly being utilized in the financial sector to enhance fraud detection, risk management, and compliance efforts. Its ability to analyze vast amounts of data quickly and accurately makes it an invaluable tool. AI systems can identify potential fraud in financial documents with an accuracy rate of up to 95%, significantly higher than traditional methods.
- Fraud Detection: AI systems can analyze transaction patterns in real-time to identify unusual behavior that may indicate fraudulent activity. Machine learning algorithms can be trained on historical fraud data to recognize signs of potential fraud, allowing for proactive measures.
- Risk Management: AI can assess credit risk by analyzing a borrower’s financial history, transaction behavior, and other relevant data points. Predictive analytics can forecast potential risks, enabling organizations to take preventive actions before issues arise.
- Compliance Monitoring: AI can automate the monitoring of transactions to ensure compliance with regulatory requirements, reducing the risk of human error. Natural language processing can be used to analyze regulatory texts and ensure that financial practices align with current laws and regulations.
- Enhanced Reporting: AI can generate detailed reports on risk exposure and compliance status, providing stakeholders with insights that facilitate informed decision-making.
- Cost Efficiency: By automating fraud detection and compliance processes, organizations can reduce the costs associated with manual monitoring and investigations.
- Continuous Learning: AI systems can continuously learn from new data, improving their ability to detect fraud and assess risk over time, adapting to evolving threats and regulatory changes.
In conclusion, AI is revolutionizing financial document processing by automating tasks such as invoice processing and enhancing fraud detection, risk management, and compliance efforts. These applications not only improve efficiency but also contribute to better financial decision-making and risk mitigation. By partnering with Rapid Innovation, clients can leverage these advanced AI capabilities in financial document processing to achieve greater ROI, streamline operations, and ensure compliance, ultimately driving their business success. For more insights on how banks benefit from blockchain technology, check out the article on generative ai in banking.
7. Steps to Building an AI Model for Financial Document Processing
7.1. How to Develop an AI Model Tailored for Financial Documents?
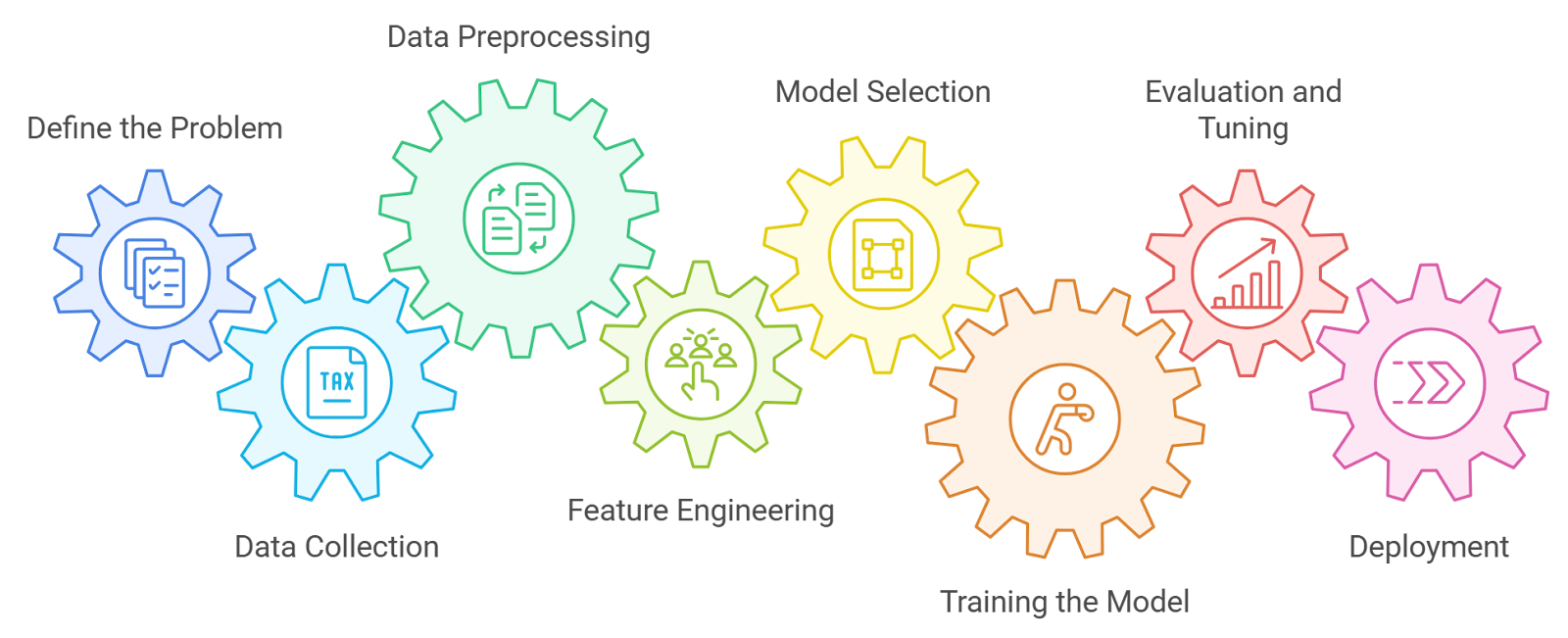
‍
Developing an AI model specifically for financial document processing involves several key steps:
- Define the Problem: Identify the specific tasks the AI model will perform, such as data extraction, classification, or sentiment analysis. Understand the types of financial documents involved, such as invoices, contracts, or bank statements.
- Data Collection: Gather a diverse dataset of financial documents to ensure the model can generalize well. Include various formats (PDFs, images, etc.) and types of documents to cover different scenarios.
