Table Of Contents
Category
Artificial Intelligence
AIML
IoT
Blockchain
Retail & Ecommerce
Customer Service
1. Introduction to AI-Driven Service Personalization
AI-driven service personalization is revolutionizing the way businesses interact with their customers. By leveraging artificial intelligence, companies can tailor their services and products to meet individual customer preferences and needs. This transformation is not just about enhancing customer satisfaction; it’s about creating a more engaging and relevant experience that fosters loyalty and drives sales.
- AI technologies analyze vast amounts of data to identify patterns and preferences.
- AI-driven service personalization can occur across various touchpoints, including websites, emails, and customer service interactions.
- The goal is to create a seamless experience that feels intuitive and customized for each user.
The rise of AI in service personalization is largely due to advancements in machine learning, natural language processing, and data analytics. These technologies enable businesses to gather insights from customer behavior, allowing for more informed decision-making.
- Machine learning algorithms can predict customer needs based on historical data.
- Natural language processing helps in understanding customer inquiries and providing relevant responses.
- Data analytics offers insights into customer trends and preferences, enabling proactive service adjustments.
At Rapid Innovation, we harness these AI capabilities to help our clients achieve greater ROI. By implementing AI-driven personalization strategies, we enable businesses to deliver personalized recommendations, targeted marketing campaigns, and tailored customer support. This not only enhances the customer experience but also increases conversion rates and customer retention.
- Personalized recommendations can lead to higher sales; studies show that personalized marketing can increase conversion rates by up to 10% (source: McKinsey).
- Customers are more likely to engage with brands that offer personalized experiences, with 80% of consumers stating they are more likely to make a purchase when brands offer personalized experiences (source: Epsilon).
- Businesses that prioritize personalization can see a significant boost in customer loyalty, with 44% of consumers likely to become repeat buyers after a personalized shopping experience (source: Segment).
In summary, AI-driven service personalization is a game-changer for businesses looking to enhance customer experience. By utilizing advanced technologies, companies can create tailored interactions that resonate with their audience, ultimately leading to increased satisfaction and loyalty. At Rapid Innovation, we are committed to guiding our clients through this transformative journey, ensuring they leverage AI effectively to meet their business goals. For more information on how we can assist you, check out our AI customer service agent services.
Refer to the image for a visual representation of AI-driven service personalization concepts.

1.1. Evolution of Service Personalization
Service personalization has undergone significant transformation over the years, evolving from basic customer service interactions to highly tailored experiences.
- Early Days: Initially, service personalization was limited to face-to-face interactions where businesses relied on personal relationships. Customers received individualized attention based on their preferences and past interactions.
- Technological Advancements: With the advent of technology, businesses began to collect data on customer behavior. This data allowed for more targeted marketing and service offerings. For instance, companies started using customer relationship management (CRM) systems to track interactions and preferences, similar to the approach taken by stitch fix customer service.
- Data-Driven Personalization: The rise of big data analytics has further enhanced service personalization. Businesses can now analyze vast amounts of data to understand customer needs and preferences better. This has led to the development of personalized marketing strategies, where recommendations are tailored based on individual behavior, as seen in aws personalization.
- Omnichannel Experiences: Today, service personalization extends across multiple channels. Customers expect a seamless experience whether they are interacting online, via mobile apps, or in-store. Companies are integrating their services to provide a consistent and personalized experience across all platforms, including personalized assistance and customer greeting.
- Future Trends: The future of service personalization is likely to involve even more sophisticated technologies, such as predictive analytics and machine learning, which will enable businesses to anticipate customer needs before they even express them. At Rapid Innovation, we leverage these technologies to help our clients create personalized experiences that drive customer engagement and satisfaction, ultimately leading to greater ROI.
1.2. Role of AI Agents in Modern Services
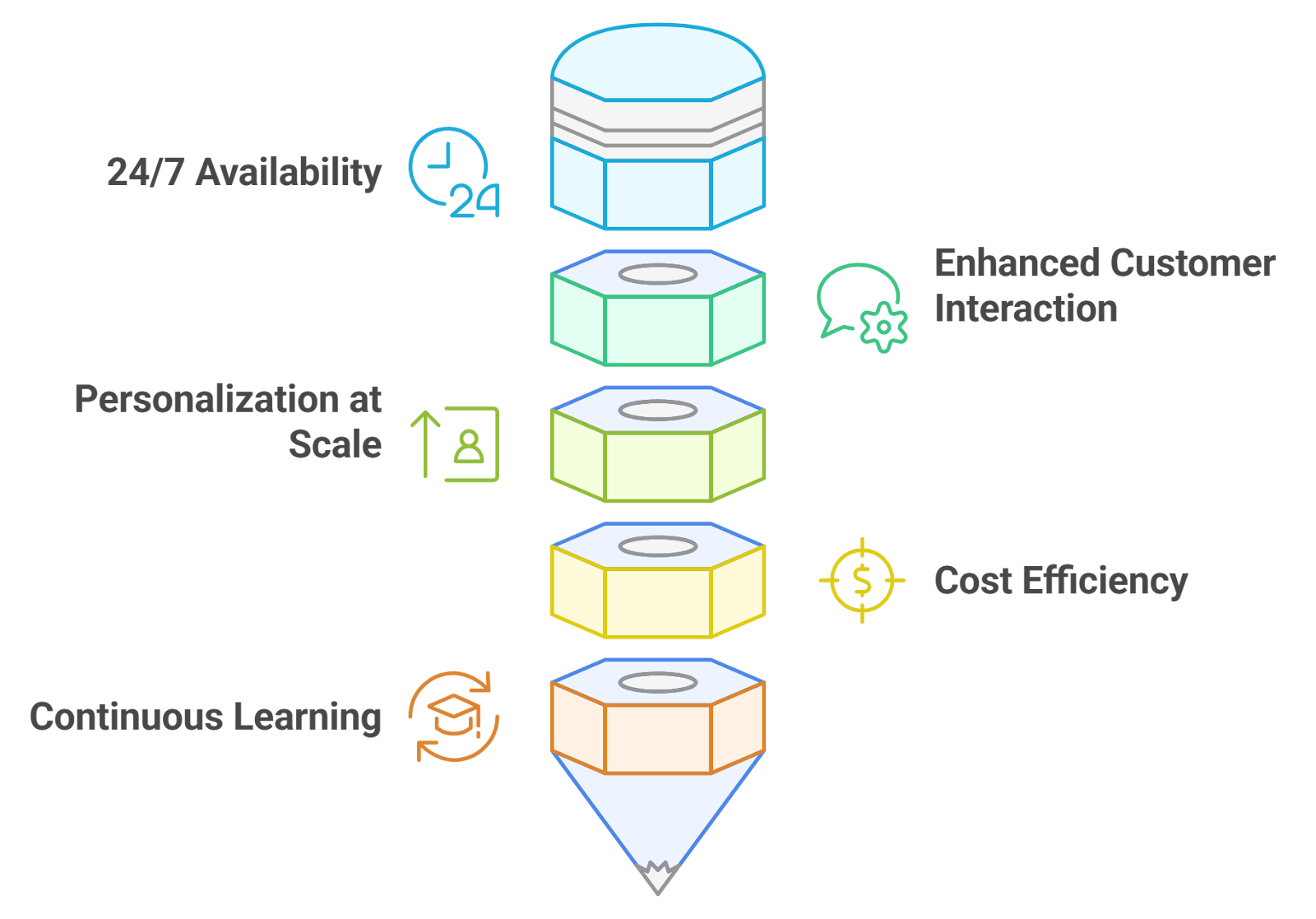
AI agents are becoming integral to modern service delivery, transforming how businesses interact with customers and manage operations.
- 24/7 Availability: AI agents, such as chatbots and virtual assistants, provide round-the-clock service. This ensures that customers can receive assistance at any time, enhancing customer satisfaction, similar to the support offered by stitch fix customer service number.
- Enhanced Customer Interaction: AI agents can analyze customer queries and provide instant responses. They can handle multiple inquiries simultaneously, reducing wait times and improving efficiency, much like the efficiency seen in consumer card services bank.
- Personalization at Scale: AI agents utilize machine learning algorithms to analyze customer data and preferences. This allows them to offer personalized recommendations and solutions, creating a more tailored experience for each user. Rapid Innovation specializes in developing AI solutions that enable businesses to scale their personalization efforts effectively, including personalized customer service and service personalization.
- Cost Efficiency: Implementing AI agents can significantly reduce operational costs. Businesses can automate routine tasks, allowing human agents to focus on more complex issues that require emotional intelligence and critical thinking, similar to the approach taken by harland clarke checks contact number.
- Continuous Learning: AI agents improve over time through machine learning. They learn from past interactions, enabling them to provide better service and adapt to changing customer needs, akin to the continuous improvement seen in customized customer service.
1.3. The Personalization Revolution
The personalization revolution is reshaping how businesses engage with customers, driven by advancements in technology and changing consumer expectations.
- Customer-Centric Approach: Modern consumers expect brands to understand their unique preferences and needs. Personalization is no longer a luxury; it is a necessity for businesses to remain competitive, as demonstrated by examples of personalised care.
- Data Utilization: Companies are leveraging data analytics to gain insights into customer behavior. This data-driven approach allows businesses to create targeted marketing campaigns and personalized service offerings. At Rapid Innovation, we assist clients in harnessing data analytics to enhance their personalization strategies, ensuring they meet customer expectations effectively, similar to the strategies employed by personalization in digital marketing.
- Enhanced Customer Loyalty: Personalized experiences foster stronger customer relationships. When customers feel valued and understood, they are more likely to remain loyal to a brand and make repeat purchases, as seen with personalized service examples.
- Cross-Industry Adoption: The personalization revolution is not limited to retail. Industries such as healthcare, finance, and entertainment are also adopting personalized strategies to enhance customer engagement and satisfaction, including the practices of ordermychecks com customer service and harland checks customer service.
- Future Implications: As technology continues to evolve, the personalization revolution will likely expand. Businesses will need to stay ahead of trends, utilizing emerging technologies like augmented reality and virtual reality to create immersive personalized experiences. Rapid Innovation is committed to guiding clients through these advancements, ensuring they leverage the latest technologies to achieve their business goals efficiently and effectively, including the use of personalized print on demand and custom local phone number solutions.
Refer to the image for a visual representation of the evolution of service personalization.

1.4. Core Components of AI-Driven Personalization
AI-driven personalization is transforming how businesses interact with customers. By leveraging advanced technologies, companies can create tailored experiences that resonate with individual preferences. The core components of AI-driven personalization include:
- Data Collection: Gathering data from various sources is crucial. This includes user behavior, preferences, demographics, and interactions across multiple channels. The more comprehensive the data, the better the AI-driven personalization.
- Data Analysis: Once data is collected, AI algorithms analyze it to identify patterns and trends. Machine learning models can process vast amounts of data quickly, enabling businesses to understand customer needs and preferences effectively.
- Segmentation: AI-driven personalization often involves segmenting users into distinct groups based on shared characteristics. This allows businesses to tailor their marketing strategies and product offerings to specific segments, enhancing relevance.
- Recommendation Systems: These systems use algorithms to suggest products or content based on user behavior and preferences. For instance, platforms like Netflix and Amazon utilize recommendation engines to enhance user engagement and drive sales.
- Real-Time Personalization: AI enables businesses to deliver personalized experiences in real-time. By analyzing user interactions as they happen, companies can adjust their offerings instantly, improving customer satisfaction.
- Feedback Loops: Continuous learning is essential for AI-driven personalization. Feedback loops allow AI systems to refine their algorithms based on user responses, ensuring that personalization efforts remain effective over time.
- Privacy and Ethics: As personalization relies heavily on data, businesses must prioritize user privacy and ethical considerations. Transparency in data usage and compliance with regulations like GDPR are vital for maintaining customer trust.
2. Understanding AI Agents for Personalization
AI agents play a pivotal role in delivering personalized experiences. These intelligent systems can interact with users, analyze data, and make decisions based on user preferences. Understanding AI agents involves recognizing their capabilities and functions in the personalization landscape.
- Definition of AI Agents: AI agents are software programs that use artificial intelligence to perform tasks autonomously. They can learn from data, adapt to new information, and interact with users in a human-like manner.
- Role in Personalization: AI agents enhance AI-driven personalization by analyzing user data and providing tailored recommendations. They can engage with customers through chatbots, virtual assistants, and other interactive platforms, creating a seamless experience.
- Benefits of AI Agents: AI agents offer several advantages, including increased efficiency in handling customer inquiries, enhanced user engagement through personalized interactions, and the ability to analyze large datasets quickly for better insights.
2.1. Types of AI Agents
AI agents come in various forms, each designed to fulfill specific roles in the personalization process. Understanding the different types of AI agents can help businesses choose the right solutions for their needs.
- Chatbots: These AI agents simulate human conversation and can assist users with inquiries, provide recommendations, and guide them through processes. Chatbots are widely used in customer service to enhance user experience.
- Virtual Assistants: More advanced than chatbots, virtual assistants like Siri and Alexa can perform a range of tasks, from setting reminders to controlling smart home devices. They learn from user interactions to provide increasingly personalized responses.
- Recommendation Engines: These AI agents analyze user behavior and preferences to suggest products or content. They are commonly used in e-commerce and streaming services to enhance user engagement and drive sales.
- Predictive Analytics Agents: These agents use historical data to forecast future trends and behaviors. By understanding potential customer actions, businesses can tailor their marketing strategies and improve customer retention.
- Personalization Engines: These AI systems focus specifically on delivering personalized content and experiences. They analyze user data to create customized marketing messages, product recommendations, and user interfaces.
- Social Media Bots: These agents interact with users on social media platforms, providing personalized content and responding to inquiries. They help brands maintain engagement and foster community interaction.
- Voice Recognition Agents: These AI agents use natural language processing to understand and respond to voice commands. They enhance AI-driven personalization by allowing users to interact with technology in a more intuitive manner.
By understanding the core components of AI-driven personalization and the various types of AI agents, businesses can effectively leverage these technologies to create more engaging and tailored experiences for their customers. At Rapid Innovation, we specialize in implementing these AI-driven solutions, ensuring that our clients achieve greater ROI through enhanced customer engagement and satisfaction. For more information on how we can assist you, visit our AI agents for user experience personalization and our AI agent development company.
Refer to the image for a visual representation of the core components of AI-driven personalization.
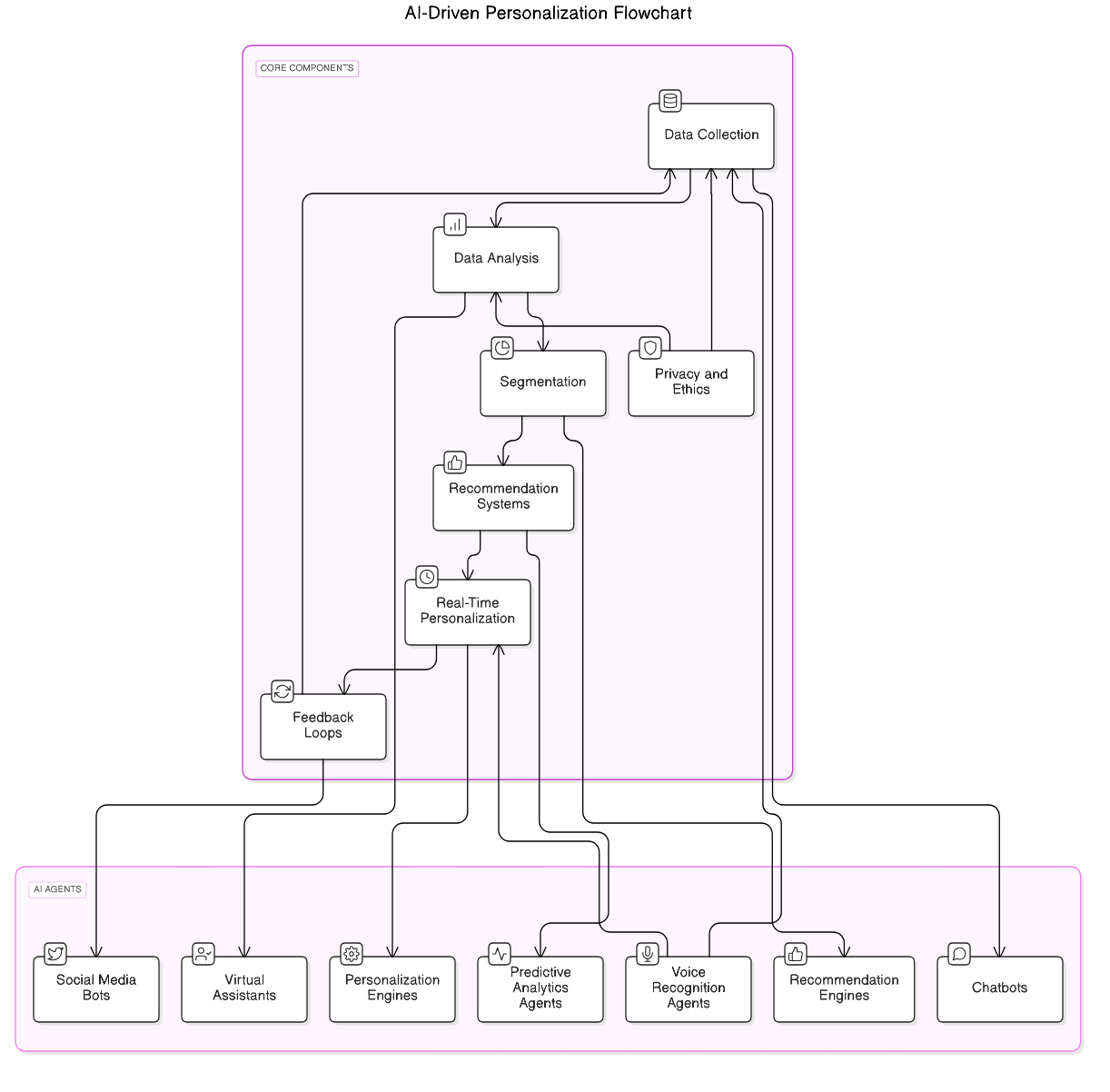
2.1.1. Recommendation Agents
Recommendation agents are sophisticated algorithms designed to analyze user behavior and preferences to suggest products, services, or content. They play a crucial role in enhancing user experience and driving engagement across various platforms. These agents tailor recommendations based on individual user data, such as past purchases, browsing history, and demographic information. They utilize machine learning techniques to process vast amounts of data, identifying patterns and trends that inform their suggestions. By providing relevant recommendations, such as a recommendation for real estate agent or realtor recommendation, these agents can significantly boost user interaction and satisfaction, leading to higher conversion rates. Popular platforms like Netflix and Amazon employ recommendation agents to suggest movies and products, respectively, based on user preferences.
