Table Of Contents
Category
Artificial Intelligence
Blockchain
IoT
AIML
1. Introduction to AI-Driven Premium Calculation
AI-driven premium calculation is revolutionizing the insurance industry by leveraging advanced algorithms and machine learning techniques to assess risk and determine insurance premiums more accurately. This technology enables insurers to analyze vast amounts of data quickly, leading to more personalized and fair pricing for policyholders.
AI agents can process data from various sources, including: - Historical claims data - Customer demographics - Behavioral data - External factors like weather and economic conditions
The integration of AI in premium calculation offers several advantages: - Enhanced accuracy in risk assessment - Improved customer experience through personalized pricing - Increased efficiency in underwriting processes
At Rapid Innovation, we specialize in implementing AI-driven premium calculation solutions that optimize premium calculation processes for our clients. By harnessing the power of AI, we help insurance companies achieve greater ROI through improved risk assessment and customer satisfaction.
However, the adoption of AI in premium calculation also presents challenges: - Data privacy concerns - The need for transparency in AI decision-making - Potential biases in algorithms that could affect pricing fairness
As the insurance landscape continues to evolve, understanding the role of AI-driven premium calculation is crucial for both insurers and consumers. This technology not only streamlines operations but also fosters a more equitable insurance market. Rapid Innovation is committed to guiding our clients through these complexities, ensuring they leverage AI effectively while addressing potential challenges.
Refer to the image for a visual representation of AI-driven premium calculation in the insurance industry:
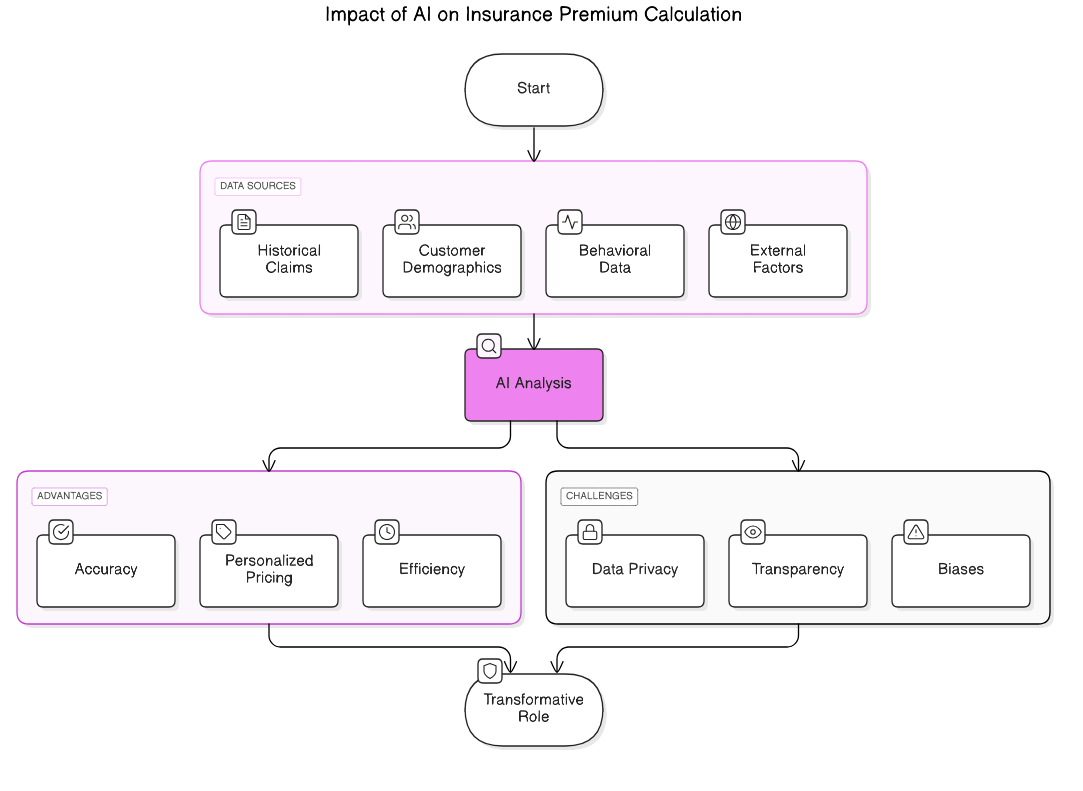
1.1. Defining AI Agents in Insurance
AI agents in the insurance sector refer to intelligent systems that utilize artificial intelligence to perform tasks traditionally handled by human agents, such as those provided by ai insurance agency and a & i insurance agency. These systems can analyze vast amounts of data, learn from patterns, and make decisions based on predictive analytics.
- AI agents can automate customer service, underwriting, and claims processing.
- They enhance operational efficiency by reducing human error and speeding up processes.
- These agents can provide personalized insurance solutions by analyzing individual customer data.
- They utilize machine learning algorithms to improve their performance over time.
- AI agents can predict risks and assess claims more accurately, leading to better risk management.
The integration of AI agents in insurance, including ai agents in insurance, is transforming how companies interact with customers and manage their operations, making them a crucial component of modern insurance practices. At Rapid Innovation, we leverage our expertise in AI to help insurance firms implement these agents, resulting in increased efficiency and a higher return on investment (ROI). For more insights on how AI is reshaping pricing strategies, check out how artificial intelligence is reshaping price optimization.
1.2. Evolution of Premium Pricing Methodologies
Premium pricing methodologies in insurance have evolved significantly over the years, driven by advancements in technology and data analytics. Traditionally, insurers relied on historical data and actuarial science to set premiums. However, the landscape has changed with the introduction of more sophisticated techniques.
- Early methodologies focused on broad categories and generalized risk assessments.
- The introduction of data analytics allowed for more granular risk evaluation, leading to more tailored premiums.
- The rise of telematics and IoT devices has enabled real-time data collection, allowing insurers to adjust premiums based on actual behavior.
- Predictive modeling and machine learning have further refined pricing strategies, enabling insurers to forecast risks more accurately.
- Insurers are now adopting dynamic pricing models that can change based on market conditions and individual customer profiles.
This evolution reflects a shift towards more personalized and data-driven approaches in premium pricing, enhancing competitiveness and customer satisfaction. Rapid Innovation assists clients in adopting these advanced methodologies, ensuring they remain competitive and maximize their ROI.
1.3. Paradigm Shift: From Traditional to Intelligent Pricing
The shift from traditional to intelligent pricing in insurance represents a significant transformation in how premiums are determined and adjusted. Traditional pricing methods often relied on static data and broad assumptions, while intelligent pricing leverages advanced technologies and real-time data.
- Intelligent pricing utilizes AI and machine learning to analyze vast datasets, leading to more accurate risk assessments.
- It allows for dynamic pricing, where premiums can be adjusted in real-time based on changing risk factors.
- Insurers can now offer personalized premiums that reflect individual customer behavior and preferences.
- The use of big data analytics enables insurers to identify trends and patterns that were previously undetectable.
- Intelligent pricing enhances customer engagement by providing transparent and fair pricing models.
This paradigm shift not only improves the accuracy of premium pricing but also fosters a more customer-centric approach in the insurance industry, ultimately leading to better customer retention and satisfaction. At Rapid Innovation, we guide our clients through this transition, ensuring they harness the full potential of intelligent pricing strategies to achieve greater ROI.
Refer to the image for a visual representation of AI agents in the insurance sector and their impact on premium pricing methodologies.
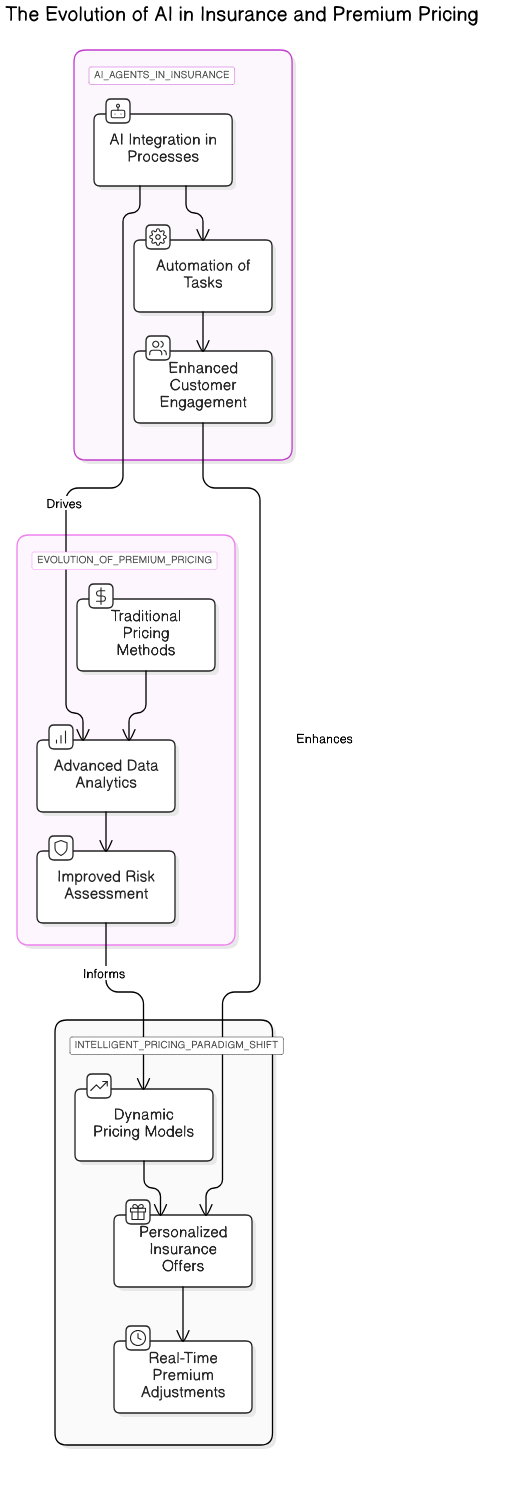
1.4. Key Challenges in Traditional Premium Calculation
Traditional premium calculation methods in insurance face several key challenges that can impact accuracy and efficiency. These challenges include:
- Data Quality and Availability: Insurers often rely on historical data, which may be incomplete or outdated. Inconsistent data formats can lead to errors in calculations, particularly when calculating car insurance rates or life insurance costs.
- Complex Risk Assessment: Accurately assessing risk is complicated by numerous variables, including economic conditions and individual behaviors. Traditional models may not account for emerging risks, such as cyber threats or climate change, which can affect the cost of health insurance and term life insurance calculators.
- Regulatory Compliance: Insurers must navigate a complex landscape of regulations that can vary by region. Compliance requirements can change frequently, necessitating constant updates to premium calculation methods, including life insurance premium calculators and whole life insurance policy calculators.
- Limited Predictive Power: Traditional actuarial methods often rely on linear models that may not capture the complexities of risk. This can lead to mispricing of premiums, resulting in financial losses for insurers, especially when using term insurance cost calculators or long-term care insurance cost estimates.
- Inefficiency in Processes: Manual calculations and legacy systems can slow down the premium calculation process. This inefficiency can lead to delays in policy issuance and customer dissatisfaction, particularly when using life policy premium calculators or health insurance payment calculators.
- Market Competition: Insurers face pressure to offer competitive pricing while maintaining profitability. Traditional methods may not provide the agility needed to respond to market changes quickly, impacting the effectiveness of tools like the premium tax credit calculator or obamacare cost per month calculator.
2. Foundational Technologies

The insurance industry is increasingly leveraging foundational technologies to enhance operations, improve customer experiences, and streamline processes. Key technologies include:
- Data Analytics: Advanced data analytics allows insurers to analyze vast amounts of data for better decision-making. Predictive analytics can help in identifying trends and potential risks, which is crucial for calculating life insurance premium estimates and whole life insurance premium calculators.
- Cloud Computing: Cloud technology enables insurers to store and process large datasets efficiently. It offers scalability and flexibility, allowing for rapid deployment of new applications, including long-term insurance calculators and term insurance calculators.
- Blockchain: Blockchain technology can enhance transparency and security in transactions. It can streamline claims processing and reduce fraud, providing a secure and immutable record of transactions that can be accessed by all parties involved.
- Internet of Things (IoT): IoT devices provide real-time data that can be used for risk assessment and premium calculation. This technology can lead to personalized insurance products based on individual behaviors, allowing insurers to tailor offerings to specific customer needs, such as those calculated through a whole life policy premium calculator.
- Artificial Intelligence (AI): AI can automate routine tasks, improving efficiency and reducing operational costs. Machine learning algorithms can enhance risk assessment and fraud detection, enabling insurers to make data-driven decisions that lead to greater ROI.
2.1. Machine Learning Fundamentals
Machine learning (ML) is a subset of artificial intelligence that focuses on the development of algorithms that allow computers to learn from and make predictions based on data. Understanding the fundamentals of machine learning is crucial for its application in various fields, including insurance. Key concepts include:
- Supervised Learning: Involves training a model on labeled data, where the outcome is known. Common algorithms include linear regression, decision trees, and support vector machines, which can be applied in calculating insurance rates.
- Unsupervised Learning: Involves training a model on data without labeled outcomes. Techniques like clustering and dimensionality reduction are used to identify patterns, which can be beneficial in developing tools like the term life insurance calculator.
- Reinforcement Learning: A type of learning where an agent learns to make decisions by receiving rewards or penalties. This approach is useful in dynamic environments where the model must adapt to changing conditions.
- Feature Engineering: The process of selecting and transforming variables to improve model performance. Effective feature engineering can significantly enhance the accuracy of predictions, particularly in applications like life insurance policy calculators.
- Model Evaluation: Assessing the performance of a machine learning model using metrics such as accuracy, precision, and recall. Cross-validation techniques help ensure that the model generalizes well to unseen data.
- Overfitting and Underfitting: Overfitting occurs when a model learns noise in the training data, leading to poor performance on new data. Underfitting happens when a model is too simple to capture the underlying patterns in the data.
- Applications in Insurance: Machine learning can be used for risk assessment, fraud detection, and customer segmentation. It enables insurers to create personalized products and improve customer service through predictive analytics, ultimately leading to enhanced profitability and customer satisfaction.
At Rapid Innovation, we leverage these foundational technologies, including AI and blockchain, to help our clients overcome the challenges in traditional premium calculation. By implementing advanced data analytics and machine learning, we empower insurers to make informed decisions, optimize their pricing strategies, and enhance operational efficiency, resulting in greater ROI and a competitive edge in the market.
Refer to the image for a visual representation of the key challenges in traditional premium calculation in insurance.
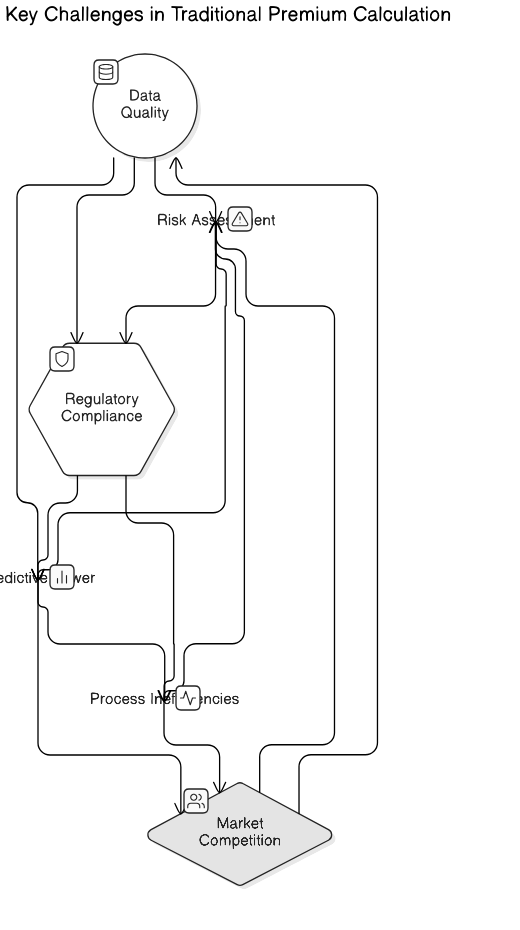
2.2. Predictive Analytics
Predictive analytics is a branch of advanced analytics that uses historical data, machine learning, and statistical algorithms to forecast future outcomes. It plays a crucial role in various industries, helping organizations make informed decisions based on data-driven insights.