- Data Preprocessing: Clean the data by removing irrelevant information and correcting errors. Convert documents into a machine-readable format, using Optical Character Recognition (OCR) if necessary.
- Feature Engineering: Identify and extract relevant features from the documents that will help the model learn. Consider using techniques like tokenization, stemming, and lemmatization for text data.
- Model Selection: Choose an appropriate machine learning or deep learning model based on the complexity of the task. Common models include Convolutional Neural Networks (CNNs) for image data and Recurrent Neural Networks (RNNs) for sequential data.
- Training the Model: Split the dataset into training, validation, and test sets to evaluate the model's performance. Use techniques like cross-validation to ensure the model is robust and not overfitting.
- Evaluation and Tuning: Assess the model's performance using metrics such as accuracy, precision, recall, and F1 score. Fine-tune hyperparameters and retrain the model as necessary to improve performance.
- Deployment: Integrate the trained model into existing financial systems or applications. Ensure the model can handle real-time data and provide outputs in a user-friendly format.
- Monitoring and Maintenance: Continuously monitor the model's performance in a production environment. Update the model periodically with new data to maintain accuracy and relevance.
7.2. What Tools and Data Are Essential for Training an AI Model?
To effectively train an AI model for financial document processing, several tools and types of data are essential:
- Data Sources: Use publicly available datasets or create proprietary datasets from internal documents. Consider sources like Kaggle or government databases for financial documents.
- Data Annotation Tools: Employ tools like Labelbox or Prodigy to annotate data, which is crucial for supervised learning. Ensure annotations are accurate to improve model training.
- Machine Learning Frameworks: Utilize frameworks such as TensorFlow, PyTorch, or Scikit-learn for building and training models. These frameworks provide pre-built functions and libraries to streamline the development process.
- OCR Tools: Implement OCR software like Tesseract or Adobe Acrobat to convert scanned documents into text. This step is vital for processing non-digital documents.
- Cloud Services: Leverage cloud platforms like AWS, Google Cloud, or Azure for scalable computing resources. These platforms offer machine learning services that can simplify model training and deployment.
- Data Storage Solutions: Use databases like MongoDB or SQL for storing and managing large volumes of financial documents. Ensure data is organized and easily accessible for training purposes.
- Version Control Systems: Implement tools like Git to manage code and model versions effectively. This practice helps in tracking changes and collaborating with team members.