- Personalization: These agents tailor recommendations based on individual user data, such as past purchases, browsing history, and demographic information.
- Data Analysis: They utilize machine learning techniques to process vast amounts of data, identifying patterns and trends that inform their suggestions.
- Increased Engagement: By providing relevant recommendations, such as recommendation agents or recommendation for a realtor, these agents can significantly boost user interaction and satisfaction, leading to higher conversion rates.
- Examples: Popular platforms like Netflix and Amazon employ recommendation agents to suggest movies and products, respectively, based on user preferences.
At Rapid Innovation, we leverage our expertise in AI to develop customized recommendation systems that align with your business objectives. By implementing these systems, clients have seen a marked increase in user engagement and conversion rates, ultimately leading to a greater return on investment (ROI). For more insights, check out our article on understanding proactive AI agents.
2.1.2. Conversational Agents
Conversational agents, often referred to as chatbots or virtual assistants, are designed to simulate human-like interactions through natural language processing (NLP). They can engage users in dialogue, answer questions, and perform tasks, making them invaluable in customer service and support. These agents can handle multiple queries simultaneously, providing instant responses to users, which enhances customer satisfaction. Unlike human agents, conversational agents are available around the clock, ensuring that users can receive assistance at any time. Implementing conversational agents can reduce operational costs for businesses by minimizing the need for extensive customer service teams. Siri, Google Assistant, and customer service chatbots on websites are all forms of conversational agents that facilitate user interaction.
- User Interaction: These agents can handle multiple queries simultaneously, providing instant responses to users, which enhances customer satisfaction.
- 24/7 Availability: Unlike human agents, conversational agents are available around the clock, ensuring that users can receive assistance at any time.
- Cost Efficiency: Implementing conversational agents can reduce operational costs for businesses by minimizing the need for extensive customer service teams.
- Examples: Siri, Google Assistant, and customer service chatbots on websites are all forms of conversational agents that facilitate user interaction.
At Rapid Innovation, we specialize in creating tailored conversational agents that enhance customer engagement and streamline support processes. Our clients have reported significant reductions in operational costs while improving customer satisfaction, demonstrating a clear path to increased ROI.
2.1.3. Predictive Service Agents
Predictive service agents leverage data analytics and machine learning to forecast user needs and behaviors. They proactively offer services or solutions based on anticipated requirements, enhancing user experience and operational efficiency. By analyzing historical data, these agents can predict what users might need next, allowing businesses to offer timely solutions. Predictive analytics helps organizations make informed decisions by understanding trends and user behavior patterns. By anticipating user needs, predictive service agents can provide personalized experiences, leading to increased loyalty and satisfaction. Predictive service agents are commonly used in industries like e-commerce, where they can suggest products before users even search for them, or in healthcare, where they can predict patient needs based on medical history.
- Anticipation of Needs: By analyzing historical data, these agents can predict what users might need next, allowing businesses to offer timely solutions.
- Improved Decision-Making: Predictive analytics helps organizations make informed decisions by understanding trends and user behavior patterns.
- Enhanced Customer Experience: By anticipating user needs, predictive service agents can provide personalized experiences, leading to increased loyalty and satisfaction.
- Examples: Predictive service agents are commonly used in industries like e-commerce, where they can suggest products before users even search for them, or in healthcare, where they can predict patient needs based on medical history.
At Rapid Innovation, we harness the power of predictive analytics to develop agents that not only enhance user experience but also drive strategic decision-making. Our clients have experienced improved customer loyalty and satisfaction, translating into a higher ROI through proactive engagement and service delivery.
Refer to the image for a visual representation of Recommendation Agents and their functionalities:
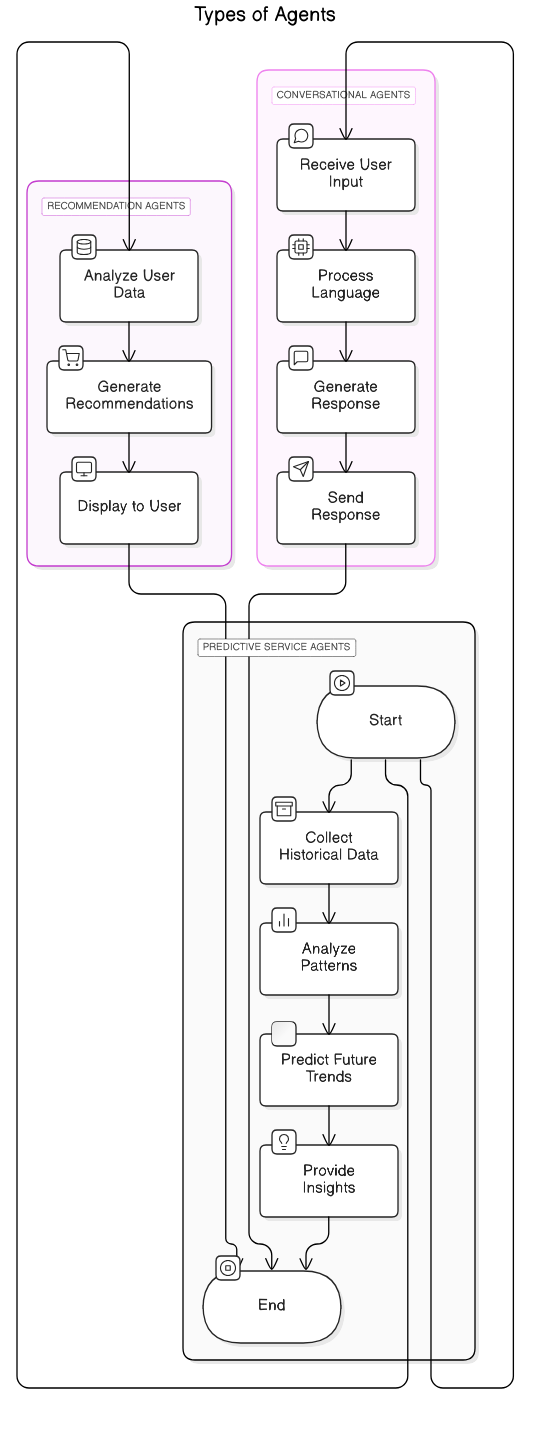
2.1.4. Behavioral Analysis Agents
Behavioral analysis agents are specialized software programs designed to monitor, analyze, and interpret user behavior within various environments, such as websites, applications, or networks. These agents utilize advanced algorithms and machine learning techniques to identify patterns and anomalies in user actions. They can detect unusual behavior that may indicate security threats, such as unauthorized access or data breaches. Additionally, behavioral analysis agents often employ techniques like user profiling, which helps in creating a baseline of normal behavior for each user. By continuously learning from user interactions, these agents can adapt to changes in behavior over time, improving their accuracy in threat detection. They are widely used in cybersecurity, fraud detection, and personalized marketing strategies. The insights generated by these agents can help organizations make informed decisions regarding user engagement and security measures, ultimately leading to enhanced operational efficiency and greater ROI. This is similar to the work done by fbi agent behavioral analysis unit and fbi special agent behavioral analysis unit, which focus on understanding and predicting behavior in various contexts. For more information on the key components of modern AI agent architecture.
2.2. Agent Architecture and Components
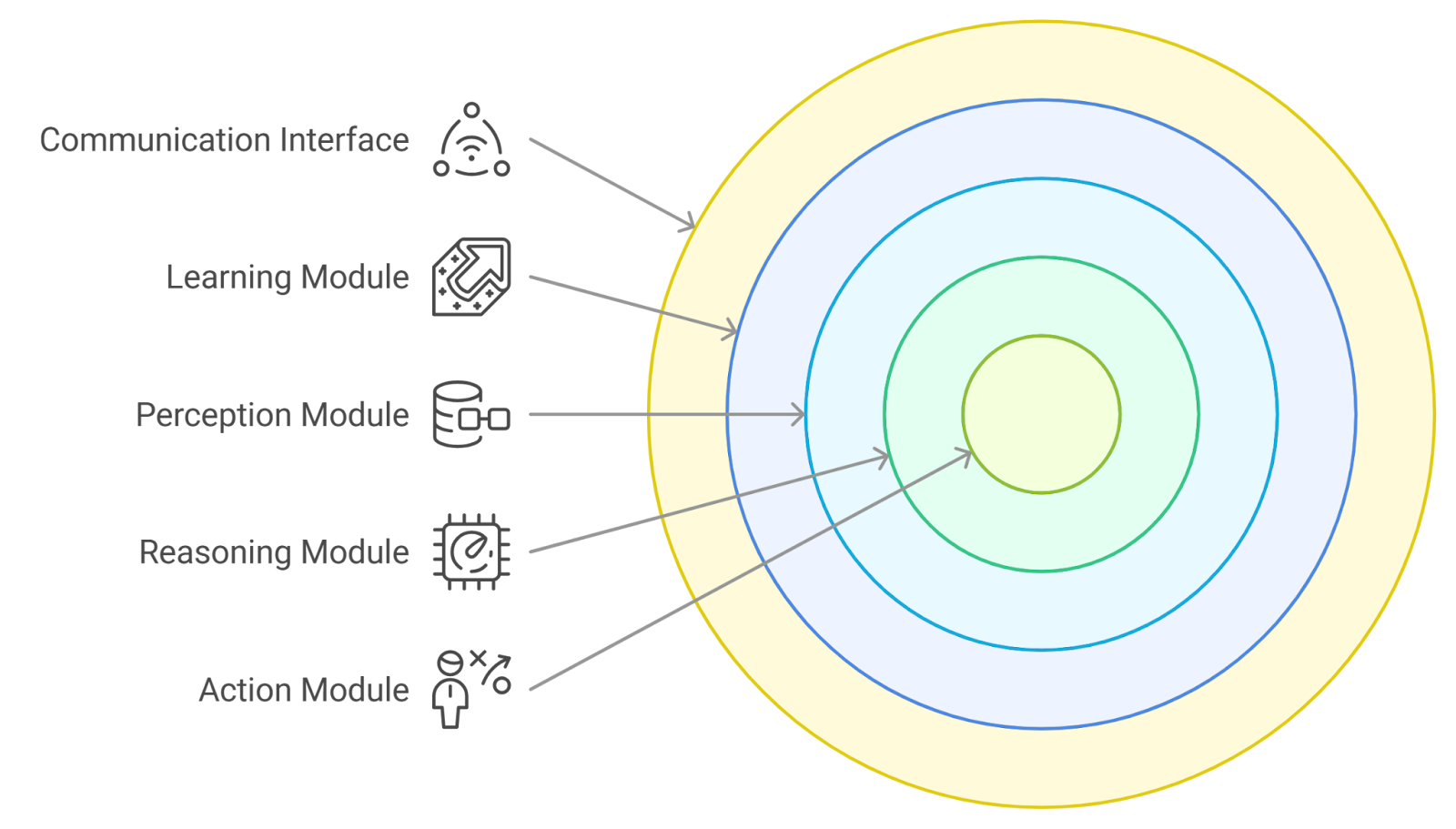
The architecture of an agent system is crucial for its functionality and efficiency. It typically consists of several key components that work together to enable the agent to perform its tasks effectively.
- Perception Module: This component gathers data from the environment, which can include user inputs, system logs, and external data sources.
- Reasoning Module: This module processes the data collected by the perception module, applying algorithms to analyze and interpret the information.
- Action Module: Based on the reasoning, this component determines the appropriate actions the agent should take, whether it be alerting users, blocking access, or providing recommendations.
- Learning Module: This component allows the agent to improve its performance over time by learning from past experiences and outcomes.
- Communication Interface: This enables the agent to interact with other systems or agents, facilitating collaboration and data sharing.
The integration of these components ensures that the agent can operate autonomously while still being able to adapt to new challenges and environments, much like the fbi bau supervisory special agent who must adapt to various investigative scenarios.
2.3. Data Processing Capabilities
Data processing capabilities are essential for agents to function effectively in analyzing and interpreting large volumes of information. These capabilities determine how well an agent can handle data and derive meaningful insights.
- Real-time Processing: Many agents are designed to process data in real-time, allowing for immediate responses to user actions or security threats.
- Batch Processing: Some agents can handle large datasets in batches, which is useful for historical analysis and trend identification, similar to the methods used by behavioral analysis agents in the fbi.
- Data Integration: Agents often have the ability to integrate data from multiple sources, providing a comprehensive view of user behavior and system performance.
- Scalability: Effective agents can scale their processing capabilities to accommodate increasing data loads without compromising performance.
- Data Visualization: Many agents include features for visualizing data, making it easier for users to understand complex information and trends.
These data processing capabilities are vital for ensuring that behavioral analysis agents can deliver accurate and timely insights, ultimately enhancing decision-making and security measures. At Rapid Innovation, we leverage these advanced capabilities to help our clients achieve their business goals efficiently and effectively, driving greater ROI through enhanced security and user engagement strategies, much like the strategies employed by fbi agent bau in their operations.
2.4. Learning Mechanisms
Learning mechanisms are essential components in the development of intelligent systems, particularly in the context of machine learning and artificial intelligence. These mechanisms enable systems to adapt and improve over time based on the data they encounter.
- Types of Learning Mechanisms:
- Supervised Learning: Involves training a model on a labeled dataset, where the input data is paired with the correct output. This method is widely used for classification and regression tasks, allowing businesses to make data-driven decisions that enhance operational efficiency.
- Unsupervised Learning: This mechanism deals with unlabeled data, allowing the model to identify patterns and relationships without explicit guidance. Clustering and dimensionality reduction are common techniques that can uncover hidden insights, driving innovation and strategic planning.
- Reinforcement Learning: Here, an agent learns to make decisions by taking actions in an environment to maximize cumulative rewards. This approach is often used in robotics and game playing, but it can also be applied to optimize processes in various industries, leading to significant cost savings.
- Importance of Learning Mechanisms:
- They enable systems to improve accuracy over time, which is crucial for maintaining a competitive edge.
- They facilitate the discovery of hidden patterns in data, allowing businesses to identify new opportunities and mitigate risks.
- They support adaptive learning, allowing systems to respond to new information dynamically, ensuring that organizations remain agile in a rapidly changing market.
- Applications:
- Personalized recommendations in e-commerce, enhancing customer experience and increasing sales.
- Fraud detection in financial services, protecting assets and reducing losses.
- Predictive maintenance in manufacturing, minimizing downtime and optimizing resource allocation.
Understanding these learning mechanisms is crucial for developing effective AI systems that can provide personalized experiences and insights, ultimately leading to greater ROI for businesses. For more information on how AI agents can be utilized in marketing applications.
3. Data Foundation for Personalization
The data foundation for personalization is critical in creating tailored experiences for users. Personalization relies heavily on data to understand user preferences, behaviors, and needs.
- Key Components:
- User Data: Information collected from user interactions, such as browsing history, purchase history, and feedback, which can be leveraged to enhance customer engagement.
- Contextual Data: Data that provides context about the user’s environment, such as location, time of day, and device type, enabling businesses to deliver timely and relevant content.
- Behavioral Data: Insights into how users engage with content, including click patterns, time spent on pages, and social media interactions, which can inform marketing strategies and product development.
- Benefits of a Strong Data Foundation:
- Enhances user engagement by delivering relevant content, leading to increased customer loyalty.
- Increases conversion rates through targeted marketing strategies, maximizing the effectiveness of advertising spend.
- Improves customer satisfaction by anticipating user needs, fostering long-term relationships.
- Challenges:
- Ensuring data privacy and compliance with regulations like GDPR, which is essential for maintaining trust and avoiding legal repercussions.
- Managing data quality and accuracy to avoid misleading insights, which can lead to poor decision-making.
- Integrating data from multiple sources for a comprehensive view, enabling a holistic understanding of customer behavior.
A robust data foundation is essential for effective personalization, enabling businesses to create meaningful connections with their customers.
3.1. Data Collection Strategies
Data collection strategies are vital for gathering the information necessary to drive personalization efforts. Effective strategies ensure that the right data is collected in a manner that respects user privacy and enhances the overall user experience.
- Methods of Data Collection:
- Surveys and Questionnaires: Directly asking users for their preferences and feedback can yield valuable insights that inform product development and marketing strategies.
- Web Analytics: Tools like Google Analytics track user behavior on websites, providing data on page views, session duration, and user demographics, which can be used to optimize user journeys.
- Social Media Monitoring: Analyzing user interactions on social media platforms can reveal trends and preferences, allowing businesses to engage with their audience more effectively.
- Best Practices:
- Transparency: Clearly communicate to users how their data will be used and obtain their consent, fostering trust and compliance.