- Utilizes historical data to identify patterns and trends.
- Employs machine learning algorithms to enhance accuracy.
- Commonly used in finance, healthcare, marketing, and supply chain management.
- Helps in risk assessment, customer segmentation, and demand forecasting.
- Tools like
R
,Python
, andSAS
are often used for predictive modeling.
The effectiveness of predictive analytics can be seen in its ability to improve operational efficiency and customer satisfaction. For instance, businesses can predict customer behavior, allowing them to tailor marketing strategies accordingly. According to a report by McKinsey, companies that leverage predictive analytics can increase their profitability by up to 15%. At Rapid Innovation, we harness the power of predictive analytics, including predictive data analytics and predictive modeling, to help our clients optimize their operations and enhance decision-making processes, ultimately driving greater ROI. We also utilize tools like Power BI predictive analytics and Azure predictive analytics to provide comprehensive solutions. In healthcare, predictive analytics in healthcare and predictive healthcare models are transforming patient care and operational efficiency. For more information on using R for predictive analytics, visit R Programming for Data Science.
2.3. Data Science Techniques
Data science encompasses a variety of techniques and methodologies that enable the extraction of insights from structured and unstructured data. It combines elements from statistics, computer science, and domain expertise to analyze complex data sets.
- Involves data cleaning, preparation, and exploration.
- Techniques include machine learning, data mining, and natural language processing (NLP).
- Data visualization tools like
Tableau
andPower BI
help in presenting findings. - Emphasizes the importance of domain knowledge for effective analysis.
- Collaboration between data scientists, analysts, and business stakeholders is essential.
Data science techniques are pivotal in driving innovation and efficiency across sectors. For example, in retail, data science can optimize inventory management and enhance customer experience through personalized recommendations. At Rapid Innovation, we implement advanced data science methodologies to empower our clients to make data-driven decisions that lead to improved performance and increased profitability. According to a study by IBM, the demand for data scientists is expected to grow by 28% by 2020. The integration of predictive analytics softwares and tools like Alteryx predictive analytics further enhances our capabilities in this domain.
2.4. Statistical Modeling
Statistical modeling is a mathematical approach that uses statistical methods to represent complex data relationships. It is fundamental in understanding data distributions and making predictions based on observed data.
- Involves creating mathematical models to describe data patterns.
- Common techniques include linear regression, logistic regression, and time series analysis.
- Helps in hypothesis testing and estimating relationships between variables.
- Provides a framework for making inferences about a population based on sample data.
- Software tools like
R
,Python
, andSPSS
are widely used for statistical modeling.
Statistical modeling is essential for decision-making in various fields, including economics, healthcare, and social sciences. It allows researchers and analysts to quantify uncertainty and assess the impact of different variables. For instance, in healthcare, statistical models can predict patient outcomes based on treatment options, leading to better clinical decisions. At Rapid Innovation, we leverage statistical modeling to provide our clients with actionable insights that enhance their strategic initiatives. According to the American Statistical Association, statistical modeling is crucial for evidence-based policy-making. The application of prescriptive analytics and prescriptive analysis can further refine our approach to decision-making processes.
Refer to the image below for a visual representation related to Predictive Analytics.

2.5. Neural Network Architectures
Neural network architectures are the backbone of many machine learning applications, particularly in deep learning. These architectures consist of interconnected nodes or neurons that mimic the way the human brain processes information. Different architectures are designed to handle various types of data and tasks.
- Feedforward Neural Networks (FNNs): The simplest type of neural network where information moves in one direction—from input to output. They are commonly used for tasks like classification and regression.
- Convolutional Neural Networks (CNNs): Primarily used for image processing and computer vision, CNNs employ convolutional layers to automatically detect features in images. They are effective in tasks such as image recognition and object detection. Variants of CNNs include architectures like AlexNet, SqueezeNet, and very deep convolutional networks for large-scale image recognition.
- Recurrent Neural Networks (RNNs): Designed for sequential data, such as time series or natural language, RNNs are capable of retaining information from previous inputs through loops. They are useful in applications like language modeling and speech recognition. LSTM networks are a specific type of RNN architecture that addresses the vanishing gradient problem.
- Long Short-Term Memory Networks (LSTMs): A type of RNN that maintains long-term dependencies, making them suitable for complex sequences. They are widely used in natural language processing and time series forecasting. The LSTM architecture is particularly effective for tasks requiring memory of previous inputs.
- Generative Adversarial Networks (GANs): Comprising two networks—a generator and a discriminator—that compete against each other, GANs are used for generating realistic data, such as images or text. Applications include image synthesis and data augmentation.
- Transformers: A newer architecture that has revolutionized NLP tasks, transformers utilize self-attention mechanisms to process data in parallel. They power models like BERT and GPT, which excel in understanding context and semantics.
- Residual Networks (ResNets): These architectures introduce skip connections to allow gradients to flow through the network more effectively, enabling the training of very deep networks.
Understanding these architectures is crucial for selecting the right model for specific tasks, especially in fields like computer vision and natural language processing. At Rapid Innovation, we leverage these architectures, including neural architecture search techniques, to develop tailored AI solutions that align with our clients' business objectives, ensuring they achieve greater ROI through efficient and effective implementations.
2.6. Natural Language Processing
Natural Language Processing (NLP) is a subfield of artificial intelligence that focuses on the interaction between computers and human language. It enables machines to understand, interpret, and generate human language in a valuable way.
- Text Analysis: Involves extracting meaningful information from text using techniques such as sentiment analysis, topic modeling, and keyword extraction. This is useful in applications like customer feedback analysis and market research.
- Machine Translation: The process of translating text from one language to another using algorithms. Models like Google Translate utilize deep learning for improved accuracy, which is essential for global communication and content localization.
- Chatbots and Virtual Assistants: NLP powers conversational agents that can understand and respond to user queries. Applications include customer service, personal assistants, and information retrieval, enhancing user experience by providing instant responses.
- Speech Recognition: Converts spoken language into text using NLP techniques. This technology is used in applications like voice-activated assistants and transcription services, and it continues to improve with advancements in deep learning.
- Text Generation: Involves creating coherent and contextually relevant text. Models like GPT-3 can generate human-like text for various applications, which is useful in content creation, summarization, and creative writing.
- Named Entity Recognition (NER): Identifies and classifies key entities in text, such as names, dates, and locations. This is important for information extraction and data organization, enhancing search capabilities and data analysis.
NLP is transforming how we interact with technology, making it more intuitive and accessible. Rapid Innovation harnesses the power of NLP to create solutions that enhance customer engagement and streamline operations for our clients.
3. Use Cases Across Insurance Domains
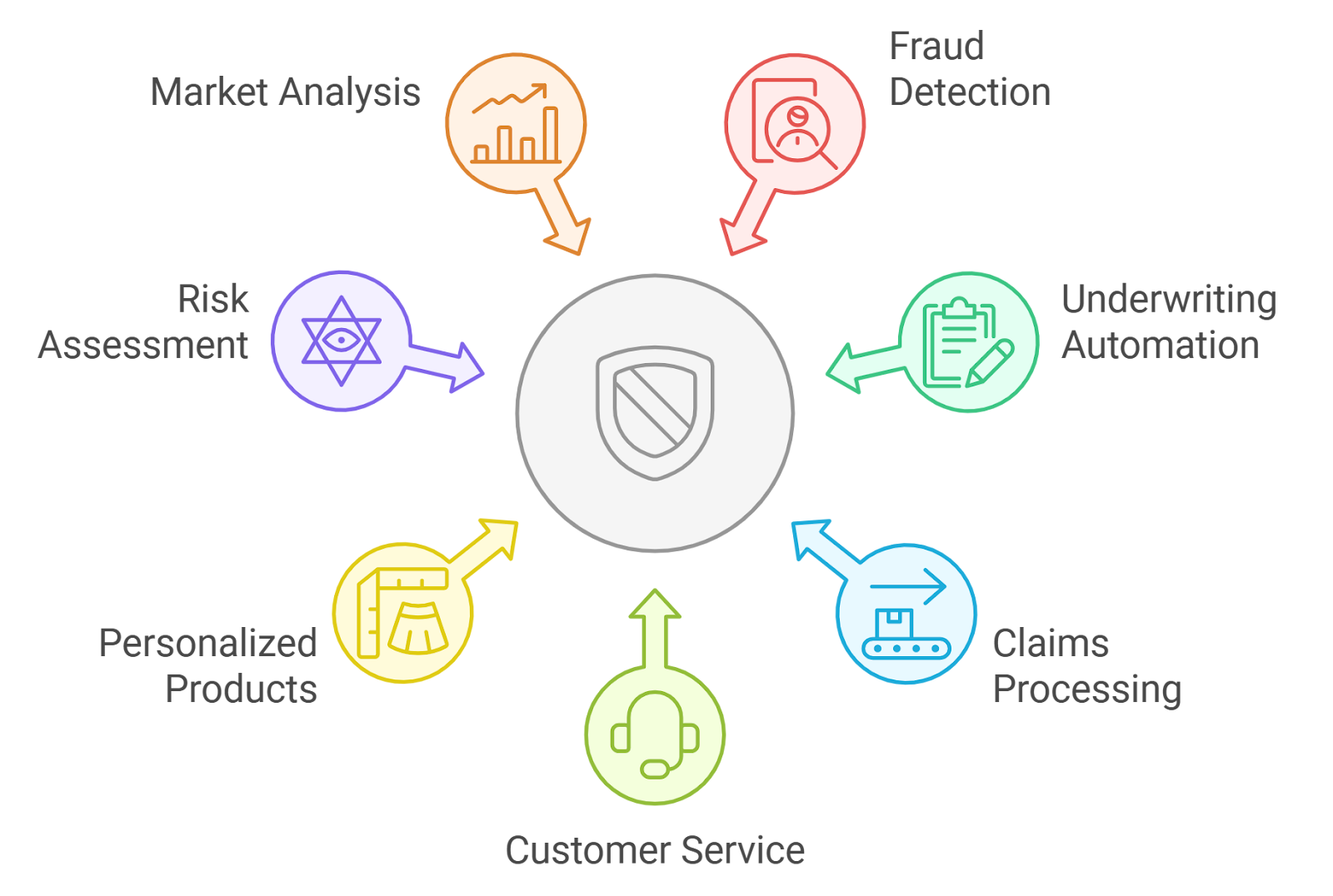
The insurance industry is increasingly leveraging technology, particularly artificial intelligence and machine learning, to enhance operations and customer experiences. Here are some notable use cases across various insurance domains:
- Fraud Detection: AI algorithms analyze patterns in claims data to identify anomalies, helping to reduce fraudulent claims and minimize losses. Insurers can save millions by implementing effective fraud detection systems.
- Underwriting Automation: Machine learning models assess risk factors more accurately and quickly, automating the underwriting process and reducing time and costs. This enhances decision-making by providing data-driven insights.
- Claims Processing: AI streamlines the claims process by automating data entry and analysis, which reduces processing time and improves customer satisfaction. Insurers can handle higher volumes of claims efficiently.
- Customer Service Enhancement: Chatbots and virtual assistants provide 24/7 support to policyholders. NLP enables these systems to understand and respond to inquiries effectively, improving customer engagement and retention.
- Personalized Insurance Products: Data analytics allows insurers to tailor products to individual customer needs. Usage-based insurance models adjust premiums based on actual behavior, enhancing customer satisfaction by offering relevant coverage options.
- Risk Assessment and Management: Predictive analytics helps insurers identify potential risks before they materialize, enabling proactive measures to mitigate risks and reduce claims. This supports better pricing strategies and policy development.
- Market Analysis and Competitive Intelligence: AI tools analyze market trends and competitor strategies, providing insights for strategic planning and product development. This helps insurers stay ahead in a competitive landscape.
These use cases illustrate how technology is reshaping the insurance industry, driving efficiency, and improving customer experiences. At Rapid Innovation, we are committed to delivering innovative AI and blockchain solutions that empower our clients to achieve their business goals effectively and efficiently.
3.1. Life Insurance Premium Calculation
Life insurance premium calculation is a critical process that determines how much a policyholder will pay for their coverage. The premium is influenced by various factors, including the insured's age, health, lifestyle, and the type of policy chosen. Understanding how these elements interact can help individuals make informed decisions about their life insurance needs.
- Premiums are typically calculated using actuarial science, which involves statistical analysis of mortality rates and life expectancy.
- Insurers assess risk factors to determine the likelihood of a claim being made.
- The higher the risk, the higher the premium, as the insurer needs to cover potential payouts.
At Rapid Innovation, we leverage advanced AI algorithms to enhance the accuracy of premium calculations. By analyzing vast datasets, our AI solutions can identify patterns and correlations that traditional methods may overlook, leading to more precise risk assessments and ultimately, better pricing strategies for insurers. Tools such as the term life insurance calculator and life insurance premium estimator can assist in this process.
3.1.1. Health Risk Assessment
Health risk assessment is a vital component in calculating life insurance premiums. Insurers evaluate an applicant's health status to gauge their risk level. This assessment can include:
- Medical history: Insurers review past illnesses, surgeries, and chronic conditions.
- Current health: A medical examination may be required to assess the applicant's current health status.
- Lifestyle choices: Factors such as smoking, alcohol consumption, and exercise habits are considered.
The results of the health risk assessment can significantly impact the premium amount. For instance, individuals with pre-existing conditions or unhealthy lifestyles may face higher premiums due to the increased risk of mortality. Conversely, those in good health may qualify for lower rates, which can be calculated using a life insurance policy calculator.
Rapid Innovation's AI-driven health analytics tools can streamline this assessment process, providing insurers with real-time insights into applicants' health profiles. This not only enhances the underwriting process but also improves customer satisfaction by offering tailored policy options, including options from the whole life insurance policy calculator.
3.1.2. Longevity Prediction
Longevity prediction plays a crucial role in life insurance premium calculation. Insurers use statistical models to estimate how long an individual is likely to live based on various factors. Key elements influencing longevity prediction include:
- Age: Younger individuals generally have lower premiums because they are statistically less likely to pass away soon.
- Gender: Women tend to live longer than men, which can result in lower premiums for female applicants.
- Family history: A family history of longevity or chronic diseases can influence predictions about an individual's lifespan.
By accurately predicting longevity, insurers can set premiums that reflect the risk of payout. This ensures that the insurance company remains financially viable while providing coverage to policyholders. Tools like the term insurance cost calculator and whole life insurance premium calculator can aid in this assessment.
Incorporating blockchain technology, Rapid Innovation can enhance the transparency and security of the data used in longevity predictions. By creating immutable records of health data and risk assessments, insurers can build trust with policyholders while ensuring compliance with regulatory standards. This not only improves operational efficiency but also contributes to a greater return on investment for our clients, utilizing resources such as the life insurance online calculator and term life insurance premium calculator.
3.1.3. Lifestyle Factor Analysis
Lifestyle factor analysis involves examining various aspects of an individual's lifestyle to understand their impact on overall well-being and risk assessment. This analysis is crucial in sectors like health, insurance, and finance, where understanding a person's lifestyle can lead to better decision-making and tailored services.