- Monitoring Tools: Use monitoring solutions like Prometheus or Grafana to track model performance post-deployment. These tools can help identify issues and ensure the model remains effective over time.
By following these steps and utilizing the right tools and data, organizations can develop robust AI models tailored for financial document processing, enhancing efficiency and accuracy in handling financial information.
At Rapid Innovation, we specialize in guiding clients through this intricate process of building an AI model for financial document processing, ensuring that they achieve greater ROI by leveraging our expertise in AI and Blockchain technologies. Partnering with us means you can expect streamlined operations, reduced costs, and improved decision-making capabilities, ultimately driving your business towards success.
8. Best Practices for Developing AI Models in Finance
Developing AI models in finance requires a strategic approach to ensure they are effective, reliable, and secure. Here are some best practices to consider.
8.1. How to Ensure Accuracy, Security, and Scalability in AI Models?
Accuracy, security, and scalability are critical components of AI models in finance. Here’s how to address each aspect:
- Accuracy: Utilize high-quality, relevant data for training AI models in finance to enhance predictive performance. Implement rigorous validation techniques, such as cross-validation, to assess model performance. Continuously monitor model outputs and adjust algorithms based on real-world performance to ensure ongoing accuracy.
- Security: Incorporate robust data encryption methods to safeguard sensitive financial information. Regularly conduct security audits and vulnerability assessments to identify potential risks. Implement access controls and authentication measures to restrict data access to authorized personnel only, thereby enhancing security protocols.
- Scalability: Design AI models in finance with scalability in mind, allowing for seamless integration of additional data sources and increased computational power. Leverage cloud-based solutions to efficiently handle large datasets and complex computations. Ensure that the architecture of the AI model can accommodate future growth without significant redesign, thus promoting long-term viability.
8.2. Key Considerations for Training AI to Handle Complex Financial Data?

‍
Training AI to manage complex financial data involves several key considerations:
- Data Quality and Diversity: Ensure that the training dataset is comprehensive and includes various financial scenarios to improve model robustness. Clean and preprocess data to eliminate inconsistencies and errors that could skew results, thereby enhancing the reliability of the model.
- Feature Engineering: Identify and select relevant features that significantly impact financial outcomes, such as market trends, economic indicators, and historical performance. Employ techniques like dimensionality reduction to simplify models while retaining essential information, which can lead to more efficient processing.
- Regulatory Compliance: Stay informed about financial regulations and ensure that AI models in finance comply with legal standards, such as GDPR or SEC regulations. Implement transparency measures to explain model decisions, which is crucial for regulatory scrutiny and fosters trust with stakeholders.
- Model Interpretability: Choose algorithms that provide insights into decision-making processes, making it easier to understand how models arrive at conclusions. Utilize visualization tools to present model outputs and performance metrics clearly, facilitating better communication with stakeholders.
- Continuous Learning: Implement mechanisms for models to learn from new data and adapt to changing market conditions. Regularly update models to incorporate the latest financial trends and data patterns, ensuring that the AI remains relevant and effective.
By following these best practices, financial institutions can develop AI models in finance that are not only accurate and secure but also capable of handling the complexities of financial data effectively. Partnering with Rapid Innovation can help you implement these strategies, ensuring that your AI initiatives yield greater ROI and align with your business objectives. Our expertise in AI and Blockchain development will empower your organization to navigate the financial landscape with confidence and efficiency.
For more insights, check out our articles on AI Agents for Legal Applications: Use Cases, Framework, Benefits & Implementation, and An Introduction to AI Anomaly Detection.
9. Benefits of Using AI for Financial Document Processing
AI technology is transforming the way financial documents are processed, offering numerous benefits that enhance operational efficiency and accuracy. The integration of AI in financial document processing can lead to significant improvements in various aspects of financial operations.
9.1. How AI Reduces Costs and Improves Efficiency?
AI streamlines financial document processing, leading to cost savings and enhanced efficiency in several ways:
- Automation of Repetitive Tasks: AI can automate data entry, invoice processing, and reconciliation tasks, significantly reducing the need for manual intervention. This automation minimizes human error and speeds up processing times.