- Data Minimization: Collect only the data necessary for the intended purpose to reduce privacy risks and enhance user confidence.
- Regular Updates: Continuously update data collection methods to adapt to changing user behaviors and preferences, ensuring relevance and accuracy.
- Technological Tools:
- Customer Relationship Management (CRM) Systems: These systems help manage user data and interactions, providing a centralized database for personalization efforts that can drive sales and improve customer service.
- Data Management Platforms (DMPs): DMPs aggregate data from various sources, allowing for more comprehensive user profiles that can enhance targeting and segmentation.
- Machine Learning Algorithms: These can analyze collected data to identify patterns and predict user behavior, enabling proactive engagement strategies.
Implementing effective data collection strategies is crucial for building a strong foundation for personalization, ultimately leading to improved user experiences and business outcomes. Rapid Innovation is committed to helping clients harness these strategies to achieve their business goals efficiently and effectively.
3.1.1. Explicit Data Gathering
Explicit data gathering refers to the process of collecting information directly from users through various methods, including computer assisted personal interviewing (CAPI). This type of data is often characterized by its clarity and specificity, as it is obtained through intentional actions by the user.
- Surveys and Questionnaires: Organizations often use surveys to gather explicit data. These tools allow users to provide feedback on products, services, or experiences. Rapid Innovation can assist clients in designing effective surveys that yield actionable insights, ultimately enhancing product offerings and customer satisfaction. Different data collection techniques, such as online surveys and face-to-face interviews, can be employed to maximize response rates.
- Interviews: Conducting one-on-one interviews, including CAPI computer assisted personal interviewing, can yield in-depth insights into user preferences and behaviors. Our team can facilitate these interviews, ensuring that the right questions are asked to uncover valuable information that drives business decisions.
- Registration Forms: When users sign up for services, they typically provide personal information, which can be used for targeted marketing and personalization. Rapid Innovation can help clients implement secure and efficient registration processes that maximize data collection while ensuring user privacy.
- User Feedback: Direct feedback mechanisms, such as comment sections or rating systems, enable users to express their opinions explicitly. We can develop tailored feedback systems that integrate seamlessly into existing platforms, allowing businesses to gather and analyze user sentiments effectively.
Explicit data is valuable because it is often more reliable and easier to analyze. However, it requires careful design to ensure that the questions asked yield useful information, particularly in qualitative data collection methods.
3.1.2. Implicit Data Collection
Implicit data collection involves gathering information about users without their direct input. This method relies on observing user behavior and interactions, often through automated systems.
- Browsing Behavior: Tracking how users navigate a website can reveal their interests and preferences. Rapid Innovation employs advanced analytics tools to help clients understand user journeys, enabling them to optimize website design and content.
- Purchase History: Analyzing past purchases helps businesses understand consumer habits and predict future buying behavior. Our data scientists can create predictive models that enhance inventory management and marketing strategies, leading to increased sales.
- Social Media Activity: Monitoring user interactions on social media platforms can provide insights into their interests and social circles. We can assist clients in leveraging social media analytics to refine their branding and engagement strategies.
- Device Usage: Collecting data on how users interact with applications or devices can inform product development and marketing strategies. Rapid Innovation can implement tracking solutions that provide insights into user engagement across multiple platforms.
Implicit data collection is powerful because it captures real-time behavior, often leading to more accurate insights. However, it raises privacy concerns, and organizations must ensure they comply with data protection regulations.
3.1.3. Contextual Data Analysis
Contextual data analysis involves examining data within the context in which it was collected. This approach helps organizations understand the circumstances surrounding user behavior, leading to more meaningful insights.
- Environmental Factors: Analyzing data in relation to external factors, such as time of day or location, can reveal patterns in user behavior. Rapid Innovation can help clients incorporate contextual data into their analytics frameworks, enhancing decision-making processes.
- User Intent: Understanding the motivations behind user actions can enhance the interpretation of data. For example, a user browsing a product page may have different intentions than one who adds an item to their cart. Our expertise in AI can help clients develop models that accurately predict user intent, allowing for more personalized marketing efforts.
- Historical Context: Comparing current data with historical trends can help identify shifts in user behavior over time. We can assist clients in building robust data repositories that facilitate longitudinal studies and trend analysis, utilizing various data collection methodologies.
- Cross-Channel Analysis: Examining data from multiple sources (e.g., website, social media, email) provides a holistic view of user interactions. Rapid Innovation can integrate various data streams, enabling clients to gain comprehensive insights into customer journeys.
Contextual data analysis is crucial for making informed decisions. It allows businesses to tailor their strategies based on a comprehensive understanding of user behavior and preferences. By leveraging our expertise in AI and Blockchain, Rapid Innovation empowers clients to achieve greater ROI through data-driven decision-making, utilizing effective data gathering tools and techniques.
3.2. Data Quality and Preprocessing
Data quality is a critical aspect of any data-driven project. High-quality data ensures accurate analysis and reliable insights. Poor data quality can lead to misleading conclusions and ineffective decision-making.
- Importance of Data Quality:
- Accurate data leads to better business decisions.
- High-quality data enhances the performance of machine learning models.
- Poor data quality can result in increased costs and wasted resources.
- Common Data Quality Issues:
- Incomplete data: Missing values can skew results.
- Inconsistent data: Variations in data formats can lead to confusion.
- Duplicate data: Redundant entries can inflate datasets and mislead analyses.
- Preprocessing Techniques:
- Data cleaning: Removing inaccuracies and correcting errors.
- Data transformation: Normalizing data to ensure consistency.
- Data integration: Combining data from different sources for a unified view.
- Tools for Data Quality and Preprocessing:
- Open-source tools like Pandas and NumPy for data manipulation.
- Data quality assessment tools such as Talend and Informatica.
- Automated data cleaning solutions that leverage AI.
At Rapid Innovation, we understand that investing time in data quality and preprocessing can significantly enhance the effectiveness of data analytics and machine learning initiatives. Our expertise in AI-driven data solutions ensures that your data is not only clean but also primed for actionable insights, ultimately leading to greater ROI. Learn about the critical role of data quality in AI implementations.
3.3. Real-time Data Processing
Real-time data processing refers to the immediate processing of data as it is generated. This capability is essential for businesses that require timely insights to make quick decisions.
- Benefits of Real-time Data Processing:
- Instant insights: Businesses can react promptly to changing conditions.
- Enhanced customer experience: Real-time data allows for personalized interactions.
- Improved operational efficiency: Organizations can optimize processes on-the-fly.
- Technologies for Real-time Data Processing:
- Stream processing frameworks like Apache Kafka and Apache Flink.
- Event-driven architectures that facilitate real-time data flow.
- Cloud services such as AWS Kinesis and Google Cloud Dataflow for scalable solutions.
- Use Cases:
- Fraud detection in financial transactions.
- Monitoring social media sentiment in real-time.
- Real-time inventory management in retail.
Real-time data processing is becoming increasingly vital in various industries, enabling organizations to stay competitive and responsive to market demands. Rapid Innovation leverages cutting-edge technologies to implement real-time data solutions that empower businesses to make informed decisions swiftly, enhancing overall operational efficiency and customer satisfaction.
3.4. Privacy and Compliance Considerations
As data collection and processing become more prevalent, privacy and compliance have emerged as significant concerns. Organizations must navigate various regulations to protect user data and maintain trust.
- Key Regulations:
- General Data Protection Regulation (GDPR): Enforces strict data protection and privacy for individuals in the EU.
- California Consumer Privacy Act (CCPA): Grants California residents rights regarding their personal information.
- Health Insurance Portability and Accountability Act (HIPAA): Protects sensitive patient health information in the U.S.
- Best Practices for Compliance:
- Data minimization: Collect only the data necessary for specific purposes.
- Anonymization: Remove personally identifiable information (PII) to protect user privacy.
- Regular audits: Conduct compliance audits to ensure adherence to regulations.
- Challenges:
- Keeping up with evolving regulations can be complex.
- Balancing data utility with privacy concerns is often challenging.
- Ensuring third-party vendors comply with privacy standards.
Organizations must prioritize privacy and compliance to build trust with customers and avoid potential legal repercussions. Implementing robust data governance frameworks can help manage these challenges effectively. At Rapid Innovation, we provide consulting services to help organizations navigate these complexities, ensuring that your data practices align with regulatory requirements while maximizing the value derived from your data assets.
4. Core Technologies and Algorithms
The landscape of technology is rapidly evolving, and at the heart of this evolution are core technologies and algorithms that drive innovation. Understanding these technologies is crucial for anyone looking to leverage them effectively in various applications, from artificial intelligence to data analytics.
4.1. Machine Learning Models
Machine learning (ML) is a subset of artificial intelligence that enables systems to learn from data and improve their performance over time without being explicitly programmed. The core of machine learning lies in its models, which can be broadly categorized into different types based on how they learn from data.
Machine learning models can be classified into: - Supervised Learning - Unsupervised Learning - Reinforcement Learning
Each of these categories has its own set of algorithms and applications, but supervised learning is one of the most widely used and understood.
4.1.1. Supervised Learning Approaches
Supervised learning is a type of machine learning where the model is trained on a labeled dataset. This means that the input data is paired with the correct output, allowing the model to learn the relationship between the two. The goal is to make predictions or classifications based on new, unseen data.
Key characteristics of supervised learning include: - Requires labeled data for training - Involves a clear objective, such as classification or regression - The model's performance can be evaluated using metrics like accuracy, precision, and recall
Common algorithms used in supervised learning include: - Linear Regression: Used for predicting continuous values, often implemented with scikit learn linear regression. - Logistic Regression: Used for binary classification problems. - Decision Trees: A flowchart-like structure that makes decisions based on feature values, also available through decision tree scikit learn. - Support Vector Machines (SVM): Effective for high-dimensional spaces and classification tasks. - Neural Networks: Particularly useful for complex patterns and large datasets, as seen in neural network machine learning.
Applications of supervised learning include: - Image recognition: Classifying images into categories. - Spam detection: Identifying whether an email is spam or not. - Medical diagnosis: Predicting diseases based on patient data. - Financial forecasting: Predicting stock prices or market trends.
At Rapid Innovation, we harness the power of supervised learning to help our clients achieve greater ROI. For instance, in the healthcare sector, we have developed predictive models that assist in early disease detection, significantly improving patient outcomes and reducing costs. Similarly, our financial forecasting models have enabled clients to make informed investment decisions, optimizing their portfolios and maximizing returns.
Supervised learning has proven to be effective in various domains, but it also comes with challenges. The need for large amounts of labeled data can be a significant barrier, and the model's performance is heavily dependent on the quality of the data used for training.
In conclusion, supervised learning approaches are foundational to many machine learning applications, providing a structured way to train models and make predictions. Understanding these core technologies and algorithms, such as convolutional neural networks, random forest scikit learn, and restricted boltzmann machine, is essential for harnessing the power of machine learning in real-world scenarios, and at Rapid Innovation, we are committed to guiding our clients through this transformative journey. For more information on how we can assist with adaptive AI development, visit our Adaptive AI Development page. For a comprehensive overview of machine learning, check out our Machine Learning: The Complete Guide.
4.1.2. Unsupervised Learning Methods
Unsupervised learning is a type of machine learning where the model is trained on data without labeled responses. This method is particularly useful for discovering hidden patterns or intrinsic structures in data. Unlike supervised learning, where the model learns from labeled data, unsupervised learning relies on the inherent characteristics of the data itself.
- Clustering: This technique groups similar data points together. Common algorithms include K-means, hierarchical clustering, and DBSCAN. Clustering is widely used in market segmentation, social network analysis, and image compression. At Rapid Innovation, we leverage clustering to help businesses identify distinct customer segments, enabling targeted marketing strategies that enhance customer engagement and drive sales. Unsupervised learning clustering is a key aspect of this process, allowing for effective unsupervised classification.
- Dimensionality Reduction: This method reduces the number of features in a dataset while preserving its essential characteristics. Techniques like Principal Component Analysis (PCA) and t-Distributed Stochastic Neighbor Embedding (t-SNE) are popular for visualizing high-dimensional data and improving model performance. By applying dimensionality reduction, we assist clients in simplifying complex datasets, leading to more efficient data processing and analysis. Dimensionality reduction unsupervised learning techniques are crucial for handling large datasets.
- Anomaly Detection: Unsupervised learning can identify unusual data points that deviate from the norm. This is crucial in fraud detection, network security, and fault detection in manufacturing processes. Rapid Innovation employs anomaly detection techniques to help organizations safeguard their operations, minimizing risks and potential losses. Anomaly detection using unsupervised learning is an effective strategy for identifying outliers.
- Association Rule Learning: This method uncovers interesting relationships between variables in large datasets. The Apriori algorithm and FP-Growth are commonly used for market basket analysis, helping retailers understand customer purchasing behavior. Our expertise in association rule learning allows clients to optimize inventory management and enhance cross-selling opportunities. This is part of the broader scope of data mining unsupervised learning.
Unsupervised learning is essential in various fields, including finance, healthcare, and marketing, as it helps organizations make data-driven decisions without the need for extensive labeled datasets. Techniques such as unsupervised machine learning models and unsupervised learning techniques are vital for extracting insights from unstructured data.
4.1.3. Reinforcement Learning Applications
Reinforcement learning (RL) is a type of machine learning where an agent learns to make decisions by taking actions in an environment to maximize cumulative rewards. This approach is inspired by behavioral psychology and is particularly effective in dynamic and complex environments.
- Robotics: RL is widely used in robotics for training autonomous agents to perform tasks such as navigation, manipulation, and interaction with humans. Robots can learn from trial and error, improving their performance over time. Rapid Innovation harnesses RL to develop intelligent robotic systems that enhance operational efficiency in various industries.
- Game Playing: RL has gained significant attention in the gaming industry. Notable examples include AlphaGo, which defeated a world champion in the game of Go, and OpenAI's Dota 2 bot. These applications demonstrate RL's ability to learn complex strategies and adapt to opponents. Our team at Rapid Innovation applies similar principles to create engaging and adaptive gaming experiences.
- Autonomous Vehicles: Reinforcement learning plays a crucial role in the development of self-driving cars. By simulating various driving scenarios, RL algorithms can optimize decision-making processes, such as lane changing, obstacle avoidance, and route planning. We collaborate with automotive companies to integrate RL solutions that enhance vehicle safety and navigation.
- Healthcare: In personalized medicine, RL can optimize treatment plans by learning from patient responses to different therapies. This approach can lead to more effective and tailored healthcare solutions. Rapid Innovation partners with healthcare providers to implement RL strategies that improve patient outcomes and operational efficiency.
- Finance: RL is applied in algorithmic trading, where agents learn to make buy or sell decisions based on market conditions. This can enhance investment strategies and improve portfolio management. Our expertise in RL enables clients to develop sophisticated trading algorithms that maximize returns.
Reinforcement learning continues to evolve, with ongoing research exploring its applications in various domains, including education, energy management, and supply chain optimization.
4.2. Natural Language Processing

Natural Language Processing (NLP) is a subfield of artificial intelligence that focuses on the interaction between computers and human language. NLP enables machines to understand, interpret, and generate human language in a valuable way. The applications of NLP are vast and impactful across numerous industries.
- Text Analysis: NLP techniques are used to analyze and extract meaningful information from text data. This includes sentiment analysis, topic modeling, and keyword extraction, which help businesses understand customer opinions and trends. Rapid Innovation utilizes NLP to provide clients with insights that inform strategic decision-making.
- Machine Translation: NLP powers translation services like Google Translate, enabling real-time translation of text between different languages. This technology relies on deep learning models to improve accuracy and fluency. We assist organizations in breaking language barriers, facilitating global communication and collaboration.
- Chatbots and Virtual Assistants: NLP is integral to the development of chatbots and virtual assistants like Siri and Alexa. These systems use NLP to understand user queries and provide relevant responses, enhancing customer service and user experience. Rapid Innovation designs and implements intelligent chatbots that improve customer engagement and operational efficiency.
- Information Retrieval: Search engines utilize NLP to improve the relevance of search results. By understanding user intent and context, NLP algorithms can deliver more accurate and personalized content. Our NLP solutions help clients enhance their search capabilities, leading to better user experiences.
- Speech Recognition: NLP enables voice-activated systems to convert spoken language into text. This technology is widely used in applications such as transcription services, voice commands, and accessibility tools. We develop advanced speech recognition systems that cater to diverse user needs.
- Text Generation: Advanced NLP models, such as GPT-3, can generate human-like text based on prompts. This capability is used in content creation, summarization, and even creative writing. Rapid Innovation leverages text generation technologies to assist clients in automating content production and enhancing communication.
NLP continues to advance, driven by developments in machine learning and deep learning, making it an essential tool for businesses looking to leverage data and enhance communication.
4.3. Computer Vision Integration
Computer vision integration is a crucial aspect of modern technology, enabling machines to interpret and understand visual information from the world. This integration is increasingly being utilized across various industries, enhancing functionality and user experience.
- Applications in Various Fields:
- Healthcare: Computer vision assists in diagnosing diseases through image analysis, such as detecting tumors in radiology images. Rapid Innovation has successfully implemented computer vision solutions that improve diagnostic accuracy, leading to better patient outcomes and reduced healthcare costs.
- Retail: It helps in inventory management and customer behavior analysis by monitoring foot traffic and product interactions. Our tailored computer vision systems enable retailers to optimize stock levels and enhance customer engagement, driving sales growth. Computer vision in retail applications has proven to be a game changer.
- Automotive: Self-driving cars rely heavily on computer vision to navigate and recognize obstacles. Rapid Innovation's expertise in this area has contributed to advancements in autonomous vehicle technology, improving safety and efficiency. Computer vision in autonomous vehicles is a key area of focus.