- Health and Wellness: Lifestyle choices such as diet, exercise, and sleep patterns significantly affect health. For instance, individuals who maintain a balanced diet and regular exercise are less likely to develop chronic diseases.
- Social Habits: Social interactions and relationships can influence mental health. Engaging in community activities or maintaining strong social ties can lead to improved emotional well-being.
- Work-Life Balance: A balanced lifestyle that prioritizes both work and personal time can reduce stress and enhance productivity. Overworking can lead to burnout, affecting both mental and physical health.
- Financial Habits: Spending and saving behaviors are also part of lifestyle analysis. Individuals who budget effectively and save regularly are more likely to achieve financial stability.
- Risk Behaviors: Engaging in risky behaviors, such as smoking or excessive drinking, can lead to higher health risks and insurance premiums. Understanding these factors helps insurers assess risk more accurately. For more insights on how AI agents can personalize risk evaluation in insurance.
3.2. Auto Insurance Premium Optimization
Auto insurance premium optimization is the process of adjusting insurance premiums based on various factors to ensure that they are fair, competitive, and reflective of the risk associated with insuring a particular driver. This optimization is essential for both insurers and policyholders.
- Data-Driven Insights: Utilizing data analytics to assess risk factors such as driving history, vehicle type, and location can lead to more accurate premium pricing.
- Personalized Premiums: By analyzing individual risk profiles, insurers can offer personalized premiums that reflect the actual risk posed by the driver, rather than relying on broad categories.
- Discount Programs: Implementing discount programs for safe driving, bundling policies, or maintaining a good credit score can incentivize responsible behavior and reduce premiums.
- Market Competitiveness: Regularly reviewing and adjusting premiums based on market trends ensures that insurers remain competitive while still covering their risk exposure.
- Regulatory Compliance: Adhering to state regulations regarding premium pricing is crucial. Insurers must ensure that their pricing strategies comply with legal standards to avoid penalties.
3.2.1. Driver Behavior Analysis
Driver behavior analysis focuses on understanding how individual driving habits affect road safety and insurance risk. This analysis is increasingly important in the context of auto insurance, as it allows insurers to tailor their offerings based on actual driving behavior.
- Telematics Data: Many insurers now use telematics devices to monitor driving behavior in real-time. This data can include speed, braking patterns, and acceleration, providing insights into a driver's habits.
- Risk Assessment: Analyzing driver behavior helps insurers assess risk more accurately. For example, frequent hard braking or rapid acceleration may indicate aggressive driving, which can lead to higher premiums.
- Incentives for Safe Driving: Insurers can offer discounts or rewards for safe driving behaviors, encouraging policyholders to adopt safer habits. This not only benefits the driver but also reduces the insurer's risk.
- Behavioral Trends: Understanding broader trends in driver behavior, such as the impact of distracted driving or the effects of weather conditions, can help insurers develop better risk models.
- Feedback Mechanisms: Providing drivers with feedback on their driving habits can lead to improved safety. Many apps and devices offer insights that help drivers become more aware of their behavior behind the wheel.
At Rapid Innovation, we leverage advanced AI and blockchain technologies to enhance lifestyle factor analysis and auto insurance premium optimization. By integrating AI-driven analytics, we help clients gain deeper insights into lifestyle factors that influence health and financial stability, ultimately leading to more informed decision-making. Our blockchain solutions ensure data integrity and security, enabling insurers to build trust with policyholders while optimizing their premium strategies for greater ROI.
3.2.2. Real-time Risk Scoring
Real-time risk scoring is a dynamic approach to assessing the risk associated with insurance policies, particularly in auto and property insurance. This method leverages advanced technologies and data analytics to provide insurers with up-to-the-minute insights into risk factors.
- Continuous data collection: Insurers gather data from various sources, including telematics, social media, and IoT devices, to evaluate risk in real-time. Rapid Innovation can assist clients in implementing robust data collection frameworks that integrate these diverse data sources seamlessly.
- Enhanced accuracy: By utilizing real-time data, insurers can create more accurate risk profiles, leading to better underwriting decisions. Our AI-driven analytics solutions enable insurers to refine their risk assessment models, resulting in improved accuracy and reduced losses.
- Immediate adjustments: Real-time risk scoring allows insurers to adjust premiums or coverage based on the current risk level, ensuring that policyholders are charged fairly. Rapid Innovation's expertise in AI can help automate these adjustments, enhancing operational efficiency.
- Improved customer experience: With real-time insights, insurers can offer personalized services and proactive risk management advice to policyholders. Our consulting services can guide insurers in developing customer-centric strategies that leverage real-time data for enhanced engagement.
- Fraud detection: Real-time scoring can help identify suspicious activities or claims, reducing the likelihood of fraudulent claims being processed. Rapid Innovation employs advanced machine learning algorithms to bolster fraud detection capabilities, ensuring that insurers can mitigate risks effectively.
3.2.3. Telematics Integration
Telematics integration refers to the use of telecommunications and monitoring systems to collect and analyze data related to vehicle usage and driver behavior. This technology is increasingly being adopted in the insurance industry to enhance risk assessment and pricing strategies.
- Data collection: Telematics devices track various metrics, such as speed, braking patterns, and driving habits, providing insurers with valuable insights into driver behavior. Rapid Innovation can help clients implement telematics solutions that capture and analyze this data efficiently.
- Personalized premiums: By analyzing telematics data, insurers can offer usage-based insurance (UBI) policies, where premiums are based on actual driving behavior rather than traditional demographic factors. Our AI solutions enable insurers to develop sophisticated pricing models that reflect individual risk profiles accurately.
- Risk mitigation: Telematics can help identify high-risk behaviors, allowing insurers to provide targeted feedback and incentives for safer driving. Rapid Innovation's analytics capabilities can support insurers in creating effective risk mitigation programs that enhance driver safety.
- Enhanced claims processing: In the event of an accident, telematics data can provide crucial information to expedite claims processing and reduce disputes. Our blockchain solutions can further streamline claims management, ensuring transparency and efficiency in the process.
- Customer engagement: Telematics integration fosters a more interactive relationship between insurers and policyholders, encouraging safer driving through gamification and rewards. Rapid Innovation can assist in developing engaging platforms that leverage telematics data to enhance customer interaction.
3.3. Property Insurance Pricing
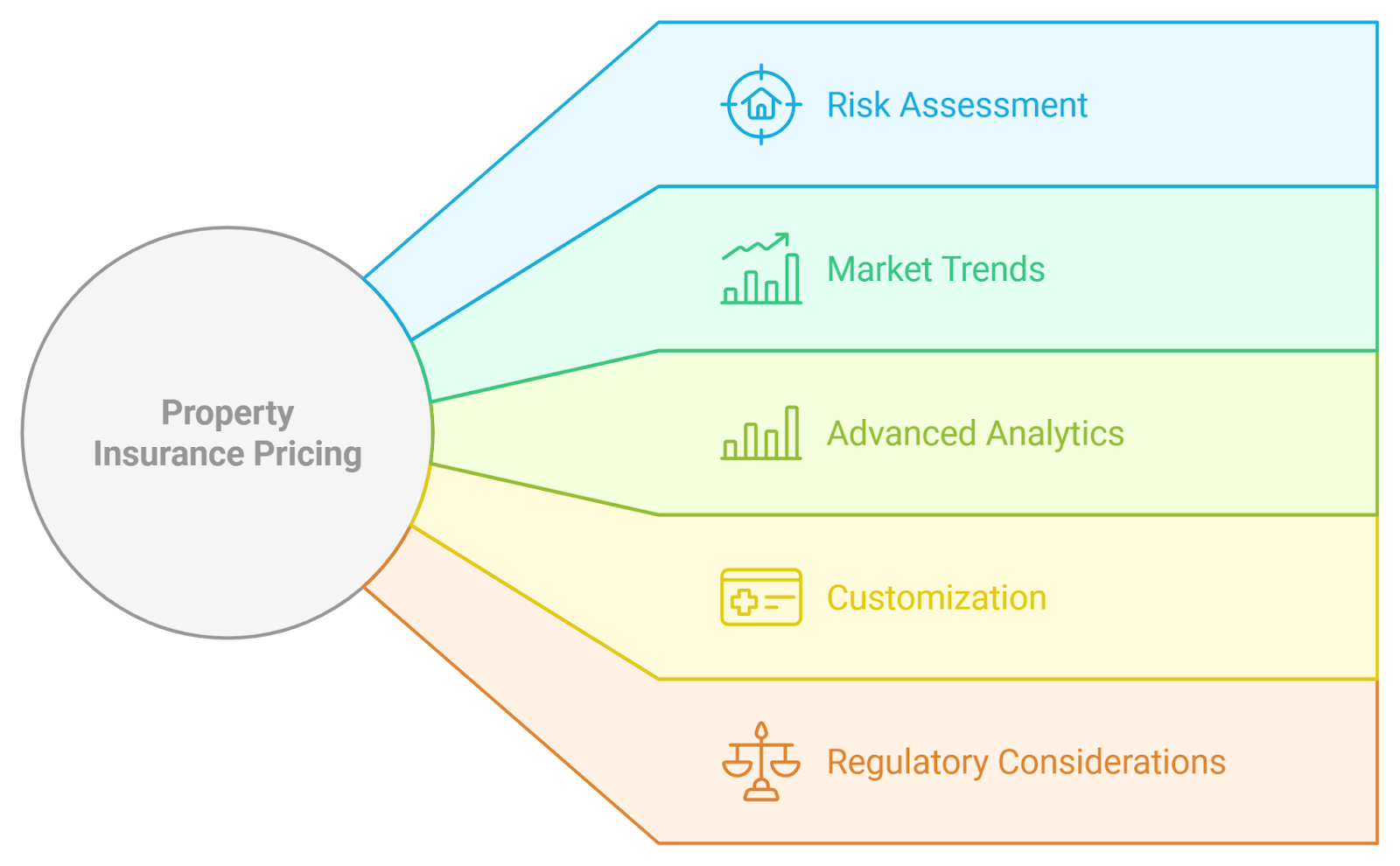
Property insurance pricing is a complex process that involves evaluating various factors to determine the appropriate premium for coverage. This process has evolved significantly with the advent of technology and data analytics.
- Risk assessment: Insurers analyze property characteristics, location, and historical claims data to assess the risk associated with insuring a particular property. Rapid Innovation's data analytics solutions can enhance this assessment, providing deeper insights into risk factors.
- Market trends: Property insurance pricing is influenced by market conditions, including supply and demand, competition, and economic factors. Our consulting services can help insurers navigate these trends effectively, ensuring competitive pricing strategies.
- Advanced analytics: Insurers utilize predictive modeling and machine learning algorithms to forecast potential losses and set premiums accordingly. Rapid Innovation specializes in developing advanced analytics frameworks that empower insurers to make data-driven decisions.
- Customization: Insurers are increasingly offering personalized pricing based on individual risk profiles, allowing policyholders to pay premiums that reflect their specific risk levels. Our AI solutions enable insurers to tailor their offerings, enhancing customer satisfaction and retention.
- Regulatory considerations: Property insurance pricing must comply with state regulations, which can vary significantly, impacting how insurers set their rates. Rapid Innovation can provide guidance on regulatory compliance, ensuring that pricing strategies align with legal requirements.
By understanding these components, insurers can create more effective pricing strategies that align with market demands and customer expectations. Rapid Innovation is committed to helping clients leverage AI and blockchain technologies to achieve greater ROI and operational efficiency in the insurance sector.
3.3.1. Geographical Risk Mapping
Geographical risk mapping is a crucial process in identifying and analyzing potential hazards in specific locations. This method utilizes geographic information systems (GIS) to visualize and assess risks associated with natural disasters, environmental changes, and human activities.
- Identifies high-risk areas: By mapping geographical data, stakeholders can pinpoint regions that are more susceptible to disasters such as floods, earthquakes, or landslides.
- Integrates various data sources: Geographical risk mapping combines satellite imagery, topographical maps, and demographic data to create a comprehensive risk profile.
- Supports decision-making: Policymakers and urban planners can use these maps to develop strategies for disaster preparedness, response, and recovery.
- Enhances community awareness: By visualizing risks, communities can better understand their vulnerabilities and take proactive measures to mitigate them.
At Rapid Innovation, we leverage AI algorithms to enhance geographical risk mapping, enabling clients to gain deeper insights and make data-driven decisions. Our solutions can help organizations optimize resource allocation and improve disaster response strategies, ultimately leading to greater ROI. For more information on our services, visit our AI insurance solutions and learn more about our predictive analytics and AI simulation for high-stakes decisions.
3.3.2. Structural Vulnerability Assessment
Structural vulnerability assessment focuses on evaluating the resilience of buildings and infrastructure against various hazards. This assessment is vital for ensuring safety and minimizing damage during disasters.
- Evaluates building materials: The assessment examines the quality and durability of materials used in construction, which can significantly impact a structure's ability to withstand extreme weather or seismic events.
- Considers design and engineering: Structural vulnerability assessments analyze architectural designs and engineering practices to identify weaknesses that could lead to failure during a disaster.
- Assesses location and site conditions: The geographical context, including soil stability and proximity to fault lines or flood zones, is crucial in determining a structure's vulnerability.
- Provides recommendations for improvement: Based on the assessment, engineers and architects can suggest retrofitting or redesigning structures to enhance their resilience.
At Rapid Innovation, we utilize advanced AI techniques to conduct structural vulnerability assessments, allowing clients to identify potential weaknesses and implement cost-effective solutions. This proactive approach not only safeguards lives and property but also maximizes the return on investment in infrastructure improvements.
3.3.3. Climate Risk Modeling
Climate risk modeling involves the use of advanced simulations and statistical methods to predict the potential impacts of climate change on various systems. This modeling is essential for understanding future risks and developing effective adaptation strategies.
- Analyzes climate data: Climate risk modeling relies on historical and current climate data to project future scenarios, including temperature changes, precipitation patterns, and extreme weather events.
- Evaluates potential impacts: The models assess how climate change could affect ecosystems, agriculture, water resources, and human health, providing a comprehensive view of potential risks.
- Supports policy development: By understanding the risks associated with climate change, governments and organizations can create informed policies and strategies to mitigate these impacts.
- Facilitates stakeholder engagement: Climate risk modeling helps engage communities and stakeholders in discussions about climate resilience and adaptation measures.
Rapid Innovation employs cutting-edge AI and blockchain technologies to enhance climate risk modeling, ensuring data integrity and facilitating transparent stakeholder engagement. Our solutions empower organizations to make informed decisions that not only address climate risks but also drive sustainable growth and greater ROI. Effective climate risk modeling is crucial for proactive planning and resource management, enabling communities and organizations to prepare for the uncertainties posed by climate change and to implement strategies that enhance resilience.
3.4. Health Insurance Premium Determination
Health insurance premium determination is a complex process that involves various factors to assess the risk associated with insuring an individual or group. Insurers use a combination of statistical data, personal health information, and market trends to set premiums that reflect the expected costs of providing coverage. Understanding how health insurance premium determination works can help consumers make informed decisions about their health insurance options.
- Insurers analyze historical claims data to predict future costs.
- Premiums can vary significantly based on age, location, and health status.
- Regulatory factors and market competition also influence premium rates.
3.4.1. Personalized Risk Profiling
Personalized risk profiling is a method used by insurers to evaluate an individual's health risks based on their unique characteristics. This approach allows for a more tailored premium calculation, reflecting the specific health risks associated with each policyholder. Factors considered in risk profiling include age and gender, medical history and pre-existing conditions, lifestyle choices (e.g., smoking, diet, exercise), and family health history. Advanced data analytics and algorithms are employed to assess risk more accurately. Personalized risk profiles can lead to more equitable premium pricing, as healthier individuals may pay lower rates.