- Faster Data Processing: AI algorithms can analyze and extract data from documents at a much faster rate than humans. This rapid processing allows organizations to handle larger volumes of documents without a proportional increase in resources.
- Reduced Labor Costs: By automating routine tasks, companies can reduce the number of employees needed for document processing. This leads to lower labor costs and allows staff to focus on more strategic activities.
- Improved Workflow Management: AI can optimize workflows by identifying bottlenecks and suggesting improvements. Enhanced workflow management leads to quicker turnaround times for financial transactions.
- Scalability: AI systems can easily scale to accommodate increased document volumes without significant additional costs. This scalability is particularly beneficial during peak periods, such as tax season or financial audits.
9.2. Why AI Enhances Compliance, Accuracy, and Data Security?
AI plays a crucial role in enhancing compliance, accuracy, and data security in financial document processing:
- Improved Accuracy: AI algorithms can achieve higher accuracy rates in data extraction and processing compared to manual methods. This reduction in errors leads to more reliable financial reporting and decision-making.
- Regulatory Compliance: AI can be programmed to stay updated with changing regulations and compliance requirements. This ensures that financial documents are processed in accordance with the latest laws, reducing the risk of non-compliance. For more on this, see AI and Machine Learning for Regulatory Compliance.
- Real-time Monitoring: AI systems can continuously monitor transactions and document processing for compliance violations. This real-time oversight helps organizations quickly identify and rectify potential issues.
- Enhanced Data Security: AI can implement advanced security measures, such as anomaly detection, to identify suspicious activities. This proactive approach to security helps protect sensitive financial data from breaches.
- Audit Trails: AI can maintain detailed logs of all document processing activities, creating an audit trail. These logs are essential for compliance audits and can help organizations demonstrate adherence to regulations.
- Data Encryption and Access Control: AI systems can enforce strict access controls and data encryption protocols to safeguard sensitive information. This ensures that only authorized personnel can access critical financial documents.
By leveraging AI financial document processing, organizations can achieve significant cost reductions, improve operational efficiency, and enhance compliance and data security. The integration of AI technology is not just a trend; it is becoming a necessity for financial institutions aiming to stay competitive in a rapidly evolving landscape. At Rapid Innovation, we are committed to helping our clients harness the power of AI technology to achieve their goals efficiently and effectively, ultimately leading to greater ROI and sustainable growth. For a deeper understanding of the impact of AI on finance, check out Risk Management Reinvented: AI's Impact on Finance.
10. Key Technologies Behind AI-Powered Financial Document Processing
AI-powered financial document processing relies on several key technologies that enhance efficiency, accuracy, and data management. These technologies work together to automate the extraction, analysis, and interpretation of financial documents, significantly reducing manual effort and errors.
10.1. How Natural Language Processing (NLP) Enhances Data Understanding?
Natural Language Processing (NLP) is a branch of artificial intelligence that focuses on the interaction between computers and human language. In the context of financial document processing, NLP plays a crucial role in understanding and interpreting unstructured data.
NLP algorithms analyze text to identify key entities, such as names, dates, and monetary values, which helps in extracting relevant information from financial documents. Additionally, NLP can assess the sentiment of financial reports or news articles, providing insights into market trends and investor sentiment. The models can understand context, allowing them to differentiate between similar terms based on their usage in financial documents. Furthermore, NLP facilitates the translation of documents in multiple languages, making it easier for global financial institutions to process international documents. It can also categorize documents based on their content, enabling efficient organization and retrieval of financial information, such as tax return verification and financial statement verification.
Overall, NLP enhances the ability to process large volumes of text data quickly and accurately, which is essential in the fast-paced financial sector.
10.2. How Optical Character Recognition (OCR) and Machine Learning (ML) Drive Automation?
Optical Character Recognition (OCR) and Machine Learning (ML) are pivotal technologies that drive automation in financial document processing.