- Technologies Involved:
- Deep Learning: Neural networks, particularly convolutional neural networks (CNNs), are pivotal in processing and analyzing visual data. Our team leverages deep learning techniques to develop robust computer vision applications that deliver high performance. Deep learning for computer vision is essential for achieving high accuracy.
- Image Recognition: Algorithms can identify and classify objects within images, enabling applications like facial recognition and security surveillance. Rapid Innovation's solutions ensure accurate and reliable image recognition, enhancing security measures for businesses. Computer vision algorithms play a significant role in this process.
- Challenges:
- Data Privacy: The use of computer vision raises concerns about surveillance and data collection without consent. We prioritize ethical practices and compliance with data protection regulations in our computer vision projects. Computer vision and data privacy are critical considerations.
- Accuracy: Ensuring high accuracy in image recognition can be challenging, especially in varied lighting and environmental conditions. Our continuous improvement processes and advanced algorithms help mitigate these challenges, ensuring optimal performance. Computer vision and machine learning are often combined to enhance accuracy.
4.4. Sentiment Analysis
Sentiment analysis is a technique used to determine the emotional tone behind a series of words, helping businesses understand customer opinions and feedback. This process is vital for enhancing customer engagement and improving products or services.
- Importance in Business:
- Customer Feedback: Companies can analyze reviews and social media comments to gauge public sentiment about their brand. Rapid Innovation's sentiment analysis tools provide actionable insights that help businesses refine their strategies and improve customer satisfaction.
- Market Research: Understanding consumer emotions can guide marketing strategies and product development. Our solutions enable clients to stay ahead of market trends by accurately interpreting consumer sentiment.
- Techniques Used:
- Natural Language Processing (NLP): NLP algorithms analyze text data to identify sentiment, categorizing it as positive, negative, or neutral. We utilize state-of-the-art NLP techniques to enhance the accuracy of sentiment analysis.
- Machine Learning: Supervised learning models are trained on labeled datasets to improve sentiment classification accuracy. Our machine learning expertise ensures that our clients benefit from the latest advancements in sentiment analysis technology.
- Applications:
- Social Media Monitoring: Brands can track sentiment trends over time to adjust their marketing strategies. Rapid Innovation's tools empower businesses to respond proactively to customer feedback and enhance their online presence.
- Customer Support: Automated systems can prioritize customer inquiries based on the sentiment expressed in messages. Our sentiment analysis solutions streamline customer support processes, improving response times and customer satisfaction.
4.5. Behavioral Pattern Recognition
Behavioral pattern recognition involves identifying and analyzing patterns in human behavior, often through data collected from various sources. This technology is essential for understanding user interactions and improving user experience.
- Applications Across Industries:
- Security: Behavioral biometrics can enhance security systems by recognizing patterns in user behavior, such as typing speed and mouse movements. Rapid Innovation's solutions provide advanced security measures that adapt to user behavior, reducing fraud and unauthorized access.
- Marketing: Businesses can analyze consumer behavior patterns to tailor marketing campaigns and improve customer targeting. Our behavioral pattern recognition tools enable clients to create personalized marketing strategies that resonate with their audience.
- Techniques and Technologies:
- Data Mining: Techniques such as clustering and classification help in identifying patterns from large datasets. Our data mining capabilities allow clients to extract valuable insights from their data, driving informed decision-making.
- Machine Learning: Algorithms learn from historical data to predict future behaviors, enabling proactive decision-making. Rapid Innovation employs machine learning to enhance the accuracy of behavioral predictions, helping businesses stay ahead of the curve.
- Challenges:
- Data Quality: The effectiveness of behavioral pattern recognition relies on the quality and quantity of data collected. We emphasize data quality in our projects, ensuring that our clients have access to reliable and actionable insights.
- Ethical Considerations: There are concerns regarding privacy and consent when monitoring user behavior, necessitating transparent practices. Rapid Innovation is committed to ethical data practices, ensuring that our solutions respect user privacy while delivering valuable insights.
5. Personalization Strategies
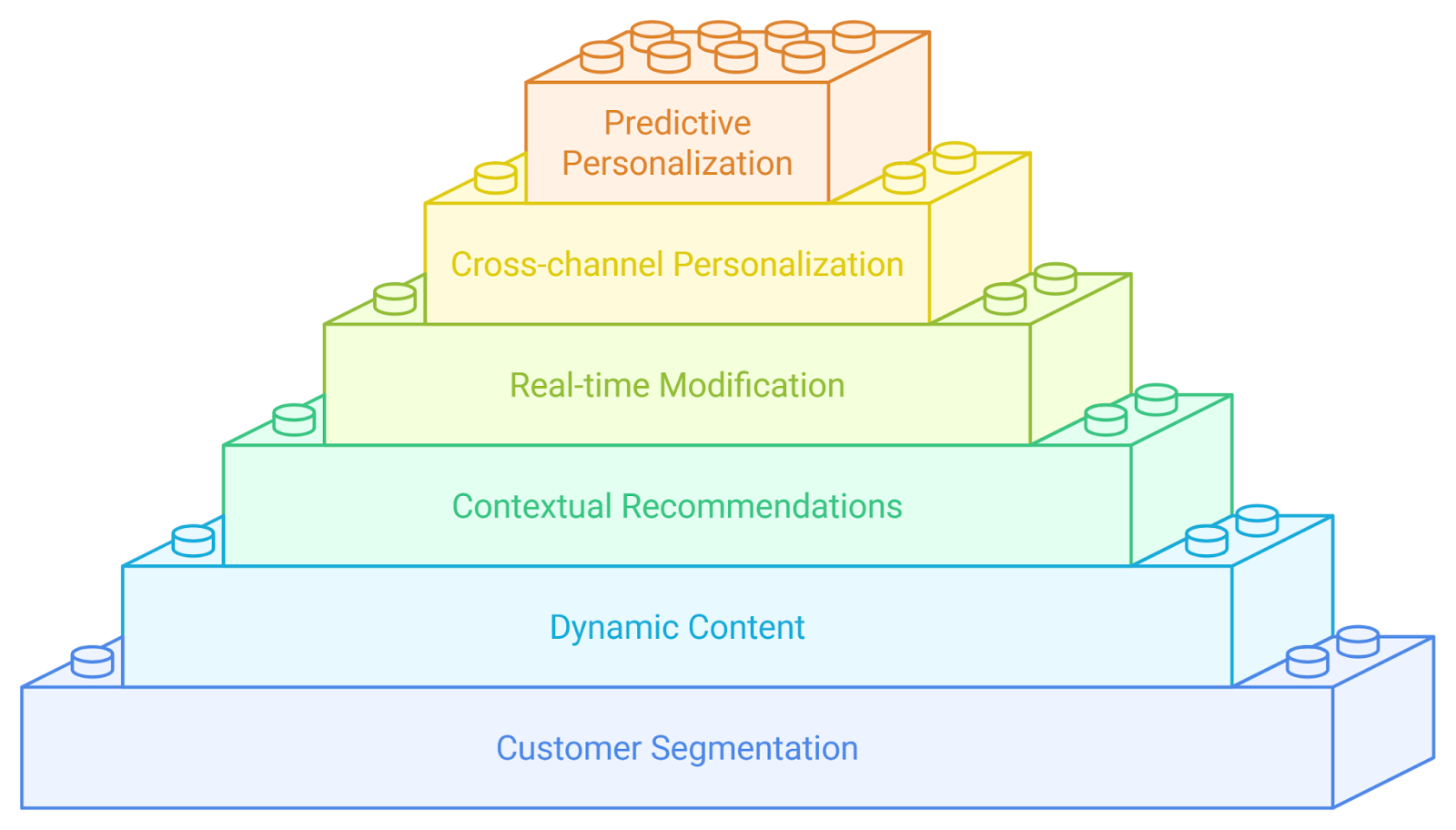
Personalization strategies are essential for businesses looking to enhance customer experience and drive engagement. By tailoring content and interactions to individual preferences, companies can foster loyalty and increase conversion rates. Here are two key components of effective personalization strategies: customer segmentation and dynamic content adaptation.
5.1. Customer Segmentation
Customer segmentation involves dividing a customer base into distinct groups based on shared characteristics. This allows businesses to tailor their marketing efforts and product offerings to meet the specific needs of each segment. Effective customer segmentation can lead to improved customer satisfaction and higher sales.
- Demographic Segmentation: This includes age, gender, income level, and education. Understanding these factors helps businesses create targeted marketing campaigns.
- Behavioral Segmentation: This focuses on customer behaviors, such as purchasing habits, brand loyalty, and product usage. Analyzing these behaviors can reveal insights into what drives customer decisions.
- Psychographic Segmentation: This involves understanding customers' lifestyles, values, and interests. By tapping into these deeper motivations, businesses can create more resonant messaging.
- Geographic Segmentation: This considers the location of customers, which can influence buying patterns. Tailoring offers based on regional preferences can enhance relevance.
- Benefits of Customer Segmentation:
- Increases marketing efficiency by targeting the right audience.
- Enhances customer experience through personalized interactions.
- Improves product development by aligning offerings with customer needs.
At Rapid Innovation, we leverage advanced AI algorithms to analyze customer data and implement effective segmentation strategies. This enables our clients to optimize their marketing efforts and achieve a higher return on investment (ROI).
5.2. Dynamic Content Adaptation
Dynamic content adaptation refers to the ability to change website or marketing content in real-time based on user behavior and preferences. This strategy enhances user engagement by providing a more relevant experience.
- Real-Time Data Utilization: By analyzing user data, businesses can adjust content on-the-fly. This includes changing product recommendations, promotional offers, and even website layouts based on user interactions.
- Personalized Recommendations: Utilizing algorithms, businesses can suggest products or services that align with individual customer preferences. This can significantly increase conversion rates.
- A/B Testing: This involves testing different content variations to see which performs better. By continuously optimizing content based on user feedback, businesses can improve engagement and satisfaction.
- User Journey Mapping: Understanding the customer journey allows businesses to tailor content at each stage. This ensures that users receive relevant information when they need it, enhancing the overall experience.
- Benefits of Dynamic Content Adaptation:
- Increases user engagement by providing relevant content.
- Boosts conversion rates through personalized recommendations.
- Enhances customer loyalty by creating a tailored experience.
Rapid Innovation employs cutting-edge AI technologies to facilitate dynamic content adaptation, ensuring that our clients can engage their customers effectively and drive higher conversion rates.
Incorporating customer segmentation and dynamic content adaptation into personalization strategies can significantly improve customer interactions and drive business success. By understanding and responding to individual customer needs, businesses can create a more engaging and effective marketing approach, ultimately leading to greater ROI. This includes implementing personalized video marketing, website personalization strategies, and customized marketing strategies to further enhance the customer experience. Additionally, our expertise in predictive analytics can help businesses anticipate customer needs and optimize their strategies accordingly. For more insights on enhancing content discovery workflows, check out our article on AI agents and content discovery.
5.3. Contextual Recommendations
Contextual recommendations are tailored suggestions provided to users based on their current situation, preferences, and behaviors. This approach enhances user engagement and satisfaction by delivering relevant content at the right moment.
- Contextual recommendations utilize data such as location, time of day, and user activity to personalize suggestions.
- For example, a user browsing a travel website may receive recommendations for nearby attractions based on their current location.
- Machine learning algorithms analyze user behavior patterns to predict what content or products may be of interest, allowing businesses to leverage AI for enhanced decision-making.
- Contextual recommendations can significantly improve conversion rates, as users are more likely to engage with content that resonates with their immediate context.
- Implementing contextual recommendations requires robust data collection and analysis systems to ensure accuracy and relevance, which Rapid Innovation can help establish through our expertise in AI and data analytics, including our services in AI-powered product recommendations in e-commerce.
5.4. Real-time Experience Modification
Real-time experience modification refers to the ability to adapt user experiences instantly based on their interactions and feedback. This dynamic approach allows businesses to create a more engaging and personalized environment for users.
- Real-time modifications can include changes to website layouts, content displays, or product recommendations based on user behavior.
- For instance, if a user spends a significant amount of time on a specific product page, the website can highlight similar products or offer discounts in real-time, enhancing the user experience.
- This technique relies on advanced analytics and tracking technologies to monitor user actions and preferences continuously, which Rapid Innovation can implement through our AI-driven solutions.
- Real-time experience modification can lead to increased user retention and satisfaction, as users feel that their needs are being addressed promptly.
- Businesses can leverage tools like A/B testing to refine real-time modifications and ensure they resonate with their audience, ultimately driving higher ROI.
5.5. Cross-channel Personalization
Cross-channel personalization involves delivering a consistent and tailored experience to users across multiple platforms and devices. This strategy ensures that users receive relevant content, regardless of where they interact with a brand.
- Cross-channel personalization requires a unified data strategy to track user interactions across various touchpoints, such as websites, mobile apps, and social media.
- By understanding user behavior across channels, businesses can create cohesive marketing campaigns that resonate with their audience, maximizing the effectiveness of their outreach.
- For example, a user who browses products on a mobile app may receive follow-up emails with personalized recommendations based on their app activity, showcasing the power of integrated data solutions.
- This approach enhances brand loyalty, as users appreciate a seamless experience that acknowledges their preferences and behaviors.
- Implementing cross-channel personalization can lead to higher engagement rates and improved customer satisfaction, as users feel recognized and valued across all interactions, ultimately contributing to greater business success. Rapid Innovation specializes in developing these integrated solutions to help clients achieve their business goals efficiently and effectively.
5.6. Predictive Personalization
Predictive personalization is a powerful strategy that leverages data analytics and machine learning to tailor experiences for individual users. By analyzing user behavior, preferences, and historical data, businesses can create highly personalized interactions that enhance customer satisfaction and drive engagement.
- Utilizes algorithms to predict user preferences based on past behavior.
- Increases conversion rates by delivering relevant content and recommendations.
- Enhances user experience by anticipating needs before they arise.
- Can be applied across various channels, including websites, emails, and social media.
- Helps in segmenting audiences more effectively, allowing for targeted marketing strategies.
For example, e-commerce platforms often use predictive personalization to recommend products based on previous purchases or browsing history. This not only improves the shopping experience but also increases the likelihood of additional sales. According to a study, personalized recommendations can lead to a 10-30% increase in sales (source: McKinsey). At Rapid Innovation, we assist clients in implementing predictive personalization strategies that drive significant ROI by enhancing customer engagement and satisfaction, including our chatbot development services and how AI agents are redefining sales and marketing.
6. Implementation Framework
An effective implementation framework is crucial for successfully integrating predictive personalization into a business strategy. This framework outlines the steps necessary to deploy predictive analytics and personalization techniques effectively.
- Establish clear objectives for what you want to achieve with predictive personalization.
- Identify the data sources required for analysis, including customer interactions, purchase history, and demographic information.
- Choose the right technology stack that supports data collection, analysis, and personalization.
- Develop a roadmap that outlines the timeline and milestones for implementation.
- Ensure cross-departmental collaboration to align marketing, IT, and customer service teams.
By following a structured implementation framework, businesses can ensure that their predictive personalization efforts are aligned with overall business goals and customer needs.
6.1. Assessment and Planning
Assessment and planning are critical initial steps in the implementation framework for predictive personalization. This phase involves evaluating current capabilities and defining a clear strategy for moving forward.
- Conduct a thorough analysis of existing data infrastructure and analytics capabilities.
- Identify gaps in data collection and quality that may hinder effective personalization.
- Define key performance indicators (KPIs) to measure the success of predictive personalization efforts.
- Engage stakeholders from various departments to gather insights and foster collaboration.
- Create a detailed project plan that includes timelines, resources, and budget considerations.
Effective assessment and planning set the foundation for successful predictive personalization initiatives. By understanding current capabilities and defining a clear strategy, businesses can maximize the impact of their personalization efforts and drive better customer engagement. Rapid Innovation is here to guide you through this process, ensuring that your predictive personalisation initiatives yield the highest possible returns.
6.1.1. Business Requirements Analysis:
Business requirements analysis is a critical step in the project management process. It involves identifying the needs and expectations of stakeholders to ensure that the project aligns with the overall business objectives. This analysis helps in defining the scope of the project and serves as a foundation for subsequent phases.
Understand stakeholder needs: Engage with stakeholders through interviews, surveys, and workshops to gather insights on their expectations. This engagement is crucial for aligning AI and Blockchain solutions with business goals, as well as conducting a swot analysis in project management.
Define project scope: Clearly outline what the project will deliver and what it will not, helping to prevent scope creep. This clarity is essential for ensuring that AI models and Blockchain implementations meet specific business requirements, similar to the critical path analysis in project management.
Document requirements: Create a comprehensive requirements document that includes functional and non-functional requirements. This documentation serves as a reference point for AI algorithms and Blockchain protocols, akin to the earned value analysis in project management.
Prioritize requirements: Use techniques like MoSCoW (Must have, Should have, Could have, Won't have) to prioritize requirements based on business value. This prioritization helps in focusing on high-impact AI features and Blockchain functionalities, which can be assessed through risk analysis in project management.
Validate requirements: Ensure that the documented requirements are reviewed and approved by stakeholders to confirm alignment with business goals. This validation process is vital for the successful deployment of AI and Blockchain solutions, similar to the earned value analysis example.
6.1.2. Technical Infrastructure Evaluation:
Technical infrastructure evaluation is essential for determining whether the existing technology and systems can support the project’s requirements. This evaluation helps in identifying gaps and areas for improvement, ensuring that the project is built on a solid foundation.
Assess current systems: Review existing hardware, software, and network capabilities to understand their strengths and weaknesses. This assessment is particularly important for AI processing power and Blockchain network capabilities, as well as conducting a critical path analysis ms project.