At Rapid Innovation, we leverage AI-driven analytics to enhance personalized risk profiling. By utilizing machine learning algorithms, we help insurers refine their risk assessment processes, leading to more accurate premium calculations and improved customer satisfaction.
3.4.2. Predictive Health Outcomes
Predictive health outcomes refer to the use of data analytics to forecast an individual's future health status and potential medical costs. Insurers leverage predictive modeling to identify high-risk individuals and adjust premiums accordingly. Key components of predictive health outcomes include the analysis of demographic data and health trends, utilization of machine learning algorithms to identify patterns, and integration of electronic health records for comprehensive insights. Predictive analytics can help insurers anticipate future claims and healthcare needs, implement preventive measures to reduce costs, and offer personalized wellness programs to policyholders. By understanding potential health outcomes, insurers can create more sustainable pricing models that benefit both the insurer and the insured.
Rapid Innovation's expertise in AI and data analytics empowers insurers to harness predictive health outcomes effectively. Our solutions enable clients to implement proactive health management strategies, ultimately leading to reduced costs and enhanced ROI. For more information on how we can assist with AI for mental health care, visit our AI for Mental Health Care.
3.4.3. Treatment Cost Forecasting
Treatment cost forecasting is a critical aspect of healthcare management that involves predicting the expenses associated with medical treatments and procedures. Accurate treatment cost forecasting helps healthcare providers, insurers, and patients make informed financial decisions.
- Importance of Treatment Cost Forecasting:
- Enhances budgeting and financial planning for healthcare organizations.
- Improves patient transparency regarding potential out-of-pocket expenses.
- Aids in resource allocation and management within healthcare facilities.
- Factors Influencing Treatment Costs:
- Type of treatment or procedure: Different treatments have varying costs based on complexity and required resources.
- Patient demographics: Age, gender, and health status can affect treatment needs and associated costs.
- Geographic location: Costs can vary significantly based on regional healthcare pricing and availability of services.
- Techniques for Cost Forecasting:
- Historical data analysis: Utilizing past treatment costs to predict future expenses.
- Predictive modeling: Employing statistical methods and algorithms to forecast costs based on various factors.
- Machine learning: Leveraging advanced technologies to analyze large datasets for more accurate predictions.
- Challenges in Treatment Cost Forecasting:
- Variability in treatment protocols: Different providers may have different approaches, leading to cost discrepancies.
- Changes in healthcare regulations: New policies can impact pricing structures and reimbursement rates.
- Patient-specific factors: Individual patient needs can complicate cost predictions.
3.5. Commercial Insurance Pricing
Commercial insurance pricing is the process of determining the premiums that businesses and individuals pay for insurance coverage. This pricing is influenced by various factors, including risk assessment, market conditions, and regulatory requirements.
- Key Components of Insurance Pricing:
- Risk assessment: Insurers evaluate the likelihood of claims based on historical data and individual risk profiles.
- Underwriting criteria: Factors such as age, health status, and lifestyle choices are considered in determining premiums.
- Market competition: Insurers must remain competitive while ensuring profitability, which can influence pricing strategies.
- Pricing Models:
- Experience rating: Premiums are based on the insured's past claims history.
- Community rating: Premiums are set based on the overall risk of a group rather than individual claims history.
- Modified community rating: A blend of experience and community rating, considering specific factors like age and geography.
- Trends in Commercial Insurance Pricing:
- Increasing healthcare costs: Rising medical expenses can lead to higher premiums for commercial insurance.
- Regulatory changes: New laws and regulations can impact pricing structures and coverage options.
- Technological advancements: The use of data analytics and artificial intelligence is transforming how insurers assess risk and set prices.
3.5.1. Industry-Specific Risk Analysis
Industry-specific risk analysis involves evaluating the unique risks associated with different sectors to inform insurance pricing and coverage options. This analysis is crucial for insurers to tailor their products to meet the specific needs of various industries.
- Importance of Industry-Specific Risk Analysis:
- Enhances understanding of sector-specific challenges and exposures.
- Allows for more accurate pricing based on the unique risk profiles of different industries.
- Supports the development of customized insurance products that address specific needs.
- Factors Considered in Risk Analysis:
- Regulatory environment: Different industries face varying levels of regulation, impacting risk exposure.
- Operational risks: Each industry has unique operational challenges that can affect risk levels.
- Economic factors: Market conditions and economic trends can influence the risk landscape for specific sectors.
- Common Industries Analyzed:
- Healthcare: Risks related to patient safety, malpractice, and regulatory compliance.
- Construction: Risks associated with site safety, equipment failure, and project delays.
- Technology: Risks related to data breaches, intellectual property, and rapid innovation.
- Benefits of Industry-Specific Risk Analysis:
- Improved risk management strategies tailored to specific industries.
- Enhanced ability to predict and mitigate potential losses.
- Better alignment of insurance products with the needs of businesses in various sectors.
At Rapid Innovation, we leverage our expertise in AI and blockchain technologies to enhance treatment cost forecasting and commercial insurance pricing. By utilizing machine learning algorithms and predictive analytics, we help healthcare organizations and insurers achieve greater accuracy in their financial planning and risk assessment, ultimately leading to improved ROI. Our tailored solutions ensure that clients can navigate the complexities of treatment cost forecasting and insurance pricing with confidence and efficiency. For more information, visit our AI risk scoring in insurance page.
3.5.2. Business Performance Prediction
Business performance prediction involves using data analytics and machine learning algorithms to forecast future business outcomes. This process is crucial for organizations aiming to make informed decisions based on predictive insights.
- Predictive analytics leverages historical data to identify trends and patterns.
- Machine learning models can analyze vast datasets to improve accuracy over time.
- Key performance indicators (KPIs) are often used to measure success and guide predictions.
- Businesses can anticipate market changes, customer behavior, and operational efficiency.
- Enhanced forecasting can lead to better resource allocation and strategic planning.
Organizations can utilize various tools and techniques for business performance prediction, including:
- Time series analysis to forecast sales and revenue.
- Regression analysis to understand relationships between variables.
- Scenario analysis to evaluate potential outcomes based on different assumptions.
By implementing these predictive models, businesses can gain a competitive edge, reduce uncertainty, and enhance decision-making processes. For instance, companies that effectively use predictive analytics can improve their sales forecasting accuracy by up to 20%. Rapid Innovation specializes in integrating advanced AI solutions that empower organizations to harness the full potential of their data, driving greater ROI through informed decision-making and strategic foresight.
3.5.3. Complex Risk Scenario Modeling
Complex risk scenario modeling is a sophisticated approach to understanding and managing risks within an organization. This method involves simulating various risk scenarios to evaluate potential impacts on business operations and financial performance.
- Risk modeling helps identify vulnerabilities and assess the likelihood of adverse events.
- It incorporates various factors, including market volatility, regulatory changes, and operational risks.
- Organizations can use Monte Carlo simulations to model uncertainty and predict outcomes.
- Stress testing is another technique that evaluates how a business would perform under extreme conditions.
Key benefits of complex risk scenario modeling include:
- Improved risk management strategies by understanding potential threats.
- Enhanced decision-making through data-driven insights.
- Greater resilience against unforeseen events by preparing for various scenarios.
By employing complex risk scenario modeling, businesses can proactively address risks and develop contingency plans. This approach is particularly valuable in industries such as finance, insurance, and healthcare, where risk management is critical to success. Rapid Innovation's expertise in blockchain technology can further enhance risk management by providing transparent and immutable records, ensuring that organizations can trust their data and make informed decisions.
4. Technical Architecture of AI Premium Calculation Agents
The technical architecture of AI premium calculation agents is designed to streamline the process of calculating insurance premiums using artificial intelligence. This architecture typically consists of several key components that work together to deliver accurate and efficient premium calculations.
- Data Ingestion: The first step involves collecting data from various sources, including customer information, historical claims data, and external market data. This data is essential for training AI models.
- Data Processing: Once the data is ingested, it undergoes preprocessing to clean and normalize it. This step ensures that the data is suitable for analysis and modeling.
- Machine Learning Models: AI premium calculation agents utilize machine learning algorithms to analyze the processed data. These models can identify patterns and correlations that inform premium pricing.
- User Interface: A user-friendly interface allows insurance agents and customers to interact with the premium calculation system. This interface provides real-time feedback and visualizations of premium calculations.
- Integration with Legacy Systems: The architecture must integrate seamlessly with existing insurance systems to ensure smooth operations. This integration allows for the sharing of data and insights across platforms.
- Continuous Learning: AI premium calculation agents are designed to learn continuously from new data. This capability enables them to adapt to changing market conditions and improve their accuracy over time.
By implementing a robust technical architecture, organizations can enhance their premium calculation processes, reduce manual errors, and improve customer satisfaction. The use of AI in premium calculations can lead to more personalized pricing, ultimately benefiting both insurers and policyholders. Rapid Innovation's commitment to leveraging cutting-edge AI technologies ensures that clients can achieve operational excellence and maximize their return on investment.
4.1. Data Collection Mechanisms
Data collection is a critical step in any data-driven project. It involves gathering relevant information that will be used for analysis, model training, and decision-making. Effective data collection mechanisms ensure that the data is accurate, reliable, and representative of the problem being addressed. At Rapid Innovation, we leverage these mechanisms to help our clients achieve their business goals efficiently.
- Surveys and Questionnaires: These tools are commonly used to gather qualitative and quantitative data directly from individuals. They can be distributed online or in person, allowing for a wide reach. By utilizing targeted surveys, we help clients gain insights into customer preferences, leading to improved product offerings and increased customer satisfaction.
- Web Scraping: This technique involves extracting data from websites. It is useful for collecting large datasets from online sources, such as social media, e-commerce sites, and news articles. Rapid Innovation employs web scraping to gather competitive intelligence, enabling clients to make informed strategic decisions that enhance their market positioning.
- APIs (Application Programming Interfaces): Many platforms provide APIs that allow developers to access their data programmatically. This is particularly useful for real-time data collection from services like Twitter, Google, or financial markets. Our expertise in API integration allows clients to harness real-time data, improving their responsiveness to market changes and customer needs.
- IoT Devices: Internet of Things devices collect data from the physical world, such as sensors in smart homes or wearables. This data can provide insights into user behavior and environmental conditions. Rapid Innovation helps clients implement IoT solutions that optimize operations and enhance user experiences, ultimately driving greater ROI.
- Databases: Existing databases can be tapped into for historical data. This includes public datasets, company records, and academic research databases. By analyzing historical data, we assist clients in identifying trends and making data-driven predictions that inform their strategic planning.
4.2. Feature Engineering
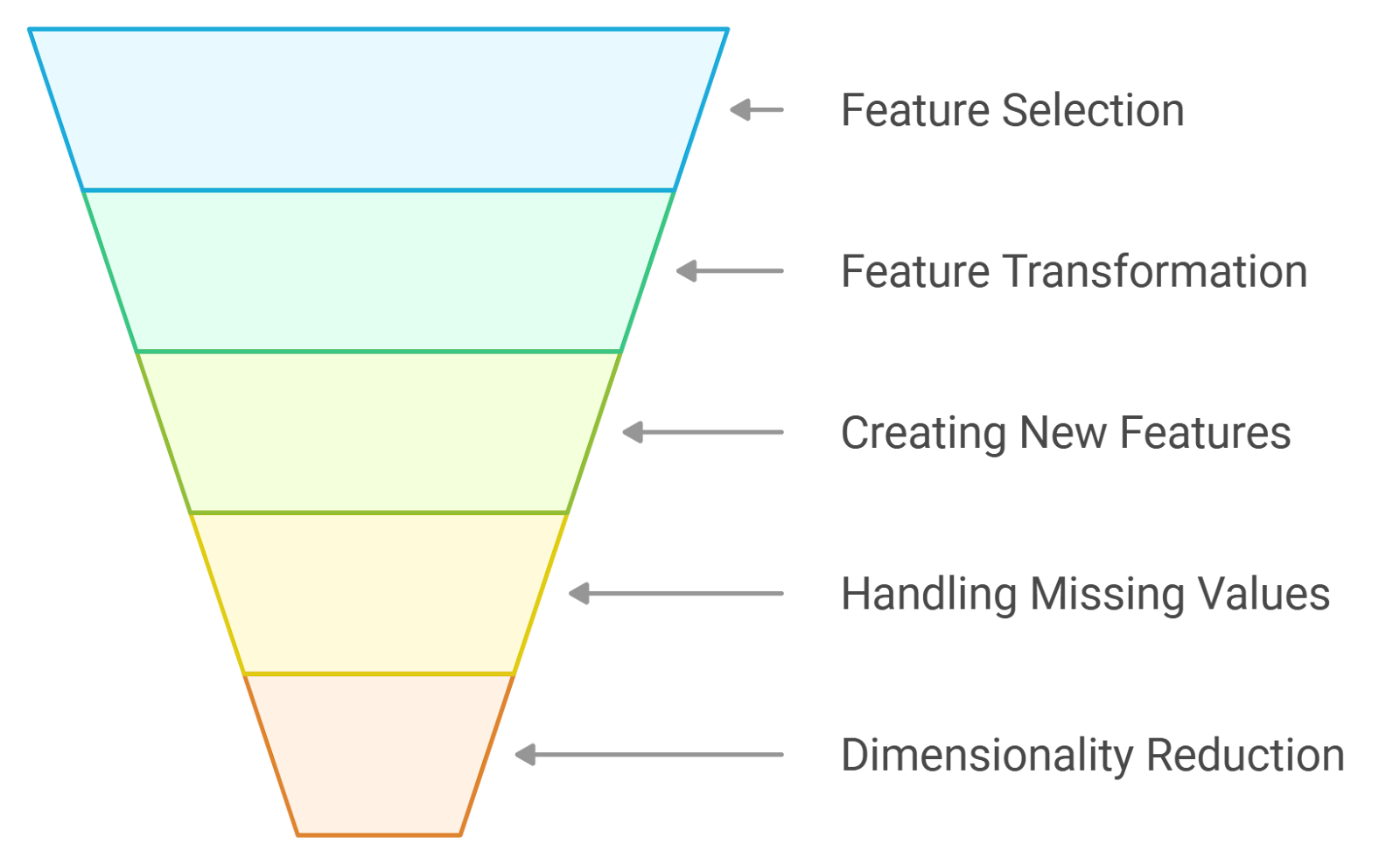
Feature engineering is the process of selecting, modifying, or creating new features from raw data to improve the performance of machine learning models. It plays a crucial role in determining the success of predictive analytics. At Rapid Innovation, we focus on feature engineering to ensure our clients' models deliver actionable insights.
- Feature Selection: This involves identifying the most relevant features that contribute to the model's predictive power. Techniques like Recursive Feature Elimination (RFE) and Lasso regression can help in this process. Our team employs these techniques to enhance model accuracy, ensuring clients achieve better outcomes from their data initiatives.
- Feature Transformation: Raw data often needs to be transformed to be useful. Common transformations include normalization, standardization, and encoding categorical variables into numerical formats. We apply these transformations to prepare data for analysis, maximizing the effectiveness of our clients' machine learning models.
- Creating New Features: Sometimes, new features can be derived from existing ones. For example, combining date and time into a single timestamp or creating interaction terms between variables can provide additional insights. Our innovative approach to feature creation helps clients uncover hidden patterns in their data.
- Handling Missing Values: Missing data can skew results. Techniques such as imputation, where missing values are filled in based on other data, or removing records with missing values, are essential in feature engineering. We ensure that our clients' datasets are clean and reliable, leading to more accurate predictions.