OCR Technology converts scanned documents and images into machine-readable text, enabling the extraction of data from various document formats, including PDFs, invoices, and receipts. This technology reduces the need for manual data entry, minimizing human error and saving time. For instance, it can be used for tax verification documents and balance sheet reconciliation checklists.
Machine Learning Integration involves ML algorithms that learn from historical data to improve accuracy in data extraction and classification. These algorithms can identify patterns and anomalies in financial documents, enhancing fraud detection and risk management. Moreover, ML models can adapt to new types of documents and formats, ensuring continuous improvement in processing capabilities, such as identity and tax return verification.
Automation of Workflows is achieved by combining OCR and ML, allowing for the automation of entire workflows, from data extraction to analysis and reporting. This integration streamlines processes, reduces operational costs, and increases productivity in financial institutions, particularly in tasks like balance sheet reconciliation procedures and accounting year-end closing checklists.
Together, OCR and ML create a powerful framework for automating financial document processing, enabling organizations to handle large volumes of data efficiently and accurately.
At Rapid Innovation, we leverage these advanced technologies to help our clients achieve greater ROI by streamlining their financial document processing. By automating tedious tasks, we enable organizations to focus on strategic initiatives, ultimately driving growth and profitability. Our expertise in AI and blockchain ensures that our clients benefit from cutting-edge solutions tailored to their unique needs.
When you partner with us, you can expect:
- Increased Efficiency: Our solutions significantly reduce processing time, allowing your team to allocate resources more effectively.
- Enhanced Accuracy: With advanced NLP and ML algorithms, we minimize errors, ensuring that your data is reliable and actionable, especially in areas like financial month-end closing checklists and general ledger reconciliation.
- Cost Savings: By automating workflows, we help you lower operational costs and improve your bottom line.
- Scalability: Our solutions are designed to grow with your business, accommodating increasing volumes of data without compromising performance.
- Expert Guidance: Our team of experts provides ongoing support and consultation, ensuring that you maximize the benefits of our technologies.
Let Rapid Innovation be your partner in navigating the complexities of financial document processing, and together, we can unlock new opportunities for success. For more information on our services, visit our AI Business Automation Solutions.
11. Conclusion
In summary, this conclusion serves as the final section of our discussion, encapsulating key points and reinforcing the main arguments presented. It offers a moment to reflect on the implications of our findings and the value we deliver to our clients. A well-crafted conclusion can leave a lasting impression, encouraging readers to consider how our specialized solutions, including AI development for financial document processing, can help them achieve their goals efficiently and effectively.
Key elements to include in a conclusion:
- Restate the main thesis or purpose of our discussion: Rapid Innovation is dedicated to delivering AI and blockchain development solutions that drive greater ROI for our clients.
- Summarize the main points or arguments made in the body: Our expertise in AI and blockchain allows us to tailor solutions that enhance operational efficiency, reduce costs, and foster innovation.
- Highlight the significance of the findings or arguments: By partnering with us, clients can expect not only improved performance but also a competitive edge in their respective markets.
- Suggest potential areas for further research or exploration: As technology evolves, we encourage clients to explore new applications of AI and blockchain to stay ahead of industry trends.
- End with a strong closing statement that resonates with the reader: Choosing Rapid Innovation means investing in a future where your business can thrive through cutting-edge technology and strategic insights.
Importance of a conclusion:
- It ties together the entire discussion, providing coherence and clarity about how we can support our clients' ambitions.
- It reinforces the relevance of our services and their implications for the reader's business success.
- A strong conclusion can motivate readers to take action, whether that means reaching out for a consultation or considering how our AI and blockchain development solutions can transform their operations.
Tips for writing an effective conclusion:
- Avoid introducing new information or arguments; focus on what has been discussed.
- Keep it concise and focused on the main points that highlight our value proposition.
- Use clear and impactful language to engage the reader and inspire confidence in our capabilities.
- Consider the tone and style of the overall piece to maintain consistency with our corporate identity.
Overall, the conclusion is a critical component of our communication, serving to encapsulate the discussion and leave the reader with a clear understanding of the importance of partnering with Rapid Innovation for their development needs.