Identify technology gaps: Determine if current infrastructure can meet the project’s requirements or if new technology is needed. This identification is crucial for ensuring that AI models can be trained effectively and Blockchain networks can operate efficiently, similar to the monte carlo analysis in pmp.
Evaluate scalability: Consider whether the infrastructure can handle future growth and increased demand. Scalability is a key factor in both AI applications and Blockchain solutions to accommodate expanding data and transaction volumes, which can be visualized through a critical path network diagram.
Analyze security measures: Ensure that the technical infrastructure complies with security standards and can protect sensitive data. This analysis is critical for safeguarding AI data and ensuring the integrity of Blockchain transactions, akin to the cost benefit analysis in project management.
Plan for integration: Identify how new systems will integrate with existing ones to ensure seamless operation. Effective integration is essential for leveraging AI insights and Blockchain transparency across business processes, similar to the critical path analysis and pert charts.
6.1.3. Resource Planning:
Resource planning is a vital component of project management that involves identifying and allocating the necessary resources to achieve project objectives. Effective resource planning ensures that the project is completed on time and within budget.
Identify resource needs: Determine the types and quantities of resources required, including personnel, equipment, and materials. This identification is crucial for assembling teams with expertise in AI and Blockchain technologies, as well as conducting a project risk analysis.
Create a resource allocation plan: Develop a plan that outlines how resources will be distributed across the project timeline. This plan should account for the specific needs of AI development cycles and Blockchain deployment phases, similar to the critical paths in ms project.
Monitor resource availability: Keep track of resource availability to avoid conflicts and ensure that the right resources are in place when needed. This monitoring is essential for maintaining momentum in AI projects and Blockchain initiatives, akin to the monte carlo simulation pmp.
Optimize resource utilization: Implement strategies to maximize the efficiency of resource use, such as cross-training team members. This optimization can enhance the team's ability to adapt to the dynamic requirements of AI and Blockchain projects, similar to assessing project risk.
Adjust plans as necessary: Be prepared to adapt resource plans based on project changes, delays, or unforeseen challenges. Flexibility in resource planning is vital for navigating the complexities of AI and Blockchain implementations, as well as conducting an analysis of risk in project management.
6.2. Development Methodology
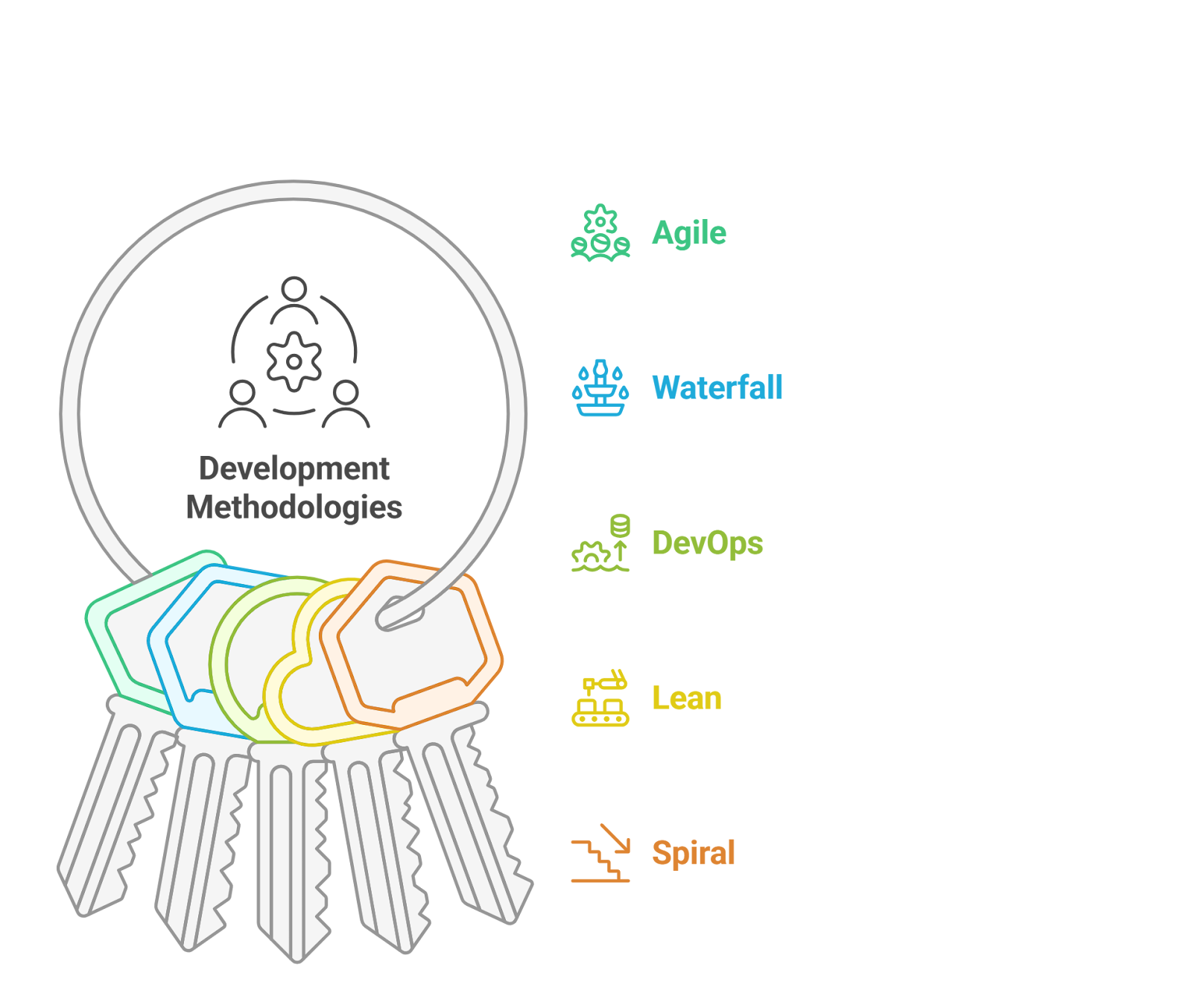
The development methodology is a structured approach to software development that outlines how a project will be planned, executed, and monitored. Choosing the right methodology is crucial for the success of any software project, especially in the rapidly evolving fields of AI and Blockchain.
- Agile: This iterative approach focuses on collaboration, customer feedback, and small, rapid releases. Agile methodologies, such as Scrum and Kanban, allow teams to adapt to changes quickly and deliver functional software in shorter cycles. For instance, in AI projects, Agile enables teams to refine algorithms based on user feedback, leading to more effective solutions. Agile software development methodologies, including adaptive software development and scrum development, are widely adopted for their flexibility and responsiveness.
- Waterfall: A traditional model where each phase must be completed before the next begins. This linear approach is suitable for projects with well-defined requirements and minimal expected changes. In Blockchain development, where regulatory compliance is critical, a Waterfall approach can ensure thorough documentation and adherence to standards.
- DevOps: This methodology integrates development and operations teams to improve collaboration and productivity. It emphasizes automation, continuous integration, and continuous delivery (CI/CD) to streamline the software development lifecycle. For AI applications, DevOps practices can facilitate rapid deployment of machine learning models, enhancing responsiveness to market needs.
- Lean: Focuses on maximizing value while minimizing waste. Lean principles encourage teams to identify and eliminate non-value-adding activities, leading to more efficient processes. In Blockchain projects, Lean methodologies can help streamline processes, reducing the time to market and increasing ROI.
- Spiral: Combines iterative development with the systematic risk assessment of the waterfall model. This approach is beneficial for large, complex projects where risks need to be managed carefully. In AI and Blockchain initiatives, the Spiral model allows for ongoing risk evaluation, ensuring that potential issues are addressed proactively.
Selecting the appropriate development methodology depends on various factors, including project size, complexity, team structure, and stakeholder involvement. At Rapid Innovation, we leverage our expertise in these methodologies, including agile software development project management and the manifesto for agile software development, to tailor solutions that align with our clients' specific business goals, ultimately driving greater ROI.
6.3. Integration Strategies
Integration strategies are essential for ensuring that different software components work together seamlessly. Effective integration can enhance functionality, improve user experience, and streamline workflows.
- Continuous Integration (CI): This practice involves regularly merging code changes into a central repository. Automated tests are run to detect issues early, allowing teams to address problems before they escalate.
- API Integration: Application Programming Interfaces (APIs) enable different software systems to communicate. By using APIs, developers can integrate third-party services, enhancing the functionality of their applications.
- Middleware Solutions: Middleware acts as a bridge between different applications, facilitating communication and data exchange. It can simplify integration by providing a common platform for disparate systems.
- Data Integration: This strategy focuses on combining data from different sources to provide a unified view. Techniques such as ETL (Extract, Transform, Load) and data warehousing are commonly used for effective data integration.
- Microservices Architecture: This approach breaks down applications into smaller, independent services that can be developed, deployed, and scaled individually. Microservices enhance flexibility and allow for easier integration of new features.
Choosing the right integration strategy depends on the specific needs of the project, the existing technology stack, and the desired level of scalability and flexibility.
6.4. Testing and Validation
Testing and validation are critical components of the software development lifecycle. They ensure that the software meets the required standards and functions as intended.
- Unit Testing: This involves testing individual components or functions of the software to ensure they work correctly. Unit tests are typically automated and help catch bugs early in the development process.
- Integration Testing: This type of testing focuses on the interactions between different components or systems. It ensures that integrated parts work together as expected and helps identify issues that may arise during integration.
- System Testing: Conducted on the complete system, this testing verifies that the software meets the specified requirements. It includes functional and non-functional testing, such as performance, security, and usability testing.
- User Acceptance Testing (UAT): This final phase of testing involves real users testing the software to ensure it meets their needs and expectations. UAT is crucial for validating that the software is ready for deployment.
- Regression Testing: After changes or updates are made, regression testing ensures that existing functionalities remain unaffected. This is essential for maintaining software quality over time.
Implementing a robust testing and validation strategy helps identify defects early, reduces the risk of failures, and ensures a high-quality product that meets user expectations. At Rapid Innovation, we prioritize these practices to deliver solutions that not only meet but exceed client expectations, ultimately driving greater ROI.
6.5. Deployment Models
Deployment models refer to the specific configurations and environments in which cloud services are made available to users. Understanding these models, including cloud deployment models and cloud computing deployment models, is crucial for businesses to choose the right cloud solution that aligns with their operational needs and security requirements. The primary deployment models include:
- Public Cloud: Services are delivered over the internet and shared across multiple organizations. This model is cost-effective due to shared resources. Examples include Amazon Web Services (AWS), Microsoft Azure, and Google Cloud Platform.
- Private Cloud: This model features dedicated infrastructure for a single organization, providing enhanced security and control. It is ideal for businesses with strict regulatory requirements or sensitive data and can be hosted on-premises or by a third-party provider. The private cloud deployment model is particularly beneficial for organizations that prioritize data security.
- Hybrid Cloud: This model combines public and private clouds, allowing data and applications to be shared between them. It offers flexibility and scalability while maintaining security for sensitive data, making it suitable for businesses that need to scale resources quickly while keeping critical operations secure. Hybrid cloud deployment is increasingly popular among organizations looking for a balanced approach.
- Community Cloud: This model provides shared infrastructure for a specific community of users with common concerns (e.g., security, compliance). It is cost-effective as resources are pooled among several organizations and is often used by government agencies or organizations within the same industry. Examples of community cloud computing include government community clouds and other collaborative efforts.
Choosing the right deployment model, whether it be a deployment model for cloud computing or a specific cloud deployment method, depends on various factors, including budget, security needs, and compliance requirements. Organizations must evaluate their specific needs to determine the most suitable model for their operations. At Rapid Innovation, we assist clients in navigating these options, ensuring they select a deployment model that maximizes efficiency and aligns with their strategic goals.
7. Industry-Specific Applications
Industry-specific applications are tailored software solutions designed to meet the unique needs of particular sectors. These applications enhance efficiency, streamline processes, and improve customer experiences. Various industries benefit from specialized applications, including healthcare, finance, manufacturing, and more.
- Healthcare: Applications for electronic health records (EHR), telemedicine, and patient management focus on improving patient care, data security, and regulatory compliance.
- Finance: Solutions for risk management, fraud detection, and regulatory compliance enhance customer service through personalized banking experiences.
- Manufacturing: Applications for supply chain management, inventory control, and production planning improve operational efficiency and reduce costs.
7.1. E-commerce and Retail
E-commerce and retail industries have seen significant transformations due to technological advancements. Industry-specific applications in this sector focus on enhancing customer experiences, optimizing operations, and driving sales. Key applications include:
- Online Storefronts: Platforms like Shopify and WooCommerce enable businesses to set up and manage online stores easily, featuring product listings, payment processing, and inventory management.
- Customer Relationship Management (CRM): Tools like Salesforce and HubSpot help retailers manage customer interactions and data, enhancing customer engagement through personalized marketing and support.
- Inventory Management Systems: These applications track stock levels, orders, and deliveries, helping businesses maintain optimal inventory levels and reduce costs.
- Analytics and Business Intelligence: Tools that analyze customer behavior, sales trends, and market conditions enable data-driven decision-making to improve marketing strategies and product offerings.
- Mobile Applications: Retailers are increasingly developing mobile apps to enhance customer engagement, with features that may include mobile shopping, loyalty programs, and personalized promotions.
- Supply Chain Management: Applications that streamline logistics, procurement, and distribution processes improve efficiency and reduce lead times in product delivery.
The integration of these applications allows e-commerce and retail businesses to remain competitive in a rapidly evolving market. By leveraging technology, companies can enhance customer satisfaction, optimize operations, and ultimately drive growth. Rapid Innovation is committed to providing tailored solutions that empower businesses to harness the full potential of these industry-specific applications, ensuring they achieve greater ROI and operational excellence.
7.2. Financial Services
The financial services sector is a critical component of the global economy, encompassing a wide range of services that facilitate the management of money. This sector includes banking, investment, insurance, and real estate services.
- Banking: Traditional banks, such as bb&t corporation and pnc personal loans, offer services such as savings accounts, loans, and mortgages. Online banking has revolutionized how consumers manage their finances, providing convenience and accessibility. Rapid Innovation leverages AI to enhance customer service through chatbots and personalized financial advice, improving user experience and operational efficiency.
- Investment: Investment firms help individuals and institutions grow their wealth through stocks, bonds, and mutual funds. The rise of robo-advisors has made investment management more accessible to the average consumer. By utilizing AI algorithms, Rapid Innovation can assist clients in developing sophisticated investment strategies that optimize returns while minimizing risks. Companies like ubs financial services inc and voya life insurance are key players in this space.
- Insurance: Insurance companies, including trustage insurance and ally auto insurance, provide risk management solutions, protecting individuals and businesses from financial loss. This includes life, health, property, and liability insurance. Rapid Innovation employs blockchain technology to streamline claims processing and enhance transparency, ultimately reducing fraud and improving customer trust.
- Real Estate: Real estate services encompass property management, real estate investment, and brokerage services. The real estate market is influenced by economic conditions, interest rates, and demographic trends. Rapid Innovation can implement AI-driven analytics to provide insights into market trends, helping clients make informed investment decisions.
The financial services industry is heavily regulated to protect consumers and maintain market stability. Innovations such as fintech are transforming the landscape, offering new solutions for payments, lending, and investment. According to a report by Statista, the global fintech market is expected to reach $305 billion by 2025. Rapid Innovation is at the forefront of this transformation, providing tailored AI banking solutions that help clients achieve greater ROI and operational efficiency. Companies like synchrony bank credit card, td auto financing, and onemain loan are examples of innovative financial service providers.
7.3. Healthcare Services
Healthcare services are essential for maintaining public health and improving quality of life. This sector includes a wide range of services provided by hospitals, clinics, and healthcare professionals.
- Primary Care: Primary care providers are often the first point of contact for patients. They offer preventive care, diagnosis, and treatment for various health issues.
- Specialty Care: Specialists focus on specific areas of medicine, such as cardiology, oncology, or orthopedics. They provide advanced care and treatment options for complex health conditions.
- Mental Health Services: Mental health is a crucial aspect of overall well-being. Services include counseling, therapy, and psychiatric care to address mental health disorders.
- Telehealth: The rise of telehealth has made healthcare more accessible, allowing patients to consult with healthcare providers remotely. This trend has gained momentum, especially during the COVID-19 pandemic.
Healthcare services are increasingly focused on patient-centered care, emphasizing the importance of patient engagement and satisfaction. The integration of technology, such as electronic health records (EHRs) and health apps, is enhancing the efficiency and effectiveness of healthcare delivery. According to the World Health Organization, global spending on health is projected to reach $10 trillion by 2022.
7.4. Entertainment and Media
The entertainment and media industry encompasses a diverse range of activities that provide enjoyment and information to audiences. This sector includes film, television, music, gaming, and digital media.
- Film and Television: The film and television industry produces content for cinemas and streaming platforms. The rise of streaming services like Netflix and Disney+ has transformed how audiences consume media.
- Music: The music industry includes recording, distribution, and live performances. Digital platforms have changed how music is consumed, with streaming services dominating the market.
- Gaming: The gaming industry has seen exponential growth, with video games becoming a major form of entertainment. Mobile gaming, in particular, has expanded the audience significantly.
- Digital Media: Social media platforms and online content creation have revolutionized how information is shared and consumed. Influencers and content creators play a significant role in shaping trends and audience preferences.
The entertainment and media industry is heavily influenced by technology and consumer behavior. As audiences demand more personalized and interactive experiences, companies are adapting their strategies to meet these expectations. According to PwC, the global entertainment and media market is projected to grow to $2.6 trillion by 2023. Rapid Innovation is committed to helping clients in this sector harness AI and blockchain technologies to create engaging content and optimize distribution channels, ultimately driving growth and enhancing audience engagement.