- Dimensionality Reduction: Techniques like Principal Component Analysis (PCA) can reduce the number of features while retaining essential information, making models more efficient and easier to interpret. Rapid Innovation utilizes dimensionality reduction to streamline models, enhancing performance and interpretability for our clients.
4.3. Model Development Frameworks
Model development frameworks provide the tools and libraries necessary for building, training, and deploying machine learning models. They streamline the process and enhance productivity. At Rapid Innovation, we leverage these frameworks to deliver robust AI solutions that drive business success.
- TensorFlow: An open-source framework developed by Google, TensorFlow is widely used for deep learning applications. It offers flexibility and scalability, making it suitable for both research and production environments. Our expertise in TensorFlow allows us to build sophisticated models that meet our clients' unique needs.
- PyTorch: Known for its dynamic computation graph, PyTorch is favored in academia and research. It allows for easy debugging and is particularly effective for tasks involving natural language processing and computer vision. Rapid Innovation harnesses PyTorch to develop cutting-edge AI applications that provide clients with a competitive edge.
- Scikit-learn: This Python library is ideal for traditional machine learning algorithms. It provides simple and efficient tools for data mining and data analysis, making it accessible for beginners and experts alike. We utilize Scikit-learn to implement proven algorithms that deliver reliable results for our clients.
- Keras: A high-level neural networks API, Keras runs on top of TensorFlow. It simplifies the process of building deep learning models, allowing for rapid prototyping and experimentation. Our team uses Keras to accelerate model development, ensuring timely delivery of solutions that meet client expectations.
- Apache Spark: For big data applications, Apache Spark offers a powerful framework for distributed data processing. Its MLlib library provides scalable machine learning algorithms that can handle large datasets efficiently. Rapid Innovation employs Apache Spark to enable clients to process and analyze vast amounts of data, unlocking valuable insights that drive growth.
4.4. Real-time Processing Capabilities
Real-time processing capabilities are essential for businesses that require immediate data analysis and decision-making. This feature allows organizations to respond to events as they happen, enhancing operational efficiency and customer satisfaction.
- Instant data analysis: Real-time processing enables the immediate analysis of incoming data streams, allowing businesses to make quick decisions based on the latest information. Rapid Innovation employs advanced AI algorithms to facilitate this instant analysis, ensuring that our clients can act on real-time insights effectively. Technologies such as real time data processing using Apache Kafka and Spark streaming are integral to this capability.
- Enhanced customer experience: By processing data in real-time, companies can provide personalized services and immediate responses to customer inquiries, improving overall satisfaction. Our solutions help businesses leverage AI to tailor interactions, thereby enhancing customer engagement and loyalty. For instance, real time data enrichment can significantly improve the quality of customer interactions.
- Competitive advantage: Organizations that leverage real-time processing can react faster to market changes, giving them an edge over competitors who rely on batch processing. Rapid Innovation's expertise in AI and blockchain allows clients to harness real-time data for strategic decision-making, positioning them ahead in their respective markets. Real time analytics processing is a key component of this competitive strategy.
- Use cases: Industries such as finance, healthcare, and e-commerce benefit significantly from real-time processing. For example, financial institutions use real-time data to detect fraudulent transactions instantly, a capability that Rapid Innovation can help implement through our tailored solutions. Real time stream analytics is particularly useful in these scenarios.
- Technology stack: Implementing real-time processing often involves technologies like Apache Kafka, Apache Flink, or AWS Kinesis, which facilitate the handling of large data streams efficiently. Rapid Innovation specializes in integrating these technologies into existing infrastructures, ensuring seamless operation and maximum ROI. Our expertise includes real time data ingestion and real time ETL processes to streamline data flow.
4.5. Integration with Existing Systems
Integration with existing systems is crucial for ensuring that new technologies work seamlessly with current infrastructure. This capability minimizes disruption and maximizes the value of investments in new solutions.
- Compatibility: New systems should be compatible with legacy systems to ensure smooth data flow and operational continuity. Rapid Innovation focuses on creating solutions that bridge the gap between old and new technologies, ensuring a smooth transition. This includes ensuring compatibility with near real time ETL processes.
- API utilization: Application Programming Interfaces (APIs) play a vital role in integration, allowing different software applications to communicate and share data effectively. Our team at Rapid Innovation excels in developing robust APIs that enhance interoperability and streamline operations.
- Data synchronization: Ensuring that data is synchronized across platforms is essential for maintaining accuracy and consistency in reporting and analytics. We implement solutions that guarantee real-time data synchronization, enabling our clients to make informed decisions based on accurate information. This is particularly important in environments that require real time data integration.
- Reduced costs: Effective integration can reduce the need for extensive retraining of staff and minimize the costs associated with implementing entirely new systems. Rapid Innovation's approach to integration is designed to be cost-effective, maximizing the return on investment for our clients.
- Incremental upgrades: Organizations can adopt a phased approach to integration, gradually upgrading components without overhauling the entire system at once. Our consulting services guide clients through this process, ensuring minimal disruption and maximum efficiency.
4.6. Scalability Considerations
Scalability is a critical factor for businesses looking to grow and adapt to changing market demands. A scalable system can handle increased loads without compromising performance.
- Horizontal vs. vertical scaling: Horizontal scaling involves adding more machines to handle increased load, while vertical scaling means upgrading existing machines. Both approaches have their advantages and should be considered based on specific business needs. Rapid Innovation provides tailored strategies to help clients choose the best scaling approach for their operations.
- Cloud solutions: Many organizations are turning to cloud-based solutions for scalability, as they offer flexible resources that can be adjusted based on demand. Our expertise in cloud technologies ensures that clients can leverage scalable solutions that align with their growth objectives, including real time data processing on cloud platforms.
- Performance monitoring: Regularly monitoring system performance helps identify bottlenecks and areas that require scaling, ensuring that the system can handle future growth. Rapid Innovation implements advanced monitoring tools that provide actionable insights, enabling proactive management of system performance.
- Cost implications: Scalability should be planned with cost in mind. Businesses need to evaluate the financial impact of scaling up resources and ensure that it aligns with their budget. Our consulting services include cost analysis to help clients make informed decisions about their scaling strategies.
- Future-proofing: Investing in scalable solutions helps future-proof the organization, allowing it to adapt to technological advancements and market changes without significant disruptions. Rapid Innovation's commitment to innovation ensures that our clients are always equipped to meet future challenges head-on, including the integration of real time stream processing capabilities.
5. Benefits of AI-Driven Premium Calculation
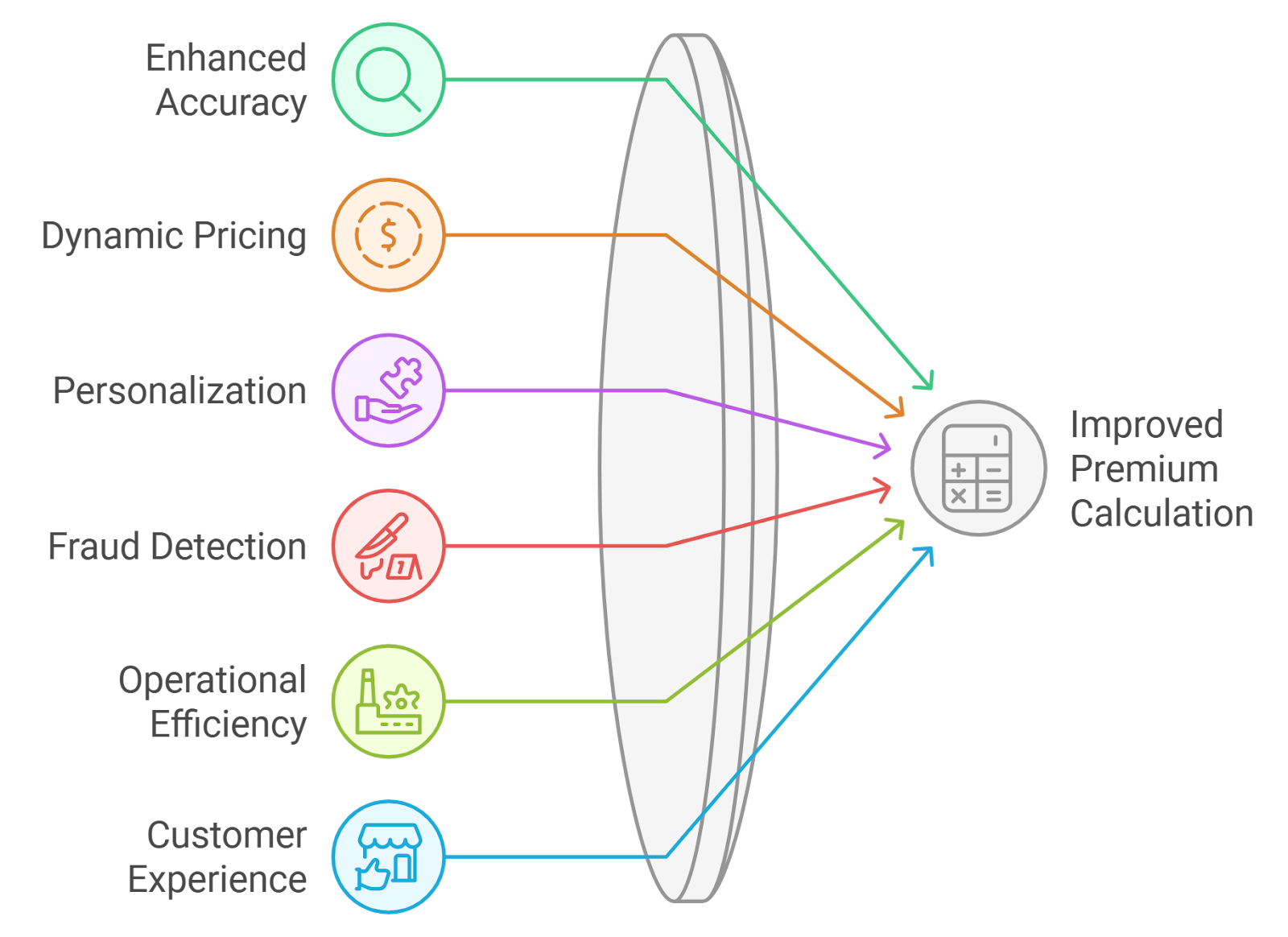
5.1. Enhanced Accuracy
AI-driven premium calculation significantly improves the accuracy of insurance pricing. Traditional methods often rely on historical data and manual processes, which can lead to errors and inconsistencies. AI algorithms, on the other hand, analyze vast amounts of data quickly and efficiently, ensuring that the premium calculations are based on the most relevant and up-to-date information.
- AI can process data from various sources, including social media, IoT devices, and customer behavior patterns.
- Machine learning models continuously learn from new data, refining their predictions over time.
- Enhanced accuracy reduces the risk of underpricing or overpricing policies, leading to better financial stability for insurers.
By leveraging AI-driven premium calculation, insurers can achieve a more precise understanding of risk factors, which allows for tailored premium calculations that reflect the true risk associated with each policyholder. At Rapid Innovation, we specialize in implementing AI solutions that enhance accuracy in premium calculations, ultimately driving greater ROI for our clients. You can learn more about this in our article on the secret to better pricing with artificial intelligence.
5.2. Dynamic Pricing Strategies
AI-driven premium calculation enables insurers to implement dynamic pricing strategies that adapt to changing market conditions and individual customer profiles. This flexibility is crucial in a competitive insurance landscape where customer expectations are evolving.
- Dynamic pricing allows insurers to adjust premiums in real-time based on various factors, such as market trends, customer behavior, and risk assessments.
- AI can identify patterns in customer data, enabling insurers to offer personalized premiums that reflect individual risk profiles.
- This approach not only enhances customer satisfaction but also improves retention rates, as customers feel they are being charged fairly based on their unique circumstances.
By utilizing AI-driven premium calculation for dynamic pricing, insurers can respond quickly to market changes, optimize their pricing strategies, and ultimately drive profitability. Rapid Innovation's expertise in AI technology empowers our clients to implement these dynamic pricing strategies effectively, ensuring they remain competitive and achieve their business goals efficiently.
5.3. Personalization at Scale
Personalization at scale refers to the ability of businesses to tailor their products, services, and marketing efforts to individual customer preferences and behaviors, even when dealing with large volumes of data. This approach enhances customer experience and drives engagement, leading to increased loyalty and sales.
- Data-Driven Insights: Companies leverage data analytics to understand customer behavior, preferences, and purchasing patterns. This information allows for targeted marketing campaigns and personalized recommendations, ultimately driving higher conversion rates and ROI. For instance, personalization at scale examples can illustrate how different companies have successfully implemented these strategies.
- AI and Machine Learning: Advanced technologies like AI and machine learning enable businesses to automate personalization efforts. These tools analyze vast amounts of data to predict customer needs and deliver relevant content in real-time, ensuring that marketing efforts are both efficient and effective. Adobe personalization at scale is a prime example of how technology can enhance these efforts.
- Dynamic Content Delivery: Personalization can be achieved through dynamic content that changes based on user interactions. For example, e-commerce websites can display products based on previous searches or purchases, enhancing the shopping experience and increasing sales opportunities. This is a key aspect of digital personalization at scale.
- Customer Segmentation: By segmenting customers into distinct groups based on demographics, interests, or behaviors, businesses can create tailored marketing strategies that resonate with each segment. This targeted approach leads to improved engagement and higher return on investment. Mckinsey personalization at scale provides insights into effective segmentation strategies.
- Omnichannel Experience: Personalization at scale ensures a consistent experience across all channels, whether online or offline. This approach helps maintain brand loyalty and improves customer satisfaction, ultimately contributing to long-term business success. The concept of personalization at scale adobe emphasizes the importance of a seamless omnichannel experience.
5.4. Fraud Detection and Prevention
Fraud detection and prevention are critical components of maintaining trust and security in any business, especially in the digital landscape. With the rise of online transactions, businesses must implement robust systems to identify and mitigate fraudulent activities.
- Real-Time Monitoring: Advanced algorithms and machine learning models can analyze transactions in real-time to detect unusual patterns that may indicate fraud. This proactive approach allows businesses to respond quickly to potential threats, minimizing financial losses.
- Behavioral Analytics: By understanding typical customer behavior, businesses can identify anomalies that may suggest fraudulent activity. For instance, a sudden change in purchasing patterns can trigger alerts for further investigation, enhancing the overall security posture.
- Multi-Factor Authentication: Implementing multi-factor authentication adds an extra layer of security, making it more difficult for fraudsters to gain unauthorized access to accounts. This measure not only protects customer data but also builds trust in the brand.
- Data Encryption: Protecting sensitive customer information through encryption helps prevent data breaches and unauthorized access, reducing the risk of fraud and ensuring compliance with regulatory standards.
- Continuous Improvement: Regularly updating fraud detection systems and algorithms ensures that businesses stay ahead of evolving fraud tactics. Continuous learning from past incidents can enhance future prevention strategies, ultimately safeguarding the organization’s assets.
5.5. Operational Efficiency
Operational efficiency refers to the ability of a business to deliver products or services in the most cost-effective manner while maintaining high quality. Improving operational efficiency can lead to significant cost savings and enhanced productivity.
- Process Automation: Automating repetitive tasks reduces human error and frees up employees to focus on more strategic activities. Tools like robotic process automation (RPA) can streamline workflows and improve efficiency, leading to better resource allocation.
- Lean Methodologies: Implementing lean principles helps identify and eliminate waste in processes. This approach focuses on maximizing value for customers while minimizing resources, ultimately driving profitability.
- Performance Metrics: Establishing key performance indicators (KPIs) allows businesses to measure efficiency and identify areas for improvement. Regularly reviewing these metrics can drive continuous enhancement and ensure alignment with business goals.
- Employee Training: Investing in employee training ensures that staff are equipped with the skills needed to operate efficiently. Well-trained employees are more productive and can contribute to a positive work environment, fostering innovation.
- Technology Integration: Utilizing integrated software solutions can enhance communication and collaboration across departments. This integration reduces silos and improves overall operational flow, enabling businesses to respond swiftly to market changes and customer needs.
At Rapid Innovation, we leverage our expertise in AI and Blockchain to help clients implement these strategies effectively, ensuring they achieve their business goals with greater efficiency and ROI.
5.6. Customer Experience Improvement
Customer experience (CX) is a critical factor in the success of any business. Improving CX involves understanding customer needs and preferences, and then tailoring services and products to meet those expectations. Here are some key strategies for enhancing customer experience:
- Personalization: Leveraging AI algorithms to analyze customer data allows businesses to tailor interactions significantly, improving satisfaction through personalized recommendations, targeted marketing, and customized communication.
- Omnichannel Support: Implementing blockchain technology can ensure seamless support across various channels—such as social media, email, and live chat—enabling customers to reach out in their preferred way. This consistency builds trust and loyalty.
- Feedback Mechanisms: Utilizing AI-driven analytics to actively seek customer feedback through surveys, reviews, and direct communication helps identify areas for improvement. Implementing changes based on this feedback demonstrates to customers that their opinions matter. This is crucial for how to improve customer experience.
- Employee Training: Well-trained employees who understand the importance of customer service can create positive interactions. Investing in training programs can lead to better customer engagement and satisfaction, which is essential for how to improve customer service.
- Technology Integration: Utilizing technology, such as AI-powered chatbots and advanced CRM systems, can streamline customer interactions and provide quick responses to inquiries. This efficiency enhances the overall customer experience and is a key aspect of how to improve customer experience management.
- Proactive Communication: Keeping customers informed about their orders, service updates, or potential issues can prevent frustration. Proactive communication fosters a sense of reliability and care, which is vital for how to improve customer care.
- Consistent Branding: A consistent brand message across all platforms reinforces trust and recognition. Customers are more likely to engage with brands that present a unified image, contributing to how to increase customer experience.
6. Challenges and Limitations

While improving customer experience is essential, businesses face several challenges and limitations that can hinder their efforts. Understanding these obstacles is crucial for developing effective strategies.
- Resource Constraints: Many businesses, especially small and medium-sized enterprises, may lack the necessary resources—financial or human—to implement comprehensive CX improvement strategies.
- Resistance to Change: Employees and management may resist changes in processes or technology. This resistance can slow down the implementation of new customer experience initiatives.
- Balancing Automation and Human Touch: While technology can enhance efficiency, over-reliance on automation may lead to impersonal interactions. Striking the right balance between automated services and human engagement is vital.
- Measuring Success: Determining the effectiveness of customer experience initiatives can be challenging. Businesses often struggle to find the right metrics to gauge improvement accurately, which is important for customer experience improvement.
- Keeping Up with Trends: The rapidly changing landscape of customer expectations and technology can make it difficult for businesses to stay relevant. Continuous adaptation is necessary but can be resource-intensive.
6.1. Data Quality and Availability
Data quality and availability are fundamental to improving customer experience. High-quality data enables businesses to make informed decisions and tailor their offerings effectively. However, several issues can arise in this area:
- Incomplete Data: Many organizations struggle with incomplete customer data, which can lead to inaccurate insights. This lack of information can hinder personalized marketing efforts and customer engagement strategies.
- Data Silos: When data is stored in separate systems or departments, it becomes challenging to access a comprehensive view of the customer. Breaking down these silos is essential for a holistic understanding of customer behavior.
- Data Accuracy: Ensuring that customer data is accurate and up-to-date is crucial. Inaccurate data can lead to misguided strategies and poor customer experiences.
- Privacy Concerns: With increasing regulations around data privacy, businesses must navigate the complexities of collecting and using customer data responsibly. Balancing data utilization with privacy compliance is a significant challenge.
- Integration Issues: Integrating various data sources can be complex. Businesses often face difficulties in consolidating data from different platforms, which can impede their ability to analyze customer behavior effectively.
- Real-Time Access: Having access to real-time data is essential for making timely decisions. However, many organizations struggle with outdated systems that do not provide immediate insights.
- Data Literacy: Employees may lack the skills to analyze and interpret data effectively. Investing in training and tools to enhance data literacy can empower teams to leverage data for better customer experiences.
At Rapid Innovation, we specialize in harnessing AI and blockchain technologies to address these challenges, ensuring that our clients can enhance their customer experience strategies effectively and efficiently, ultimately leading to greater ROI. This includes how to improve client experience and how to improve guest service. For more information, visit our AI-driven customer support.
6.2. Ethical Considerations
Ethical considerations are paramount in any field, especially in technology and data-driven industries. As organizations increasingly rely on algorithms and artificial intelligence, it is crucial to address the ethical implications of these technologies.
- Transparency: Organizations should ensure that their processes and algorithms are transparent. Stakeholders must understand how decisions are made, particularly when they affect individuals' lives. At Rapid Innovation, we emphasize the importance of transparency in our AI solutions, enabling clients to build trust with their users.
- Accountability: There should be clear accountability for the outcomes of automated decisions. If an algorithm leads to a negative consequence, it is essential to identify who is responsible. Our consulting services help organizations establish accountability frameworks that align with their operational goals.
- Informed Consent: Users should be informed about how their data is being used. This includes obtaining consent for data collection and ensuring users understand the implications of their data being processed. Rapid Innovation assists clients in developing user-friendly consent mechanisms that comply with ethical standards.
- Privacy: Protecting user privacy is a critical ethical consideration. Organizations must implement robust data protection measures to safeguard personal information. We provide comprehensive data protection strategies that help clients secure sensitive information while maintaining compliance with regulations.
- Impact on Society: Organizations should consider the broader societal implications of technology. They should assess how their products and services affect communities and strive to promote positive social outcomes. Rapid Innovation encourages clients to evaluate the societal impact of their AI and blockchain solutions, fostering responsible innovation. For more insights, refer to our ethical AI development guide.
6.3. Bias and Fairness
Bias and fairness are significant concerns in the development and deployment of algorithms and AI systems. Addressing these issues is essential to ensure equitable outcomes for all users.
- Understanding Bias: Bias can occur at various stages, including data collection, algorithm design, and implementation. It is crucial to identify and mitigate biases to prevent discrimination against specific groups. Our team at Rapid Innovation conducts thorough assessments to identify potential biases in AI models.
- Diverse Data Sets: Using diverse and representative data sets can help reduce bias. Organizations should strive to include various demographics in their training data to ensure fair outcomes. We guide clients in curating diverse data sets that enhance the fairness of their AI applications.
- Regular Audits: Conducting regular audits of algorithms can help identify and rectify biases. Organizations should implement processes to continuously evaluate the fairness of their systems. Rapid Innovation offers auditing services that help clients maintain fairness and compliance in their AI systems.
- Stakeholder Involvement: Engaging with diverse stakeholders can provide valuable insights into potential biases. Involving community representatives can help organizations understand the impact of their technologies on different groups. We facilitate stakeholder engagement sessions to gather diverse perspectives for our clients.
- Ethical AI Frameworks: Developing and adhering to ethical AI frameworks can guide organizations in creating fair and unbiased systems. These frameworks should include guidelines for accountability, transparency, and inclusivity. Rapid Innovation assists clients in establishing ethical AI frameworks tailored to their specific needs.
6.4. Regulatory Compliance
Regulatory compliance is essential for organizations operating in data-driven environments. Adhering to regulations helps protect consumers and maintain trust in technology.
- Understanding Regulations: Organizations must stay informed about relevant regulations, such as GDPR, CCPA, and HIPAA. Understanding these laws is crucial for compliance and avoiding legal repercussions. Our experts at Rapid Innovation provide insights into regulatory landscapes to help clients navigate compliance challenges.
- Data Protection: Compliance with data protection regulations involves implementing measures to secure personal data. Organizations should have clear policies for data collection, storage, and processing. We help clients develop robust data protection policies that align with regulatory requirements.
- User Rights: Regulations often grant users specific rights regarding their data, such as the right to access, rectify, or delete their information. Organizations must ensure they have processes in place to uphold these rights. Rapid Innovation supports clients in establishing user rights management systems that enhance user trust.
- Regular Training: Providing regular training for employees on compliance and ethical standards is vital. This ensures that all team members understand their responsibilities regarding data handling and regulatory adherence. We offer tailored training programs to equip client teams with the necessary knowledge and skills.
- Monitoring and Reporting: Organizations should establish monitoring systems to track compliance with regulations. Regular reporting can help identify potential issues and demonstrate accountability to regulators and stakeholders. Rapid Innovation implements monitoring solutions that enable clients to maintain compliance and transparency in their operations.
6.5. Interpretability of AI Models
Interpretability in AI refers to the degree to which a human can understand the cause of a decision made by an AI model. As AI systems become more complex, particularly with deep learning models, the challenge of interpretability grows.
- Importance of Interpretability:
- Enhances trust in AI systems.
- Facilitates debugging and improvement of models.
- Ensures compliance with regulations, especially in sectors like finance and healthcare.
- Techniques for Improving Interpretability:
- Model-Agnostic Methods: Techniques like LIME (Local Interpretable Model-agnostic Explanations) and SHAP (SHapley Additive exPlanations) help explain predictions regardless of the model type. This is crucial for model agnostic explainable AI.
- Simpler Models: Using decision trees or linear models can provide clearer insights into decision-making processes, contributing to AI model interpretability.
- Visualization Tools: Tools that visualize model behavior can help stakeholders understand how inputs affect outputs, enhancing explainability and interpretability.
- Challenges:
- There is a trade-off between accuracy and interpretability; more complex models often yield better performance but are harder to interpret, which raises concerns about model explainability and interpretability.
- There is a lack of standardized metrics for measuring interpretability, making it difficult to assess AI interpretability and explainability.
6.6. Technical Complexity
Technical complexity in AI refers to the intricate nature of algorithms, data processing, and system integration involved in developing AI solutions.
- Factors Contributing to Technical Complexity:
- Algorithmic Sophistication: Advanced algorithms, such as neural networks, require deep understanding and expertise, which can complicate AI interpretability.
- Data Management: Handling large datasets, ensuring data quality, and preprocessing data can be daunting tasks.
- Integration with Existing Systems: Merging AI solutions with legacy systems often presents compatibility challenges.
- Implications of Technical Complexity:
- Increased development time and costs.
- Higher likelihood of errors and bugs in the system.
- Necessitates ongoing maintenance and updates to keep systems functional and relevant.
- Strategies to Manage Complexity:
- Modular Design: Breaking down systems into smaller, manageable components can simplify development and troubleshooting.
- Documentation: Comprehensive documentation aids in understanding and maintaining complex systems.
- Collaboration: Encouraging teamwork among data scientists, engineers, and domain experts can lead to more effective solutions.
6.7. Privacy Concerns
Privacy concerns in AI revolve around the collection, storage, and use of personal data. As AI systems often rely on vast amounts of data, ensuring user privacy is paramount.
- Key Privacy Issues:
- Data Collection: AI systems often require sensitive personal information, raising concerns about consent and data ownership.
- Data Breaches: The risk of unauthorized access to personal data can lead to significant repercussions for individuals and organizations.
- Surveillance: The use of AI in surveillance technologies can infringe on individual privacy rights.
- Regulatory Frameworks:
- GDPR: The General Data Protection Regulation in Europe sets strict guidelines for data protection and privacy.
- CCPA: The California Consumer Privacy Act provides consumers with rights regarding their personal data.
- Best Practices for Addressing Privacy Concerns:
- Data Minimization: Collect only the data necessary for the intended purpose.
- Anonymization: Removing personally identifiable information from datasets can help protect user privacy.
- Transparency: Clearly communicating data usage policies to users fosters trust and compliance with regulations.
At Rapid Innovation, we understand the critical importance of interpretability, technical complexity, and privacy concerns in AI development. Our expertise in these areas allows us to provide tailored solutions that not only enhance the performance of AI systems but also ensure compliance with industry regulations. By leveraging advanced techniques such as reliable and interpretable artificial intelligence and model explainability and interpretability, we help our clients achieve greater ROI while maintaining user trust and data integrity.
7. Implementation Strategies
Implementation strategies are crucial for the successful deployment of any project, particularly in data-driven environments. This section will delve into two key components: data preparation and model selection.
7.1 Data Preparation
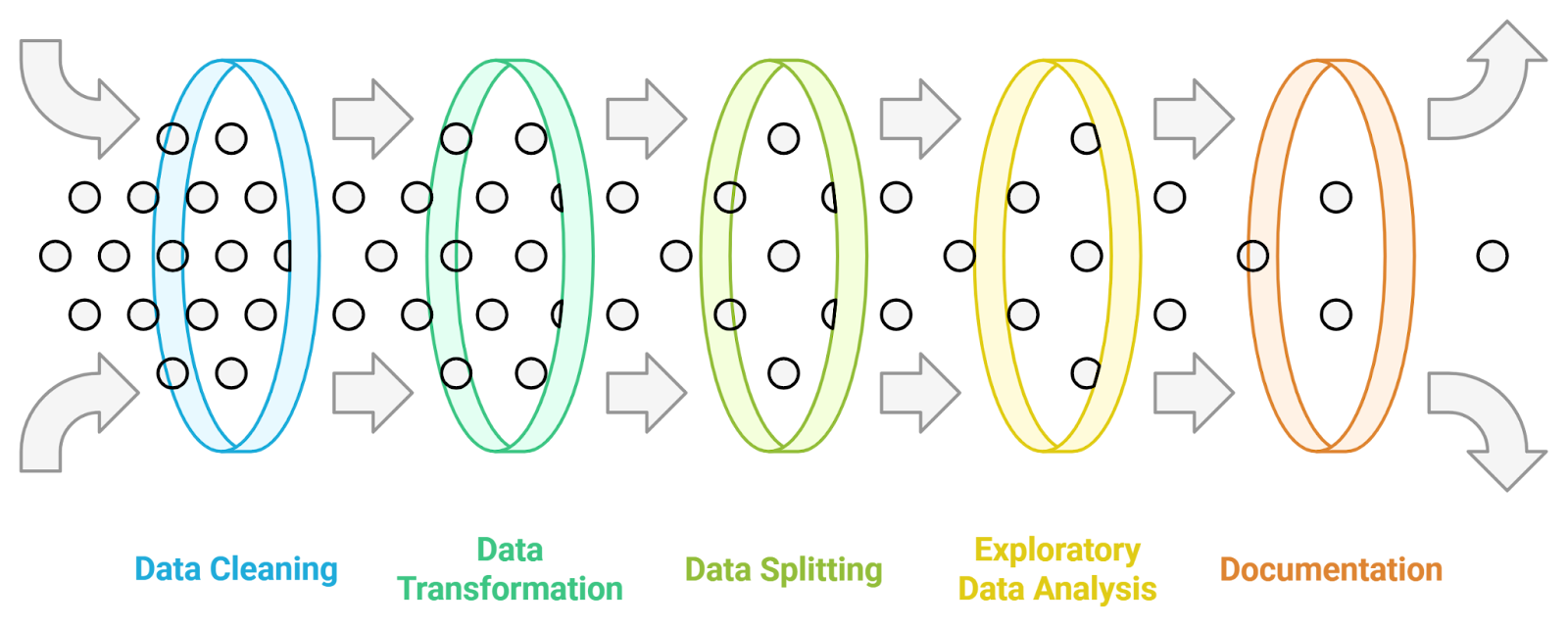
Data preparation is the foundational step in any data analysis or machine learning project. It involves cleaning, transforming, and organizing raw data into a format suitable for analysis. Effective data preparation can significantly enhance the performance of models and the accuracy of insights derived from data.