7.5. Travel and Hospitality
The travel and hospitality industry has undergone significant transformation due to technological advancements and changing consumer preferences. This sector encompasses a wide range of services, including hotels, airlines, travel agencies, and tour operators.
- Enhanced Customer Experience:
- Personalization is key. Companies are leveraging data analytics to tailor experiences based on individual preferences. Rapid Innovation can assist businesses in implementing AI-driven analytics solutions that analyze customer data to create personalized travel itineraries, enhancing customer satisfaction and loyalty.
- Mobile apps allow for seamless booking, check-ins, and customer service interactions. Our expertise in mobile app development can help clients create intuitive applications that streamline these processes, ultimately improving operational efficiency.
- Online Booking Platforms:
- Websites and apps like Expedia and Booking.com have revolutionized how travelers plan their trips. These platforms provide user-friendly interfaces, competitive pricing, and extensive reviews, making it easier for consumers to make informed decisions. Rapid Innovation can help clients develop robust online booking systems that integrate AI for dynamic pricing and personalized recommendations, driving higher conversion rates. Our hospitality software development services can further enhance these platforms.
- Sustainable Travel:
- There is a growing trend towards eco-friendly travel options, with many travelers seeking sustainable accommodations and experiences. Companies are adopting green practices, such as reducing waste and supporting local communities. Rapid Innovation can guide clients in implementing blockchain solutions to enhance transparency in sustainable practices, allowing travelers to make informed choices.
- Virtual Reality (VR) and Augmented Reality (AR):
- VR and AR technologies are being used to enhance the travel experience, allowing potential travelers to explore destinations before booking. Hotels and resorts are using these technologies for virtual tours, helping customers visualize their stay. Our team can develop immersive VR and AR applications that provide engaging previews of travel experiences, increasing customer interest and bookings.
- Social Media Influence:
- Social media platforms play a crucial role in shaping travel decisions, with travelers often seeking inspiration from influencers and user-generated content. Brands are increasingly using social media marketing to engage with customers and promote their offerings. Rapid Innovation can assist in creating AI-driven social media analytics tools that help clients understand consumer sentiment and optimize their marketing strategies.
- Travel and Hospitality Services:
- The integration of technology in travel and hospitality services is essential for meeting the evolving demands of consumers. Companies must adapt to these changes to remain competitive in the market. Our expertise in hospitality software development can provide tailored solutions that enhance service delivery and operational efficiency. For more insights on how technology, particularly generative AI, is transforming the travel industry, check out this article on how generative AI is revolutionizing the travel industry.
7.6. Education and Learning
The education sector is experiencing a digital revolution, driven by advancements in technology and the need for flexible learning options. This transformation is reshaping how knowledge is delivered and consumed.
- E-Learning Platforms:
- Online learning platforms like Coursera and Udemy offer a wide range of courses, making education accessible to a global audience. These platforms provide flexibility, allowing learners to study at their own pace and convenience. Rapid Innovation can help educational institutions develop customized e-learning solutions that incorporate AI for personalized learning paths.
- Blended Learning:
- Combining traditional classroom methods with online learning creates a more engaging and effective educational experience. This approach caters to different learning styles and helps educators reach a broader range of students. Our expertise in AI can enhance blended learning environments by providing real-time analytics on student performance.
- Gamification:
- Incorporating game-like elements into educational content enhances engagement and motivation among learners. Gamification techniques, such as rewards and challenges, make learning more interactive and enjoyable. Rapid Innovation can assist in designing gamified learning experiences that leverage AI to adapt to individual learner progress.
- Artificial Intelligence (AI) in Education:
- AI is being used to personalize learning experiences, providing tailored content based on individual student needs. Intelligent tutoring systems can offer real-time feedback and support, improving learning outcomes. Our AI solutions can empower educational institutions to implement adaptive learning technologies that enhance student engagement and success.
- Lifelong Learning:
- The concept of lifelong learning is gaining traction, with individuals seeking continuous education to adapt to changing job markets. Organizations are investing in employee training programs to foster skill development and career growth. Rapid Innovation can help companies develop AI-driven training platforms that provide personalized learning experiences for employees.
8. Advanced Features and Capabilities

As industries evolve, the demand for advanced features and capabilities in products and services is increasing. This trend is particularly evident in technology-driven sectors, where innovation is key to staying competitive.
- Automation:
- Automation technologies streamline processes, reduce human error, and enhance efficiency across various industries. Businesses are adopting robotic process automation (RPA) to handle repetitive tasks, freeing up employees for more strategic work. Rapid Innovation can implement RPA solutions tailored to specific business needs, driving operational efficiency.
- Data Analytics:
- Advanced data analytics tools enable organizations to extract valuable insights from large datasets, driving informed decision-making. Predictive analytics helps businesses anticipate trends and customer behavior, allowing for proactive strategies. Our expertise in data analytics can help clients harness their data for actionable insights, improving ROI.
- Cloud Computing:
- Cloud technology offers scalable solutions, enabling businesses to access resources and data from anywhere. This flexibility supports remote work and collaboration, essential in today’s digital landscape. Rapid Innovation can assist in migrating clients to cloud-based solutions that enhance collaboration and reduce IT costs.
- Internet of Things (IoT):
- IoT devices are transforming industries by connecting everyday objects to the internet, facilitating real-time data exchange. This connectivity enhances operational efficiency and provides valuable insights into consumer behavior. Our IoT solutions can help clients leverage connected devices to optimize operations and improve customer experiences.
- Enhanced Security Features:
- As cyber threats become more sophisticated, advanced security measures are essential for protecting sensitive data. Companies are investing in multi-factor authentication, encryption, and AI-driven security solutions to safeguard their systems. Rapid Innovation can develop comprehensive security frameworks that utilize AI for threat detection and response.
- User-Centric Design:
- Focusing on user experience (UX) is crucial for product development, ensuring that features meet the needs and preferences of end-users. Intuitive interfaces and accessibility options enhance usability, making products more appealing to a broader audience. Our design team specializes in creating user-centric solutions that drive engagement and satisfaction.
8.1. Multi-modal Interaction
Multi-modal interaction refers to the ability of systems to engage with users through various modes of communication, such as voice, text, gestures, and visual inputs. This approach enhances user experience by providing flexibility and convenience.
- Users can choose their preferred mode of interaction, making technology more accessible.
- Multi-modal systems can combine inputs, allowing for richer communication. For example, a user might speak a command while simultaneously pointing at an object on a screen.
- This interaction style is particularly beneficial in environments where hands-free operation is necessary, such as in vehicles or for individuals with disabilities.
- Technologies like virtual assistants exemplify multimodal interaction by responding to voice commands while also providing visual feedback on screens.
- The integration of augmented reality (AR) and virtual reality (VR) further enhances multimodal human computer interaction, allowing users to engage with digital content in immersive ways.
At Rapid Innovation, we harness the power of multimodal interaction in HCI to create tailored solutions that enhance user engagement and streamline processes for our clients. By implementing these systems, businesses can improve customer satisfaction and drive greater ROI through more effective communication channels.
8.2. Emotion-aware Services
Emotion-aware services leverage artificial intelligence and machine learning to recognize and respond to users' emotional states. This capability can significantly improve user engagement and satisfaction.
- By analyzing voice tone, facial expressions, and text sentiment, these services can tailor responses to match the user's emotional context.
- For instance, customer service chatbots equipped with emotion recognition can adjust their tone and responses based on the user's frustration or satisfaction levels.
- Emotion-aware technology can enhance mental health applications by providing support and resources tailored to users' emotional needs.
- Businesses can benefit from emotion-aware services by improving customer interactions, leading to higher retention rates and loyalty.
- Research indicates that emotionally intelligent systems can lead to a 20% increase in user satisfaction.
At Rapid Innovation, we integrate emotion-aware services into our client solutions, enabling businesses to foster deeper connections with their customers. This not only enhances user experience but also contributes to increased loyalty and a higher return on investment.
8.3. Proactive Service Delivery
Proactive service delivery involves anticipating user needs and addressing them before they become issues. This forward-thinking approach can enhance user experience and operational efficiency.
- Systems can analyze user behavior and preferences to predict future needs, allowing for timely interventions.
- For example, smart home devices can learn a user's schedule and adjust heating or lighting automatically, creating a comfortable environment without user input.
- Proactive customer service can involve reaching out to users before they encounter problems, such as notifying them of potential service disruptions or offering solutions based on usage patterns.
- This approach not only improves user satisfaction but also reduces the workload on customer support teams by minimizing reactive service requests.
- Companies that implement proactive service strategies often see a significant reduction in customer complaints and an increase in overall satisfaction.
At Rapid Innovation, we empower our clients to adopt proactive service delivery models, leveraging AI and data analytics to anticipate customer needs. This strategic approach not only enhances user satisfaction but also optimizes operational efficiency, ultimately leading to a more substantial return on investment.
8.4. Adaptive Learning Systems
Adaptive learning systems are educational technologies that tailor the learning experience to individual student needs. These systems utilize algorithms and data analytics to assess a learner's strengths, weaknesses, and preferences, allowing for a personalized educational journey.
- Personalized Learning Paths: Adaptive learning systems create customized learning paths based on the learner's progress and performance. This ensures that students engage with material that is neither too easy nor too difficult.
- Real-time Feedback: These systems provide immediate feedback, helping learners understand their mistakes and correct them promptly. This instant response fosters a more effective learning environment.
- Data-Driven Insights: By analyzing student data, adaptive learning systems can identify trends and patterns in learning behaviors. Educators can use this information to adjust their teaching strategies and improve overall student outcomes.
- Scalability: Adaptive learning systems can cater to a large number of students simultaneously, making them ideal for online education platforms and large classrooms.
- Continuous Improvement: As students interact with the system, it continuously learns and adapts, ensuring that the educational content remains relevant and effective.
At Rapid Innovation, we leverage AI technologies to develop adaptive learning platforms and adaptive learning systems that not only enhance the educational experience but also drive greater ROI for educational institutions. By implementing these systems, clients can expect improved student engagement and retention rates, ultimately leading to better financial performance. For more insights on integrating AI in educational evaluation.
8.5. Cross-platform Synchronization
Cross-platform synchronization refers to the ability of educational tools and resources to function seamlessly across various devices and platforms. This capability is essential in today’s digital learning environment, where students may use multiple devices for their studies.
- Device Flexibility: Students can access learning materials on smartphones, tablets, laptops, or desktops, allowing for a more flexible learning experience.
- Consistent User Experience: Cross-platform synchronization ensures that users have a consistent experience regardless of the device they are using. This uniformity helps reduce confusion and enhances usability.
- Data Synchronization: Progress and performance data are synchronized across devices, allowing students to pick up where they left off, regardless of the device they are using.
- Enhanced Collaboration: Cross-platform tools facilitate collaboration among students and educators, enabling them to work together in real-time, regardless of their location or device.
- Increased Engagement: By allowing students to access content anytime and anywhere, cross-platform synchronization can lead to higher engagement levels and improved learning outcomes.
Rapid Innovation specializes in creating cross-platform solutions that ensure seamless access to educational resources. This not only enhances the learning experience but also maximizes the reach and impact of educational programs, leading to increased enrollment and revenue for our clients.
9. Performance Measurement and Optimization
Performance measurement and optimization are critical components of effective educational systems. These processes involve assessing the effectiveness of teaching methods, learning materials, and overall student performance to enhance educational outcomes.
- Key Performance Indicators (KPIs): Establishing KPIs helps educators measure student progress and the effectiveness of instructional strategies. Common KPIs include test scores, completion rates, and engagement levels.
- Data Analytics: Utilizing data analytics tools allows educators to gather insights from student performance data. This information can be used to identify areas for improvement and to tailor instructional approaches.
- Continuous Assessment: Regular assessments, both formative and summative, provide ongoing feedback about student learning. This helps educators make timely adjustments to their teaching methods.
- Feedback Loops: Creating feedback loops between students and educators fosters a culture of continuous improvement. Students can share their experiences, and educators can adapt their strategies accordingly.
- Technology Integration: Leveraging technology for performance measurement can streamline the process. Learning management systems (LMS), including adaptive learning LMS, often include built-in analytics tools that track student progress and engagement.
- Iterative Improvement: Performance measurement is not a one-time event; it requires ongoing evaluation and refinement. By continuously optimizing teaching methods and learning materials, educators can enhance the overall educational experience.
At Rapid Innovation, we utilize advanced data analytics and AI-driven insights to help educational institutions measure and optimize their performance effectively. By focusing on data-driven decision-making, our clients can achieve significant improvements in student outcomes and operational efficiency, ultimately leading to a higher return on investment.
9.1. Key Performance Indicators
Key Performance Indicators (KPIs) are essential metrics that help organizations measure their success in achieving specific objectives. They provide a clear framework for evaluating performance and making informed decisions.
- Definition: KPIs are quantifiable measures that reflect the critical success factors of an organization. They can be financial, operational, or customer-focused.
- Types of KPIs:
- Quantitative KPIs: These are numerical indicators, such as sales revenue, profit margins, or customer acquisition costs.
- Qualitative KPIs: These focus on subjective measures, like customer satisfaction or brand reputation.
- Leading KPIs: Predict future performance, such as the number of new leads generated.
- Lagging KPIs: Reflect past performance, like total sales in the last quarter.
- Setting Effective KPIs:
- Specific: Clearly define what is being measured.
- Measurable: Ensure that the KPI can be quantified.
- Achievable: Set realistic targets that can be met.
- Relevant: Align KPIs with business goals.
- Time-bound: Establish a timeframe for achieving the KPI.
- Examples of KPIs:
- Customer retention rate
- Average order value
- Website traffic growth
- Key performance indicators examples
- Importance of KPIs:
- Help track progress towards goals
- Facilitate data-driven decision-making
- Enhance accountability within teams
At Rapid Innovation, we leverage AI and Blockchain technologies to help clients define and track KPIs that align with their strategic objectives. For instance, by implementing AI-driven analytics, we can provide real-time insights into customer behavior, enabling businesses to adjust their strategies promptly and improve their key performance indicators.
9.2. Analytics and Reporting
Analytics and reporting are crucial components of any data-driven strategy. They enable organizations to gather insights from data, assess performance, and make informed decisions.
- Definition: Analytics involves the systematic computational analysis of data, while reporting refers to the presentation of this data in a structured format.
- Types of Analytics:
- Descriptive Analytics: Analyzes historical data to understand what happened.
- Diagnostic Analytics: Investigates why something happened by identifying patterns and correlations.
- Predictive Analytics: Uses statistical models to forecast future outcomes based on historical data.
- Prescriptive Analytics: Recommends actions based on data analysis to achieve desired outcomes.
- Key Components of Reporting:
- Data Visualization: Use charts, graphs, and dashboards to present data clearly.
- Regular Reporting: Establish a routine for generating reports (daily, weekly, monthly).
- Stakeholder Communication: Tailor reports to meet the needs of different stakeholders, ensuring clarity and relevance.
- Tools for Analytics and Reporting:
- Google Analytics for web traffic analysis
- Tableau for data visualization
- Microsoft Power BI for business intelligence
- Benefits of Analytics and Reporting:
- Improved decision-making based on data insights
- Enhanced operational efficiency
- Better understanding of customer behavior and preferences
Rapid Innovation employs advanced analytics tools powered by AI to help clients derive actionable insights from their data. By utilizing predictive analytics, we can forecast trends and behaviors, allowing businesses to make proactive decisions that enhance their ROI.
9.3. A/B Testing Frameworks
A/B testing is a powerful method for optimizing marketing strategies, product features, and user experiences. It involves comparing two versions of a variable to determine which one performs better.
- Definition: A/B testing, also known as split testing, is a randomized experiment with two variants, A and B, to assess which one yields better results.
- Key Steps in A/B Testing:
- Identify the Goal: Determine what you want to improve (e.g., conversion rate, click-through rate).
- Select the Variable: Choose the element to test, such as headlines, images, or call-to-action buttons.
- Create Variants: Develop two versions (A and B) with one differing element.
- Randomly Assign Users: Split your audience randomly to ensure unbiased results.
- Analyze Results: Use statistical analysis to determine which variant performed better.
- Best Practices for A/B Testing:
- Test one variable at a time to isolate its impact.
- Ensure a large enough sample size for reliable results.
- Run tests for a sufficient duration to account for variability.
- Common Use Cases:
- Email marketing campaigns
- Landing page designs
- Pricing strategies
- Benefits of A/B Testing:
- Data-driven decision-making
- Increased conversion rates
- Enhanced user experience through continuous improvement.
At Rapid Innovation, we utilize A/B testing frameworks to optimize our clients' digital strategies. By systematically testing different approaches, we help businesses identify the most effective tactics, leading to improved customer engagement and higher conversion rates, ultimately driving greater ROI.
9.4. Continuous Improvement Strategies
Continuous improvement strategies are essential for organizations aiming to enhance their processes, products, and services consistently. These strategies focus on incremental changes that lead to significant improvements over time.
- Kaizen: This Japanese term means "change for better." It emphasizes small, continuous changes that can lead to substantial improvements. Employees at all levels are encouraged to suggest changes, fostering a culture of innovation. At Rapid Innovation, we leverage AI tools to analyze employee suggestions and identify the most impactful changes, ensuring that innovation is data-driven and aligned with business goals. This aligns with continuous improvement strategies for sustainability.
- Plan-Do-Check-Act (PDCA): This iterative four-step management method is used for the control and continuous improvement of processes and products. It involves:
- Planning a change
- Implementing the change
- Checking the results
- Acting on what is learned
Our team utilizes AI analytics to monitor the results of implemented changes, allowing for real-time adjustments and ensuring that improvements are effective and sustainable. This method is a key component of a continuous improvement strategic plan.