- Data Cleaning: This involves removing duplicates to ensure data integrity, handling missing values through imputation or removal, and correcting inconsistencies in data formats (e.g., date formats, categorical variables).
- Data Transformation: Normalize or standardize numerical data to bring all features to a similar scale. Encode categorical variables using techniques like one-hot encoding or label encoding. Additionally, create new features through feature engineering to enhance model performance.
- Data Splitting: Divide the dataset into training, validation, and test sets to evaluate model performance effectively. Ensure that the split maintains the distribution of the target variable to avoid bias.
- Exploratory Data Analysis (EDA): Visualize data distributions and relationships using graphs and charts. Identify patterns, trends, and outliers that may influence model selection and performance.
- Documentation: Keep detailed records of data preparation steps for reproducibility and transparency. Use version control for datasets to track changes over time.
Effective data preparation not only improves model accuracy but also saves time during the modeling phase. According to a study, data preparation can account for up to 80% of the time spent on data science projects.
7.2 Model Selection
Model selection is the process of choosing the most appropriate algorithm or model for a specific problem based on the characteristics of the data and the objectives of the analysis. The right model can lead to better predictions and insights.
- Understanding the Problem: Clearly define the problem type: classification, regression, clustering, etc. Identify the goals of the analysis, such as accuracy, interpretability, or speed.
- Algorithm Evaluation: Research various algorithms that fit the problem type, such as decision trees, support vector machines, or neural networks. Consider the trade-offs between complexity and performance; simpler models may be easier to interpret, while complex models may yield better accuracy.
- Cross-Validation: Use techniques like k-fold cross-validation to assess model performance on different subsets of the data. This helps in understanding how the model generalizes to unseen data.
- Performance Metrics: Choose appropriate metrics to evaluate model performance, such as accuracy, precision, recall, F1 score, or AUC-ROC for classification tasks. For regression tasks, consider metrics like mean absolute error (MAE) or root mean square error (RMSE).
- Hyperparameter Tuning: Optimize model parameters using techniques like grid search or random search to improve performance. Fine-tuning can significantly impact the model's effectiveness.
- Ensemble Methods: Explore ensemble techniques like bagging and boosting to combine multiple models for improved accuracy. These methods can reduce overfitting and enhance predictive performance.
Selecting the right model is critical, as it can directly influence the success of the project. Research indicates that model selection can improve predictive performance by up to 20%.
In conclusion, both data preparation and model selection are integral to the implementation strategies of any data-driven project. By focusing on these areas, organizations can enhance their analytical capabilities and drive better decision-making. At Rapid Innovation, we leverage our expertise in AI and Blockchain to guide clients through these processes, ensuring they achieve greater ROI and operational efficiency.
7.3. Training and Validation
Training and validation are critical components in the development of machine learning models, including training ai models and training in machine learning. They ensure that the model not only learns from the data but also generalizes well to unseen data.
- Training Phase: In this phase, the model learns from a training dataset, which is typically a large collection of labeled examples. The model adjusts its parameters to minimize the error in predictions, using techniques such as gradient descent. Overfitting is a common challenge during training, where the model learns the training data too well, including noise and outliers, leading to poor performance on new data. Techniques like training neural network and training machine learning models are often employed to mitigate these issues.
- Validation Phase: After training, the model is evaluated using a separate validation dataset that is not used during the training phase. The validation process helps in tuning hyperparameters, which are settings that govern the training process, such as learning rate and batch size. Cross-validation techniques, like k-fold cross-validation, are often employed to ensure that the model's performance is consistent across different subsets of data. This is particularly important in machine learning model training.
- Metrics for Evaluation: Common metrics include accuracy, precision, recall, and F1 score, which help in assessing how well the model performs. A confusion matrix can also provide insights into the types of errors the model is making, especially in machine learning training and testing scenarios.
7.4. Continuous Learning Mechanisms
Continuous learning mechanisms are essential for keeping machine learning models relevant and effective over time. As new data becomes available, models need to adapt to changes in patterns and trends, which can be facilitated through online machine learning and transfer learning deep learning techniques.
- Incremental Learning: This approach allows models to update themselves with new data without needing to retrain from scratch, making it particularly useful in dynamic environments where data is constantly changing. Techniques such as online learning and mini-batch learning are often used to implement incremental learning, including methods like xgboost training.
- Feedback Loops: Incorporating user feedback can significantly enhance model performance. By analyzing how users interact with the model's predictions, developers can identify areas for improvement. Active learning is a strategy where the model queries users for labels on uncertain predictions, thus improving its learning efficiency.
- Model Monitoring: Continuous monitoring of model performance is crucial, involving tracking metrics over time to detect any degradation in performance. Automated alerts can be set up to notify data scientists when performance drops below a certain threshold, prompting a review or retraining of the model, which may involve training a machine learning model again.
7.5. Deployment Approaches
Deployment approaches refer to the methods used to integrate machine learning models into production environments. The choice of deployment strategy can significantly impact the model's performance and usability.
- Batch Deployment: In this approach, predictions are made on a batch of data at scheduled intervals, which is suitable for applications where real-time predictions are not critical. Batch processing can be more efficient for large datasets, as it allows for resource optimization.
- Real-Time Deployment: Real-time deployment involves making predictions on individual data points as they arrive, which is essential for applications like fraud detection or recommendation systems, where immediate responses are necessary. Technologies such as REST APIs or message queues are often used to facilitate real-time predictions.
- Containerization: Using containerization technologies like Docker allows for consistent deployment across different environments, ensuring that the model runs the same way in production as it did during development. Container orchestration tools like Kubernetes can manage scaling and load balancing, making it easier to handle varying workloads.
- Model Versioning: Keeping track of different versions of models is crucial for maintaining performance and reproducibility. Versioning allows teams to roll back to previous models if a new version underperforms. Tools like MLflow or DVC can help manage model versions effectively.
- A/B Testing: A/B testing is a method used to compare two versions of a model to determine which performs better, particularly useful for optimizing user-facing applications. By randomly assigning users to different model versions, developers can gather data on performance metrics and user satisfaction.
At Rapid Innovation, we leverage these methodologies, including fine-tuning language models and mlops training, to ensure that our clients achieve greater ROI through efficient and effective AI solutions. By implementing robust training and validation processes, continuous learning mechanisms, and strategic deployment approaches, we empower businesses to harness the full potential of their data, driving innovation and growth in their respective industries.
7.6. Performance Monitoring
Performance monitoring is a critical aspect of any system, especially in the context of machine learning and artificial intelligence. It involves the continuous assessment of a model's performance to ensure it meets the desired objectives and maintains its effectiveness over time. Key components of performance monitoring include:
- Metrics: Establishing clear metrics such as accuracy, precision, recall, and F1 score to evaluate model performance.
- Data Drift Detection: Monitoring for changes in data distribution that may affect model predictions, including shifts in input features or target variables.
- Model Retraining: Setting up protocols for retraining models when performance drops below a certain threshold, ensuring they remain relevant and accurate.
- Logging and Visualization: Implementing logging systems to track model predictions and performance over time, along with visualization tools to help interpret the data.
- Feedback Loops: Creating mechanisms for incorporating user feedback to continuously improve model performance.
At Rapid Innovation, we understand that regular performance monitoring, including ml model performance monitoring, is essential for identifying issues early, allowing for timely interventions. This proactive approach not only enhances model reliability but also aids in maintaining compliance with regulatory standards, especially in industries like finance and healthcare, where model accountability is crucial. By leveraging our expertise in AI, we help clients implement robust performance monitoring systems that drive greater ROI through improved decision-making and operational efficiency.
8. Advanced AI Techniques
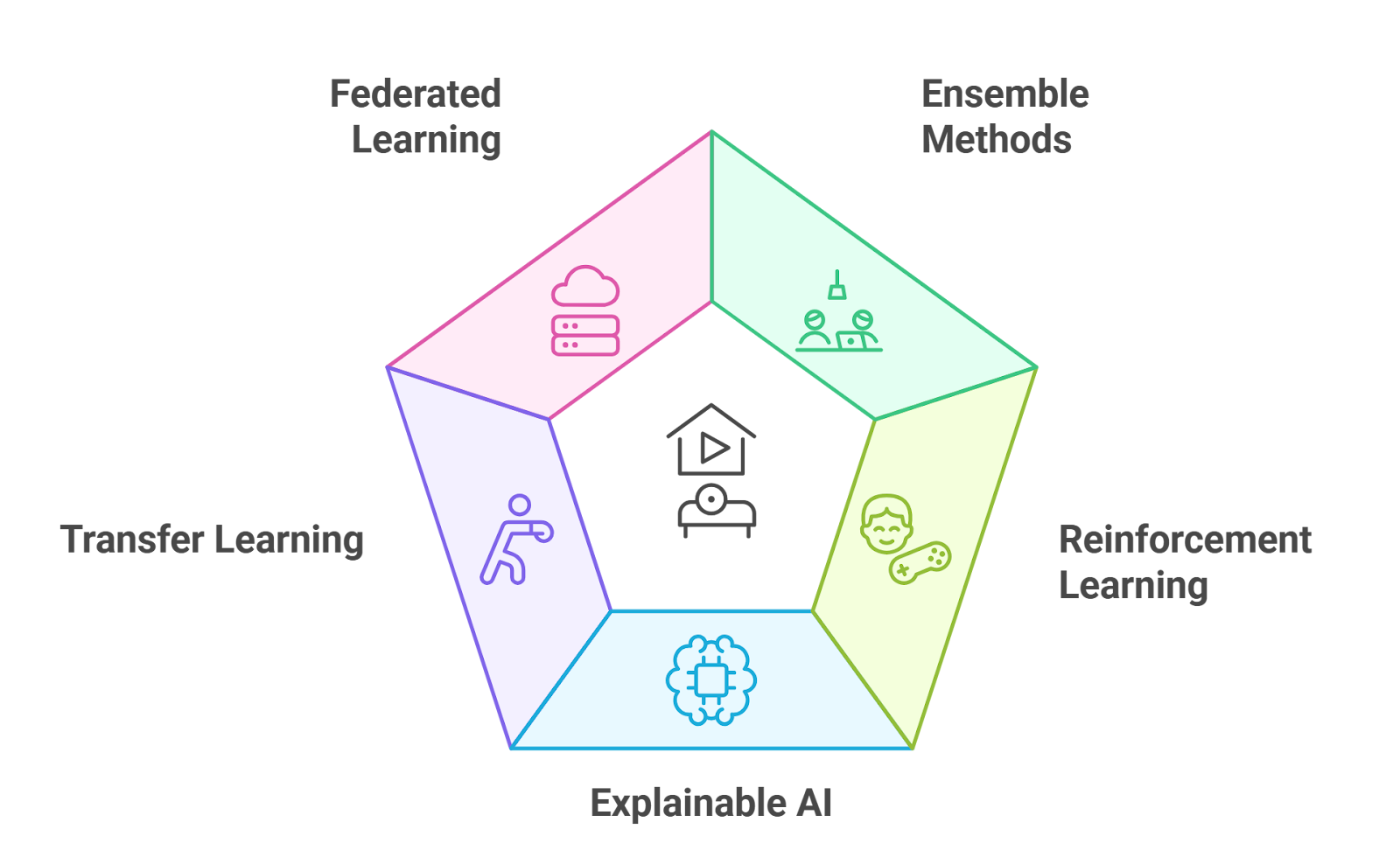
Advanced AI techniques encompass a range of sophisticated methods that enhance the capabilities of machine learning models. These techniques often lead to improved accuracy, efficiency, and robustness in various applications. Some notable advanced AI techniques include:
- Deep Learning: Utilizing neural networks with multiple layers to model complex patterns in data.
- Natural Language Processing (NLP): Techniques that enable machines to understand and interpret human language, facilitating applications like chatbots and sentiment analysis.
- Computer Vision: Methods that allow machines to interpret and make decisions based on visual data, widely used in image recognition and autonomous vehicles.
These advanced techniques are essential for tackling complex problems and driving innovation across industries. At Rapid Innovation, we harness these techniques to develop tailored solutions that align with our clients' business goals, ensuring they achieve maximum impact and return on investment.
8.1. Ensemble Methods
Ensemble methods are a powerful class of techniques in machine learning that combine multiple models to improve overall performance. By leveraging the strengths of various algorithms, ensemble methods can achieve better accuracy and robustness than individual models. Key characteristics of ensemble methods include:
- Diversity: Combining different models (e.g., decision trees, neural networks) to capture various aspects of the data.
- Aggregation: Using techniques like voting, averaging, or stacking to combine predictions from multiple models.
- Reduction of Overfitting: Ensemble methods can help mitigate overfitting by averaging out the noise from individual models.
Common ensemble methods include:
- Bagging: Techniques like Random Forests that build multiple models from random subsets of the training data and average their predictions.
- Boosting: Methods like AdaBoost and Gradient Boosting that sequentially build models, focusing on correcting the errors of previous ones.
- Stacking: Combining different models and using a meta-model to make final predictions based on their outputs.
Ensemble methods are widely used in competitions and real-world applications due to their ability to enhance predictive performance and provide more reliable results. At Rapid Innovation, we implement ensemble techniques to ensure our clients' models are not only accurate but also resilient, ultimately leading to greater business success. Additionally, we emphasize the importance of machine learning performance monitoring and performance monitoring in machine learning to maintain the effectiveness of these advanced models.
8.2. Reinforcement Learning
Reinforcement Learning (RL) is a subset of machine learning where an agent learns to make decisions by taking actions in an environment to maximize cumulative rewards. Unlike supervised learning, where the model learns from labeled data, RL focuses on learning from the consequences of actions.
- Key components of RL include:
- Agent: The learner or decision-maker.
- Environment: The context in which the agent operates.
- Actions: Choices made by the agent that affect the environment.
- Rewards: Feedback from the environment based on the agent's actions.
- RL operates on the principle of trial and error:
- The agent explores different actions to discover which ones yield the highest rewards.
- Over time, the agent learns to optimize its strategy based on past experiences.
- Applications of Reinforcement Learning:
- Robotics: Training robots to perform tasks through interaction with their environment, enhancing operational efficiency and reducing costs. This includes applications of reinforcement learning in robotics.
- Game Playing: Algorithms like AlphaGo have demonstrated RL's potential in mastering complex games, showcasing its ability to develop strategies that outperform human players. Multi agent reinforcement learning has also been explored in this domain.
- Autonomous Vehicles: RL aids in decision-making processes for navigation and obstacle avoidance, improving safety and reliability in transportation systems. Real world applications of reinforcement learning can be seen in this area.
- Challenges in Reinforcement Learning:
- Sample Efficiency: RL often requires a large number of interactions to learn effectively, which can be resource-intensive.
- Exploration vs. Exploitation: Balancing the need to explore new actions while exploiting known rewarding actions can be difficult, necessitating sophisticated strategies to optimize learning.
At Rapid Innovation, we leverage RL to help clients optimize their operations, whether it's enhancing robotic processes or improving decision-making in autonomous systems, ultimately leading to greater ROI. We also explore reinforcement learning use cases in various industries, including advanced techniques in AI agent programming.
8.3. Explainable AI Techniques
Explainable AI (XAI) refers to methods and techniques that make the outputs of AI systems understandable to humans. As AI systems become more complex, the need for transparency and interpretability grows, especially in critical applications like healthcare and finance.