- Lean Methodology: Lean focuses on minimizing waste without sacrificing productivity. It involves:
- Identifying value from the customer’s perspective
- Mapping the value stream
- Creating flow by eliminating waste
- Establishing pull systems
- Pursuing perfection
By integrating blockchain technology, we can enhance transparency in the value stream, ensuring that every step adds value and reduces waste, ultimately leading to higher ROI. This is part of our continuous improvement business strategy.
- Six Sigma: This data-driven approach aims to improve quality by identifying and removing causes of defects. It uses statistical methods to measure and improve operational performance. Rapid Innovation employs advanced AI algorithms to analyze data patterns, enabling us to identify defects and implement corrective actions swiftly. This is one of the continuous improvement strategies in TQM.
- Employee Training and Development: Investing in employee skills and knowledge is crucial for continuous improvement. Regular training sessions can help employees stay updated with the latest industry practices and technologies. We offer tailored training programs that incorporate AI and blockchain concepts, ensuring that your team is equipped with the skills needed to drive innovation. This is essential for implementing continuous improvement strategies for service organizations.
- Feedback Mechanisms: Establishing channels for feedback from customers and employees can provide valuable insights into areas needing improvement. Surveys, suggestion boxes, and regular meetings can facilitate this process. Our AI-driven feedback systems analyze responses in real-time, allowing organizations to adapt quickly to stakeholder needs. This is a vital part of the constant improvement strategy.
9.5. ROI Assessment Methods
Return on Investment (ROI) assessment methods are critical for evaluating the effectiveness of investments in projects, initiatives, or strategies. Understanding ROI helps organizations make informed decisions about resource allocation.
- Simple ROI Calculation: This basic formula calculates ROI by comparing the net profit of an investment to its cost. The formula is:
language="language-plaintext"ROI = (Net Profit / Cost of Investment) x 100
This method provides a straightforward percentage that indicates the profitability of an investment.
- Time-Weighted ROI: This method accounts for the time period over which the investment is held. It is particularly useful for investments that span multiple years. It helps in understanding the annualized return, making it easier to compare different investments.
- Net Present Value (NPV): NPV assesses the profitability of an investment by calculating the present value of expected future cash flows, discounted back to the present. A positive NPV indicates that the investment is likely to be profitable.
- Internal Rate of Return (IRR): This method calculates the discount rate that makes the NPV of all cash flows from an investment equal to zero. It helps in comparing the profitability of different investments.
- Payback Period: This method measures the time it takes for an investment to generate enough cash flow to recover its initial cost. A shorter payback period is generally preferred, as it indicates quicker returns.
- Benchmarking: Comparing ROI with industry standards or competitors can provide context to the assessment. This helps organizations understand their performance relative to others in the same sector.
10. Ethical Considerations and Best Practices
Ethical considerations and best practices are vital for maintaining integrity and trust in business operations. Organizations must navigate complex ethical dilemmas while ensuring compliance with laws and regulations.
- Transparency: Being open about business practices, decision-making processes, and financial reporting fosters trust among stakeholders. Transparency can enhance an organization’s reputation and build customer loyalty.
- Fair Treatment: Ensuring fair treatment of employees, customers, and suppliers is crucial. This includes equitable pay, non-discriminatory practices, and fair negotiation terms.
- Sustainability: Ethical considerations extend to environmental impact. Organizations should adopt sustainable practices that minimize harm to the environment, such as reducing waste and conserving resources.
- Data Privacy: With the rise of digital technology, protecting customer data has become paramount. Organizations must comply with data protection regulations and implement robust security measures to safeguard personal information.
- Corporate Social Responsibility (CSR): Engaging in CSR initiatives demonstrates a commitment to ethical practices. This can include community involvement, charitable contributions, and environmentally friendly practices.
- Whistleblower Protections: Establishing mechanisms for employees to report unethical behavior without fear of retaliation is essential. This encourages a culture of accountability and integrity.
- Regular Training: Providing ongoing ethics training for employees helps reinforce the importance of ethical behavior and decision-making. This can include workshops, seminars, and online courses.
- Stakeholder Engagement: Actively involving stakeholders in decision-making processes can lead to more ethical outcomes. This includes seeking input from employees, customers, and community members.
By implementing these strategies, organizations can foster a culture of continuous improvement, accurately assess ROI, and uphold ethical standards in their operations. Rapid Innovation is committed to helping clients achieve these goals through our expertise in AI and blockchain technologies, ensuring that your organization not only meets but exceeds its business objectives.
10.1. Privacy Protection
Privacy protection is a critical aspect of the digital landscape, especially as technology continues to evolve. With the increasing amount of personal data being collected, it is essential to implement robust measures to safeguard user information. At Rapid Innovation, we leverage our expertise in AI and Blockchain to help clients enhance their data privacy strategy. Key strategies include:
- Data Encryption: Encrypting sensitive data ensures that even if it is intercepted, it remains unreadable to unauthorized users. Our blockchain solutions can provide an additional layer of security by decentralizing data storage.
- User Consent: Obtaining explicit consent from users before collecting their data fosters trust and ensures compliance with regulations like GDPR. We assist clients in developing user-friendly consent management systems that streamline this process.
- Anonymization: Removing personally identifiable information (PII) from datasets helps protect user identities while still allowing for data analysis. Our AI-driven tools can automate the anonymization process, ensuring compliance without sacrificing data utility.
- Regular Audits: Conducting regular audits of data handling practices can help identify vulnerabilities and ensure compliance with privacy laws. Rapid Innovation offers comprehensive audit services that utilize AI to detect anomalies and potential risks.
- User Control: Providing users with control over their data, including options to delete or modify their information, enhances privacy protection. We can implement blockchain-based solutions that empower users with ownership and control over their data, including our ethical AI development guide.
10.2. Transparency in Personalization

Transparency in personalization refers to the clarity with which organizations communicate how they use personal data to tailor experiences for users. This practice is vital for building trust and ensuring users feel comfortable with the services they engage with. At Rapid Innovation, we help clients establish transparent personalization practices through the following aspects:
- Clear Communication: Organizations should clearly explain how data is collected, used, and shared in their privacy policies. We assist in crafting clear and concise communication strategies that resonate with users.
- Opt-In Options: Allowing users to opt-in to personalized experiences rather than defaulting them into such services promotes transparency. Our solutions can facilitate seamless opt-in processes that enhance user engagement.
- Data Usage Examples: Providing examples of how data influences personalization can help users understand the benefits and implications of data usage. We can develop interactive tools that visually demonstrate data usage in real-time.
- Feedback Mechanisms: Implementing feedback channels allows users to express concerns or preferences regarding their personalized experiences. Rapid Innovation can create AI-driven feedback systems that analyze user input for continuous improvement.
- Regular Updates: Keeping users informed about changes in data usage policies or personalization algorithms fosters ongoing transparency. We help clients establish automated communication systems that keep users updated in a timely manner.
10.3. Bias Prevention and Fairness
Bias prevention and fairness are essential components of ethical data practices, particularly in machine learning and artificial intelligence. Addressing bias ensures that algorithms do not perpetuate discrimination or inequality. At Rapid Innovation, we prioritize fairness in AI development through key measures:
- Diverse Data Sets: Utilizing diverse and representative data sets helps minimize bias in algorithmic decision-making. We guide clients in sourcing and curating diverse datasets to enhance the fairness of their AI models.
- Regular Testing: Conducting regular tests for bias in algorithms can identify and mitigate unfair outcomes before they affect users. Our AI tools can automate bias detection, ensuring ongoing compliance with ethical standards.
- Inclusive Design: Involving diverse teams in the design process can lead to more equitable solutions and reduce the risk of bias. Rapid Innovation promotes inclusive design practices that reflect a variety of perspectives.
- Transparency in Algorithms: Providing insights into how algorithms make decisions can help users understand potential biases and advocate for fairness. We develop explainable AI solutions that clarify decision-making processes for end-users.
- Continuous Monitoring: Implementing ongoing monitoring of algorithms allows organizations to adapt and correct biases as they arise. Our AI-driven monitoring systems provide real-time insights into algorithm performance, enabling proactive adjustments.
By integrating these strategies, Rapid Innovation empowers clients to achieve their business goals efficiently and effectively while ensuring ethical practices in AI and Blockchain development, including a comprehensive information protection strategy and a personal data protection strategy.
10.4. User Control and Consent
User control and consent are fundamental principles in the realm of data privacy and protection. They empower individuals to manage their personal information and dictate how it is used.
- Informed Consent: Users should be fully informed about what data is being collected, how it will be used, and who it will be shared with. This transparency builds trust and ensures that users can make educated decisions regarding their data.
- Opt-in and Opt-out Options: Organizations must provide clear options for users to opt-in or opt-out of data collection. This includes easy-to-understand mechanisms for users to withdraw consent at any time, such as utilizing data privacy compliance software.
- Granular Control: Users should have the ability to control specific aspects of their data. For instance, they might want to allow certain data to be shared while restricting others. This level of granularity enhances user autonomy and can be facilitated by privacy management software.
- Regular Updates: Organizations should regularly update users about changes in data practices. This includes notifying them of any new data collection methods or changes in how their data will be used, which can be managed through a data privacy management software.
- User-Friendly Interfaces: The design of consent forms and privacy settings should be intuitive. Complicated language or convoluted processes can lead to user confusion and unintentional consent. Implementing best data privacy management software can help streamline this process.
- Legal Compliance: Adhering to regulations such as GDPR and CCPA is crucial. These laws mandate that organizations obtain explicit consent from users before collecting or processing their data, which can be supported by GDPR compliance software.
10.5. Ethical Guidelines and Compliance
Ethical guidelines and compliance are essential for maintaining integrity in data handling practices. Organizations must navigate a complex landscape of regulations and ethical considerations to protect user data.
- Adherence to Regulations: Compliance with laws like GDPR, HIPAA, and CCPA is non-negotiable. These regulations set the standards for data protection and user privacy, which can be monitored using GDPR compliance platforms.
- Data Minimization: Organizations should only collect data that is necessary for their operations. This principle of data minimization reduces the risk of data breaches and enhances user trust, which can be supported by data privacy solutions.
- Accountability: Companies must take responsibility for their data practices. This includes having clear policies in place and appointing data protection officers to oversee compliance, often facilitated by privacy compliance solutions.
- Ethical Data Use: Beyond legal compliance, organizations should adopt ethical practices in data usage. This includes avoiding manipulative tactics in data collection and ensuring that data is used for the benefit of users, which can be enhanced through privacy management platforms.
- Training and Awareness: Regular training for employees on ethical data handling practices is vital. This ensures that everyone in the organization understands the importance of data privacy and compliance, which can be supported by privacy compliance software.
- Transparency and Reporting: Organizations should be transparent about their data practices and provide regular reports on data usage and breaches. This openness fosters trust and accountability, which can be enhanced through privacy management software gartner.
11. Challenges and Solutions
The landscape of data privacy and protection is fraught with challenges, but there are effective solutions to address these issues.
- Challenge: Data Breaches: With the increasing amount of data being collected, the risk of data breaches is higher than ever.
- Solution: Implement robust cybersecurity measures, including encryption, firewalls, and regular security audits. Training employees on recognizing phishing attempts can also mitigate risks.
- Challenge: User Awareness: Many users are unaware of their rights regarding data privacy and the extent of data collection.
- Solution: Organizations should invest in user education campaigns that inform individuals about their rights and how to protect their data. Clear communication about privacy policies is essential.
- Challenge: Compliance Complexity: Navigating the myriad of data protection regulations can be overwhelming for organizations.
- Solution: Employ legal experts or consultants specializing in data privacy to ensure compliance with all relevant laws. Regular audits can help identify areas for improvement.
- Challenge: Balancing Innovation and Privacy: Companies often struggle to innovate while respecting user privacy.
- Solution: Adopt a privacy-by-design approach, integrating privacy considerations into the development of new products and services from the outset.
- Challenge: Data Management: Managing large volumes of data can lead to inefficiencies and increased risk of non-compliance.
- Solution: Utilize data management tools and software that streamline data collection, storage, and processing, such as data privacy platforms. Regularly review data retention policies to ensure compliance.
- Challenge: Global Variability in Regulations: Different countries have varying data protection laws, complicating international operations.
- Solution: Develop a comprehensive global data strategy that accounts for regional regulations. This may involve localizing data practices to meet specific legal requirements.
By addressing these challenges with effective solutions, organizations can enhance their data privacy practices, build user trust, and ensure compliance with ethical guidelines.
At Rapid Innovation, we leverage our expertise in AI and Blockchain to help organizations navigate these complexities. Our AI-driven solutions can automate compliance checks and enhance user awareness through personalized education campaigns. Meanwhile, our Blockchain technology ensures data integrity and transparency, allowing users to have greater control over their data. By partnering with us, organizations can achieve greater ROI while maintaining ethical standards and compliance in their data practices, including utilizing tools like liveramp opt out for enhanced user control.
11.1. Technical Challenges
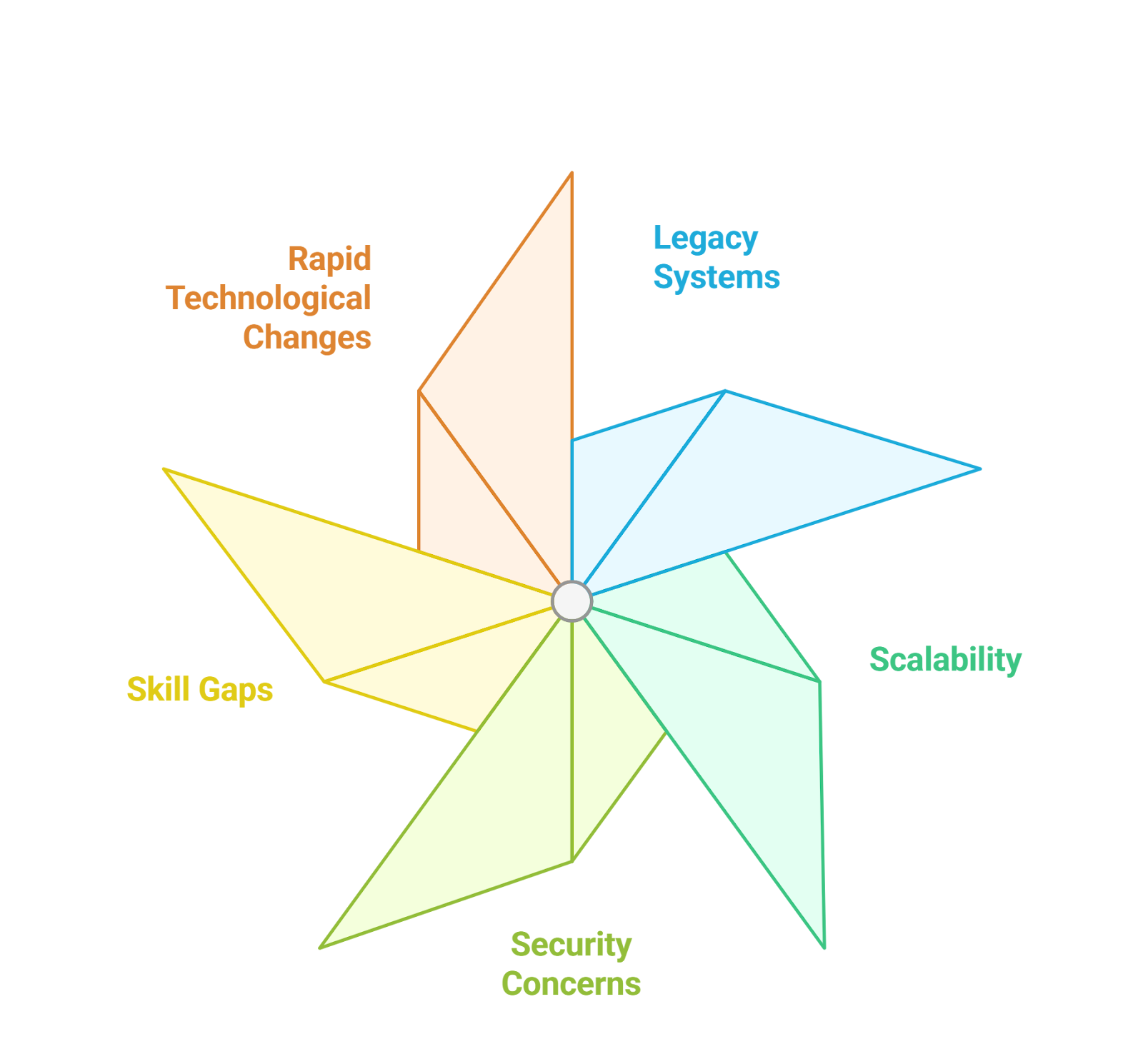
Technical challenges in any project can significantly impact its success. These challenges often arise from the rapid evolution of technology and the need for constant adaptation.
- Legacy Systems: Many organizations still rely on outdated systems that are not compatible with modern technologies, hindering the implementation of new solutions. Rapid Innovation specializes in modernizing these legacy systems, enabling seamless integration with advanced AI and Blockchain technologies, thus enhancing operational efficiency.
- Scalability: As businesses grow, their technical infrastructure must scale accordingly. Ensuring that systems can handle increased loads without performance degradation is crucial. Our team at Rapid Innovation employs scalable AI solutions that adapt to your business needs, ensuring optimal performance even during peak times.
- Security Concerns: With the rise of cyber threats, ensuring data security and compliance with regulations is a major technical challenge. Organizations must invest in robust security measures to protect sensitive information. Rapid Innovation offers Blockchain-based security solutions that provide enhanced data integrity and transparency, safeguarding your business against potential threats.
- Skill Gaps: The fast-paced nature of technology means that there is often a shortage of skilled professionals who can manage and implement new systems effectively. Rapid Innovation provides training and consulting services to bridge these skill gaps, empowering your team to leverage AI and Blockchain technologies effectively.
- Rapid Technological Changes: Keeping up with the latest advancements can be overwhelming. Organizations must continuously train their staff and update their systems to stay competitive. Rapid Innovation stays at the forefront of technological advancements, ensuring that your organization is equipped with the latest tools and knowledge to thrive in a competitive landscape. For insights on future trends, check out our article on AI Knowledge Management in 2024.
11.2. Data Quality Issues
Data quality is essential for making informed decisions. Poor data quality can lead to incorrect conclusions and wasted resources.
- Inaccurate Data: Data can be incorrect due to human error, outdated information, or faulty data entry processes, leading to misguided strategies and decisions. Rapid Innovation implements AI-driven data validation processes that enhance accuracy and reliability, ensuring that your decisions are based on solid data.
- Inconsistent Data: When data is collected from multiple sources, inconsistencies can arise, creating confusion and hindering effective analysis. Our solutions help standardize data collection methods, allowing for coherent and consistent data analysis across your organization.
- Missing Data: Incomplete datasets can skew results and lead to inaccurate insights. Organizations must ensure that they have comprehensive data collection processes in place. Rapid Innovation assists in establishing robust data governance frameworks that minimize data gaps and enhance overall data quality.
- Data Duplication: Duplicate records can inflate data volumes and complicate analysis. Organizations need to implement data cleansing processes to maintain accuracy. Our AI solutions automate data cleansing, ensuring that your datasets are free from duplicates and ready for analysis.
- Lack of Standardization: Without standardized data formats, integrating and analyzing data becomes challenging. Establishing clear data governance policies can help mitigate this issue. Rapid Innovation helps organizations develop and implement standardized data protocols, facilitating smoother integration and analysis.
11.3. Integration Complexities
Integration complexities arise when attempting to connect various systems and data sources. These challenges can hinder operational efficiency and data flow.
- Diverse Systems: Organizations often use a mix of legacy and modern systems, making integration difficult as each system may have different protocols and data formats. Rapid Innovation specializes in creating custom integration solutions that bridge the gap between diverse systems, ensuring seamless data flow.
- Data Silos: When departments operate in isolation, valuable data can become trapped in silos, limiting collaboration and the ability to gain holistic insights. Our solutions promote cross-departmental data sharing, breaking down silos and fostering a collaborative environment.
- API Limitations: Application Programming Interfaces (APIs) are essential for integration, but they can have limitations. Not all systems have robust APIs, complicating data exchange. Rapid Innovation develops custom APIs that enhance interoperability between systems, facilitating smoother data exchange.
- Change Management: Integrating new systems often requires changes in processes and workflows. Resistance to change from employees can slow down integration efforts. Our team provides change management support, helping organizations navigate transitions smoothly and ensuring employee buy-in.
- Testing and Validation: Ensuring that integrated systems work seamlessly requires extensive testing, which can be time-consuming and resource-intensive, delaying project timelines. Rapid Innovation employs automated testing frameworks that streamline the validation process, reducing time and resources spent on testing while ensuring system reliability.
- Technical Challenges in Integration: The integration process itself can present various technical challenges, including compatibility issues between different systems and the need for custom solutions to address unique organizational needs. Rapid Innovation addresses these technical challenges in integration by providing tailored strategies that ensure smooth connectivity and data flow across platforms.
11.4. Scalability Concerns
Scalability is a critical factor for any technology or business model, particularly in the digital landscape. It refers to the ability of a system to handle a growing amount of work or its potential to accommodate growth. In the context of software and technology, scalability concerns can manifest in various ways:
- Infrastructure Limitations: As user demand increases, the underlying infrastructure must be capable of supporting additional loads. This includes server capacity, bandwidth, and database management. If the infrastructure is not scalable, it can lead to slow performance or system crashes.
- Cost Implications: Scaling a system often involves significant financial investment. Businesses must consider the costs associated with upgrading hardware, software, and network capabilities. This can be a barrier for startups or smaller companies with limited budgets.
- Complexity of Scaling: As systems grow, they can become more complex. This complexity can lead to challenges in maintaining performance and reliability. Developers may face difficulties in ensuring that new features integrate seamlessly with existing systems, particularly when considering blockchain scalability solutions.
- Data Management: With increased scalability comes the challenge of managing larger volumes of data. Efficient data storage, retrieval, and processing become paramount. Poor data management can lead to inefficiencies and hinder the user experience, especially in the context of scalability in blockchain.
- User Experience: A scalable system must maintain a high-quality user experience, even as the number of users grows. If the system cannot handle increased traffic, users may experience delays or outages, leading to dissatisfaction and potential loss of customers. This is particularly relevant when discussing the scalability of blockchain, where user experience can be significantly impacted.
At Rapid Innovation, we specialize in developing scalable technology solutions that address these concerns. By leveraging our expertise in AI and blockchain technologies, we help businesses design robust infrastructures that can grow alongside their user base, ensuring optimal performance and user satisfaction. Our consulting services guide clients through the complexities of scaling, allowing them to focus on their core business objectives while we handle the technical intricacies.
11.5. User Adoption Barriers
User adoption is crucial for the success of any new technology or platform. However, several barriers can hinder users from fully embracing new solutions:
- Lack of Awareness: Many potential users may not be aware of the benefits or existence of a new technology. Effective marketing and education are essential to inform users about how the technology can solve their problems.
- Resistance to Change: Users often resist changing from familiar systems to new ones. This resistance can stem from comfort with existing processes or fear of the unknown. Overcoming this barrier requires demonstrating the value and ease of the new solution.
- Usability Issues: If a new technology is not user-friendly, it can deter adoption. Complicated interfaces or steep learning curves can frustrate users. Ensuring that the technology is intuitive and easy to navigate is vital for encouraging adoption.
- Integration Challenges: Users may be hesitant to adopt new technology if it does not integrate well with their existing systems. Compatibility issues can create additional work and complexity, making users reluctant to switch.
- Cost Concerns: The financial implications of adopting new technology can be a significant barrier. Users may be concerned about upfront costs, ongoing fees, or the potential for hidden expenses. Providing clear pricing structures and demonstrating return on investment can help alleviate these concerns.
- Security and Privacy Concerns: Users are increasingly aware of data security and privacy issues. If a new technology does not adequately address these concerns, users may be hesitant to adopt it. Transparency about security measures and compliance with regulations is essential.
At Rapid Innovation, we understand these barriers and work closely with our clients to develop comprehensive strategies that promote user adoption. By focusing on education, user-friendly design, and clear communication about the benefits and security of our AI and blockchain solutions, we empower businesses to overcome these challenges and achieve greater ROI.
12. Future Trends and Innovation

The landscape of technology is constantly evolving, and staying ahead of future trends is essential for businesses and developers. Here are some key trends and innovations to watch:
- Artificial Intelligence (AI) and Machine Learning (ML): AI and ML are transforming industries by enabling automation, predictive analytics, and personalized experiences. Businesses are increasingly leveraging these technologies to enhance decision-making and improve customer interactions.
- Blockchain Technology: Beyond cryptocurrencies, blockchain is being explored for various applications, including supply chain management, secure transactions, and identity verification. Its decentralized nature offers enhanced security and transparency. However, the scalability blockchain presents remains a challenge that needs addressing.
- Internet of Things (IoT): The proliferation of connected devices is creating new opportunities for data collection and analysis. IoT is driving innovations in smart homes, healthcare, and industrial automation, leading to increased efficiency and improved user experiences.
- 5G Technology: The rollout of 5G networks is set to revolutionize connectivity. With faster speeds and lower latency, 5G will enable advancements in areas such as augmented reality (AR), virtual reality (VR), and real-time data processing.
- Sustainability and Green Technology: As environmental concerns grow, there is a push for sustainable practices and technologies. Innovations in renewable energy, waste reduction, and eco-friendly materials are becoming increasingly important for businesses.
- Remote Work Solutions: The shift to remote work has accelerated the development of tools and technologies that facilitate collaboration and productivity. Innovations in virtual communication, project management, and cybersecurity are essential for supporting remote teams.
- Augmented Reality (AR) and Virtual Reality (VR): These technologies are gaining traction in various sectors, including education, training, and entertainment. They offer immersive experiences that can enhance learning and engagement.
Staying informed about these trends and innovations will help businesses adapt and thrive in an ever-changing technological landscape. At Rapid Innovation, we are committed to guiding our clients through these advancements, ensuring they harness the full potential of AI and blockchain technologies to achieve their business goals efficiently and effectively.
12.1. Emerging Technologies
Emerging technologies are reshaping industries and redefining how businesses operate. These innovations are characterized by their potential to disrupt existing markets and create new opportunities. Key emerging technologies include:
- Artificial Intelligence (AI) and Machine Learning (ML): These technologies enable systems to learn from data, improving decision-making and automating processes. At Rapid Innovation, we leverage AI and ML to help clients enhance operational efficiency and accuracy across various sectors, from healthcare to finance. For instance, we have developed predictive analytics solutions that allow businesses to anticipate market trends, leading to greater ROI. For more information, check out our ultimate guide to AI platforms.
- Blockchain: Originally developed for cryptocurrencies, blockchain technology is now being utilized for secure transactions, supply chain management, and data integrity. Rapid Innovation harnesses the decentralized nature of blockchain to ensure transparency and reduce fraud for our clients. By implementing blockchain solutions, we have helped businesses streamline their operations and build trust with their customers.
- Internet of Things (IoT): IoT connects everyday devices to the internet, allowing for real-time data collection and analysis. Rapid Innovation assists clients in adopting IoT technologies to transform industries like agriculture, manufacturing, and smart homes. Our IoT solutions enable better resource management, resulting in significant cost savings and improved productivity.
- Augmented Reality (AR) and Virtual Reality (VR): These technologies are enhancing user experiences in gaming, education, and training by providing immersive environments that can simulate real-world scenarios. Rapid Innovation develops AR and VR applications that help businesses engage customers in innovative ways, driving higher engagement and conversion rates.
- 5G Technology: The rollout of 5G networks is set to revolutionize connectivity, offering faster data speeds and lower latency. Rapid Innovation is at the forefront of this transformation, helping clients leverage 5G to enable advancements in IoT, autonomous vehicles, and smart cities. Our expertise ensures that businesses can capitalize on the benefits of 5G technology for enhanced operational efficiency.
The impact of these technologies is profound, leading to increased productivity, improved customer experiences, and innovative business models. Companies that adopt and integrate these technologies, including new technology, new advanced technology, and emerging technologies in healthcare, with the help of Rapid Innovation are likely to gain a competitive edge in their respective markets.
12.2. Next-Generation Personalization
Next-generation personalization refers to the advanced techniques and strategies used to tailor products, services, and experiences to individual customer preferences. This approach goes beyond traditional personalization methods by leveraging data analytics and AI. Key aspects include:
- Data-Driven Insights: Businesses are utilizing big data to understand customer behavior and preferences. Rapid Innovation helps clients analyze data from various sources to create detailed customer profiles that inform personalized marketing strategies, ultimately leading to increased sales and customer loyalty.
- Predictive Analytics: This involves using historical data to predict future customer behavior. By anticipating needs and preferences, businesses can offer timely and relevant recommendations. Rapid Innovation's predictive analytics solutions empower clients to enhance customer satisfaction and drive revenue growth.
- Dynamic Content Delivery: Next-generation personalization allows for real-time content adjustments based on user interactions. Rapid Innovation develops websites and applications that can display tailored content, offers, and recommendations, improving engagement and conversion rates for our clients.
- Omnichannel Experiences: Customers expect a seamless experience across all touchpoints. Rapid Innovation ensures that interactions are consistent and tailored, whether online, in-store, or through mobile apps, helping businesses meet and exceed customer expectations.
- Ethical Considerations: As personalization becomes more sophisticated, ethical concerns regarding data privacy and consent arise. Rapid Innovation guides businesses in navigating these challenges while providing personalized experiences that respect customer privacy.
By implementing next-generation personalization strategies with the expertise of Rapid Innovation, companies can foster stronger customer relationships, increase loyalty, and drive sales growth.
12.3. Integration with Emerging Platforms
The integration of emerging technologies with new platforms is crucial for businesses looking to stay competitive in a rapidly evolving landscape. This integration involves combining various technologies and platforms to create cohesive systems that enhance functionality and user experience. Key points include:
- Cloud Computing: The adoption of cloud platforms allows businesses to scale operations, store data securely, and access advanced tools without significant upfront investment. Rapid Innovation facilitates integration with cloud services, enabling seamless collaboration and data sharing for our clients.
- API Ecosystems: Application Programming Interfaces (APIs) facilitate communication between different software applications. By integrating APIs, Rapid Innovation enhances client platforms with third-party services, improving functionality and user experience.
- Cross-Platform Compatibility: As users engage with multiple devices and platforms, ensuring compatibility is essential. Rapid Innovation develops solutions that work seamlessly across desktops, mobile devices, and other platforms to meet customer expectations.
- Data Integration: Combining data from various sources provides a comprehensive view of customer interactions. Rapid Innovation's data integration solutions allow for better analytics, informed decision-making, and improved personalization efforts.
- Emerging Social Media Platforms: With the rise of new social media platforms, businesses must adapt their marketing strategies to engage users effectively. Rapid Innovation helps clients integrate these platforms into existing marketing efforts, enhancing brand visibility and customer engagement.
By focusing on the integration of emerging technologies with new platforms, including new technology trends and latest technologies, Rapid Innovation enables businesses to create innovative solutions that drive growth, improve customer experiences, and maintain a competitive edge in their industries.
12.4. Future of Customer Experience
The future of customer experience (CX) is poised for significant transformation, driven by technological advancements and evolving consumer expectations. Businesses are increasingly recognizing that delivering exceptional customer experiences is crucial for maintaining competitive advantage.
- Personalization will become more sophisticated: Companies will leverage data analytics and artificial intelligence (AI) to create highly personalized experiences. Tailored recommendations and customized communications will enhance customer engagement, allowing businesses to connect with their audience on a deeper level. This aligns with customer experience trends and customer experience technology trends that emphasize the importance of personalization.
- Omnichannel experiences will be the norm: Customers will expect seamless interactions across various channels, including online, mobile, and in-store. Businesses will need to integrate their platforms to provide a cohesive experience, ensuring that customers can transition effortlessly between different touchpoints. This is a key aspect of customer engagement trends and digital customer experience trends.
- Automation and AI will play a pivotal role: Chatbots and virtual assistants will handle routine inquiries, allowing human agents to focus on complex issues. Predictive analytics will help anticipate customer needs and preferences, enabling businesses to proactively address concerns and enhance satisfaction. This reflects the ongoing customer service trends and cx trends that highlight the role of technology in improving service delivery.
- Enhanced focus on emotional connection: Brands will strive to build emotional relationships with customers, recognizing that emotional engagement drives loyalty. Storytelling and authentic brand narratives will be key strategies, fostering a sense of community and belonging among consumers. This trend is increasingly recognized in consumer experience trends and customer experience management trends.
- Sustainability and social responsibility will influence choices: Consumers are increasingly prioritizing brands that demonstrate commitment to sustainability and ethical practices. Companies will need to align their values with those of their customers to foster loyalty, integrating responsible practices into their core business strategies. This is particularly relevant in the context of insurance customer experience trends and b2b customer experience trends.
- Real-time feedback and adaptation: Businesses will implement systems to gather real-time feedback, allowing for immediate adjustments to improve customer satisfaction. Continuous improvement will become a core aspect of customer experience strategies, ensuring that businesses remain responsive to changing consumer needs. This is essential for staying ahead in cx trends 2023 and customer experience trends 2023.
12.5. Research Directions
As the landscape of customer experience continues to evolve, several research directions are emerging that can provide valuable insights for businesses and scholars alike.
- Impact of technology on customer experience: Investigating how emerging technologies, such as augmented reality (AR) and virtual reality (VR), can enhance customer interactions. Exploring the role of AI in personalizing customer journeys and improving service efficiency, which is crucial in understanding cx trends 2022 and total experience gartner.
- Understanding customer behavior: Analyzing how different demographics respond to various customer experience strategies. Studying the psychological factors that influence customer satisfaction and loyalty, which is vital for consumer experience trends.
- Measuring customer experience effectiveness: Developing new metrics and frameworks to assess the impact of customer experience initiatives on business performance. Exploring the relationship between customer experience and key performance indicators (KPIs) such as customer retention and revenue growth, as highlighted in customer experience industry trends.
- Cross-industry comparisons: Conducting comparative studies across different industries to identify best practices in customer experience management. Understanding how industry-specific factors influence customer expectations and experiences, which is important for customer experience trends 2022 mckinsey.
- The role of social media in shaping customer experience: Examining how social media platforms affect customer perceptions and interactions with brands. Investigating the impact of user-generated content on brand reputation and customer trust, which is increasingly relevant in cx trends zendesk.
- Ethical considerations in customer experience: Exploring the ethical implications of data collection and personalization in customer experience strategies. Understanding consumer attitudes towards privacy and data security in the context of personalized marketing, which is a growing concern in customer service trends 2023.
By focusing on these research directions, businesses can better navigate the complexities of customer experience and develop strategies that resonate with their target audiences. At Rapid Innovation, we harness the power of AI and blockchain technologies to help our clients implement these strategies effectively, ensuring they achieve greater ROI and maintain a competitive edge in their respective markets. For more information on how we can assist with AI retail and e-commerce solutions, visit our AI for Customer Service in 2024 and our AI retail e-commerce solutions.