- Importance of Explainable AI:
- Builds trust: Users are more likely to trust AI systems that provide clear reasoning for their decisions.
- Compliance: Regulations in various industries require transparency in decision-making processes.
- Debugging: Understanding how models make decisions can help identify and correct errors.
- Common Explainable AI Techniques:
- LIME (Local Interpretable Model-agnostic Explanations): Provides local approximations of model predictions to explain individual decisions.
- SHAP (SHapley Additive exPlanations): Uses cooperative game theory to assign importance values to each feature in a model's prediction.
- Feature Importance: Ranks features based on their contribution to the model's predictions, helping to identify which inputs are most influential.
- Applications of Explainable AI:
- Healthcare: Explaining predictions in medical diagnoses to ensure clinicians understand AI recommendations, thereby improving patient outcomes.
- Finance: Providing insights into credit scoring models to help consumers understand their scores, fostering financial literacy and trust.
- Legal: Ensuring that AI-driven decisions in the legal system are transparent and justifiable, which is crucial for maintaining fairness and accountability.
At Rapid Innovation, we implement XAI techniques to ensure that our AI solutions not only perform well but also provide clarity and transparency, enhancing user trust and compliance with industry regulations.
8.4. Transfer Learning
Transfer Learning is a machine learning technique where a model developed for a specific task is reused as the starting point for a model on a second task. This approach is particularly useful when the second task has limited data available.
- Key concepts in Transfer Learning:
- Source Task: The original task where the model is trained.
- Target Task: The new task where the model is applied.
- Pre-trained Models: Models that have been trained on large datasets and can be fine-tuned for specific applications.
- Benefits of Transfer Learning:
- Reduces training time: Leveraging pre-trained models can significantly decrease the time required to train a new model, allowing for quicker deployment of solutions.
- Improves performance: Models can achieve higher accuracy on the target task, especially when data is scarce, leading to better outcomes for clients.
- Facilitates knowledge sharing: Insights gained from one domain can enhance learning in another, promoting innovation and efficiency.
- Applications of Transfer Learning:
- Natural Language Processing: Models like BERT and GPT-3 are pre-trained on vast text corpora and can be fine-tuned for specific language tasks, improving communication and understanding in applications.
- Computer Vision: Pre-trained models on large image datasets (e.g., ImageNet) can be adapted for specific image classification tasks, enhancing visual recognition capabilities. This includes applications of deep reinforcement learning in computer vision.
- Speech Recognition: Transfer learning helps improve models by utilizing knowledge from related audio tasks, leading to more accurate and responsive systems.
- Challenges in Transfer Learning:
- Domain Shift: Differences between the source and target tasks can hinder performance, requiring careful management of model adaptation.
- Overfitting: Fine-tuning a pre-trained model on a small dataset may lead to overfitting if not managed properly, necessitating robust validation techniques.
At Rapid Innovation, we harness the power of Transfer Learning to accelerate the development of AI solutions, ensuring that our clients can achieve their business goals efficiently and effectively while maximizing their return on investment. We also explore applications of reinforcement learning in machine learning to enhance our solutions.
8.5. Federated Learning Approaches
Federated learning is a decentralized machine learning approach that allows multiple parties to collaboratively train a model while keeping their data localized. This method addresses privacy concerns and reduces the need for data transfer, making it a popular choice in various industries.
- Data Privacy: Federated learning ensures that sensitive data remains on local devices, minimizing the risk of data breaches. This is particularly important in sectors like healthcare and finance, where data privacy is paramount. For example, federated learning in healthcare can help protect patient data while still enabling valuable insights.
- Reduced Latency: By processing data locally, federated learning can significantly reduce latency. This is beneficial for applications requiring real-time decision-making, such as autonomous vehicles and smart devices. Techniques like asynchronous federated learning can further enhance this aspect.
- Scalability: Federated learning can easily scale to accommodate a growing number of devices. As more participants join the network, the model can be updated without the need for centralized data storage. This is particularly relevant in vertical federated learning scenarios where different parties hold different features of the same data.
- Personalization: This approach allows for personalized models that can adapt to individual user preferences while still benefiting from the collective knowledge of the group. For instance, personalized recommendations in e-commerce can be enhanced through federated learning, including methods like personalized federated learning.
- Resource Efficiency: Federated learning can optimize resource usage by leveraging the computational power of local devices. This reduces the burden on central servers and can lead to cost savings. Frameworks like TensorFlow Federated and PyTorch federated learning facilitate this optimization.
- Challenges: Despite its advantages, federated learning faces challenges such as communication overhead, model convergence issues, and the need for robust security measures to protect against adversarial attacks. Techniques like federated averaging and scaffold federated learning are being explored to address these challenges.
9. Economic and Strategic Implications
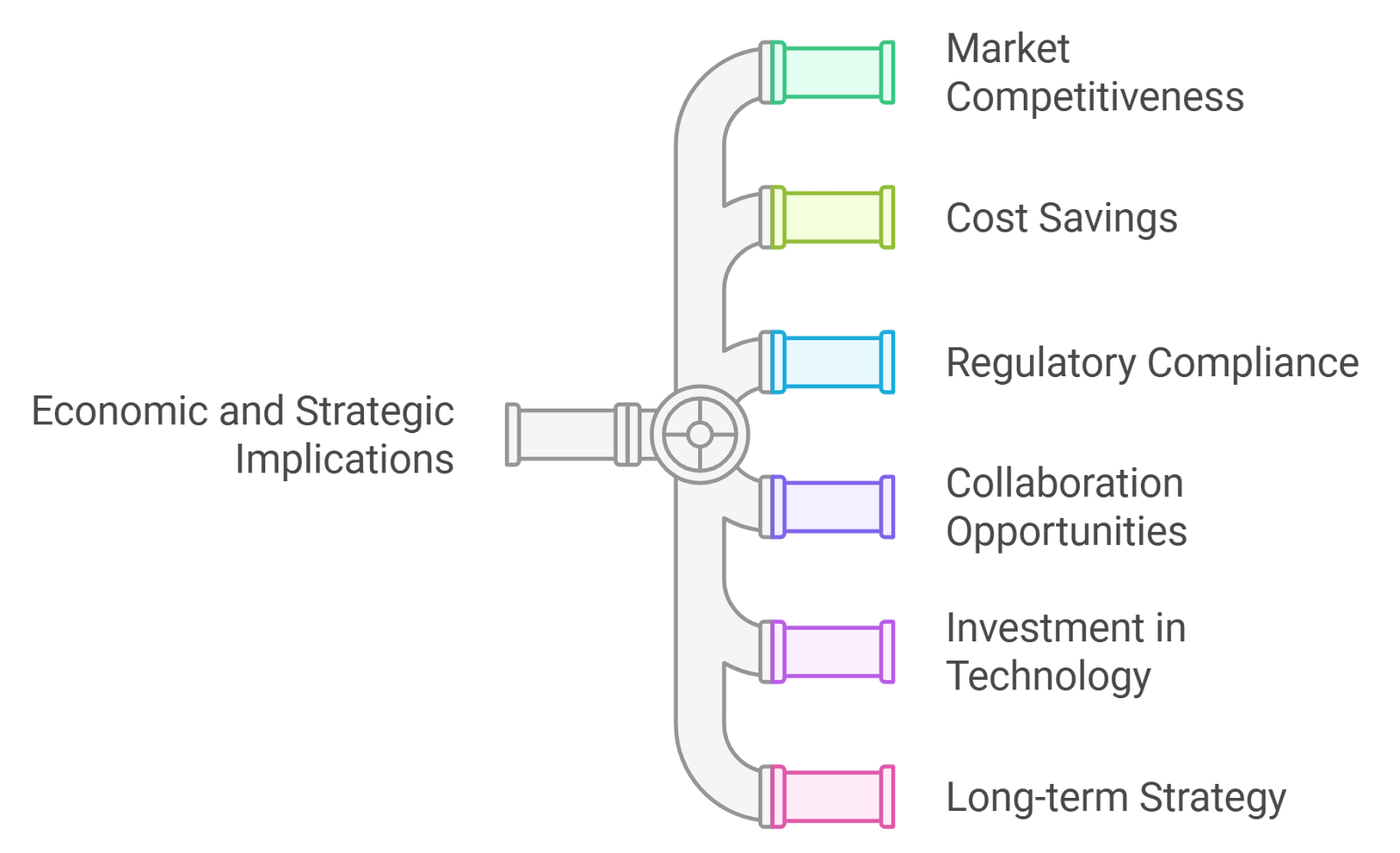
The economic and strategic implications of federated learning are significant, particularly as organizations seek to leverage data while maintaining compliance with privacy regulations. The following points highlight key considerations:
- Market Competitiveness: Organizations that adopt federated learning can gain a competitive edge by utilizing data more effectively while adhering to privacy standards. This can lead to improved products and services, especially in sectors like federated AI and federated machine learning.
- Cost Savings: By reducing the need for centralized data storage and processing, federated learning can lead to substantial cost savings. Organizations can allocate resources more efficiently, focusing on innovation rather than infrastructure.
- Regulatory Compliance: With increasing regulations around data privacy, federated learning offers a compliant way to utilize data. This can mitigate legal risks and enhance an organization’s reputation, particularly in industries like healthcare where federated learning in healthcare is becoming essential.
- Collaboration Opportunities: Federated learning encourages collaboration among organizations that may have previously been hesitant to share data. This can lead to new partnerships and innovative solutions, such as federated class incremental learning.
- Investment in Technology: Companies may need to invest in new technologies and infrastructure to implement federated learning effectively. This includes developing secure communication protocols and ensuring compatibility with existing systems, which is crucial for decentralized federated learning.
- Long-term Strategy: Organizations should consider federated learning as part of their long-term data strategy. As the landscape of data privacy continues to evolve, adopting this approach can position companies for future success.
9.1. Cost-Benefit Analysis
Conducting a cost-benefit analysis for federated learning is essential for organizations considering its implementation. This analysis helps in understanding the financial implications and potential returns on investment.
- Initial Costs:
- Investment in technology and infrastructure to support federated learning.
- Training staff to understand and implement federated learning protocols.
- Operational Costs:
- Ongoing maintenance of the federated learning system.
- Costs associated with ensuring data security and compliance with regulations.
- Potential Benefits:
- Enhanced data privacy leading to increased customer trust and loyalty.
- Improved model performance through diverse data sources without compromising privacy.
- Cost savings from reduced data transfer and storage needs.
- Return on Investment (ROI):
- Organizations can calculate ROI by comparing the potential benefits against the initial and operational costs.
- A positive ROI indicates that the benefits of implementing federated learning outweigh the costs, making it a viable option.
- Risk Assessment:
- Evaluate the risks associated with federated learning, including potential data breaches and the challenges of model convergence.
- Consider the impact of these risks on the overall cost-benefit analysis.
- Long-term Value:
- Assess the long-term value of federated learning in terms of market positioning and compliance with evolving data regulations.
- Organizations that invest in federated learning may find themselves better equipped to adapt to future challenges in data management.
By carefully analyzing these factors, organizations can make informed decisions about adopting federated learning and its economic implications. At Rapid Innovation, we specialize in guiding organizations through this process, ensuring that they can leverage federated learning to achieve their business goals efficiently and effectively. Our expertise in AI and blockchain technologies positions us to help clients maximize their ROI while navigating the complexities of data privacy and compliance. For more information on our services, visit our AI EdTech Solutions.
9.2. Competitive Advantage
Competitive advantage refers to the unique attributes or capabilities that allow a business to outperform its competitors. It is essential for long-term success and sustainability in any industry.
- Unique Selling Proposition (USP): A clear USP differentiates a brand from its competitors, which could be through superior quality, innovative features, or exceptional customer service. At Rapid Innovation, we help clients define and refine their USP by leveraging AI-driven market analysis to identify gaps and opportunities in their offerings.
- Cost Leadership: Companies can achieve competitive advantage by being the lowest-cost producer in their industry, allowing them to offer lower prices or maintain higher margins. Our blockchain solutions enable clients to streamline operations and reduce costs through enhanced transparency and efficiency in supply chain management. This aligns with the principles of a cost leadership strategy, which is a key component of a sustainable competitive strategy.
- Brand Loyalty: Strong brand recognition and customer loyalty can create a competitive edge. Businesses that invest in building a reputable brand often enjoy repeat customers and referrals. Rapid Innovation employs AI tools to analyze customer sentiment and engagement, helping clients foster deeper connections with their audience.
- Innovation: Continuous innovation in products or services can set a company apart. This includes adopting new technologies or improving existing offerings to meet changing consumer needs. Our team specializes in integrating cutting-edge AI and blockchain technologies, ensuring that our clients remain at the forefront of their industries. Companies like Amazon exemplify competitive strategy through innovation.
- Market Positioning: Effective market positioning helps businesses target specific customer segments, making it easier to cater to their preferences and needs. We assist clients in utilizing data analytics to refine their market strategies, ensuring they reach the right audience with the right message. This is particularly important in competitive strategy and competitive advantage discussions.
9.3. Revenue Optimization
Revenue optimization involves strategies and practices aimed at maximizing a company's income from its products or services. It is crucial for enhancing profitability and ensuring sustainable growth.
- Pricing Strategies: Implementing dynamic pricing, value-based pricing, or discount strategies can help optimize revenue. Understanding customer willingness to pay is key. Our AI algorithms analyze market trends and consumer behavior to recommend optimal pricing strategies.
- Upselling and Cross-selling: Encouraging customers to purchase additional or higher-value products can significantly increase revenue. Training sales teams to identify opportunities is essential. We provide AI-driven insights that empower sales teams to effectively upsell and cross-sell based on customer preferences.
- Customer Segmentation: Analyzing customer data to segment audiences allows businesses to tailor marketing efforts and pricing strategies, leading to higher conversion rates. Rapid Innovation utilizes advanced analytics to help clients identify and target high-value customer segments.
- Performance Metrics: Regularly tracking key performance indicators (KPIs) such as average revenue per user (ARPU) and customer lifetime value (CLV) helps identify areas for improvement. Our solutions include dashboards that provide real-time insights into performance metrics, enabling data-driven decision-making.
- Technology Utilization: Leveraging technology, such as revenue management software, can streamline processes and provide insights for better decision-making. We integrate AI and blockchain technologies to enhance revenue management capabilities, ensuring our clients maximize their income potential.
9.4. Risk Management
Risk management is the process of identifying, assessing, and mitigating potential risks that could negatively impact a business. Effective risk management is vital for ensuring stability and long-term success.
- Risk Identification: Recognizing potential risks, including financial, operational, and reputational risks, is the first step in risk management. This can be achieved through regular audits and assessments. Our AI tools assist in identifying risks by analyzing vast amounts of data for anomalies and trends.
- Risk Assessment: Evaluating the likelihood and impact of identified risks helps prioritize which risks need immediate attention. This can involve qualitative and quantitative analysis. We provide clients with comprehensive risk assessment frameworks that leverage data analytics for informed decision-making.
- Mitigation Strategies: Developing strategies to minimize or eliminate risks is crucial. This may include diversifying suppliers, investing in insurance, or implementing robust cybersecurity measures. Our blockchain solutions enhance security and transparency, significantly reducing operational risks.
- Monitoring and Review: Continuous monitoring of risk factors and regular reviews of risk management strategies ensure that businesses remain prepared for emerging threats. We offer ongoing support and analytics to help clients adapt their risk management strategies in real-time.
- Employee Training: Educating employees about risk management practices fosters a culture of awareness and preparedness, reducing the likelihood of risks materializing. Rapid Innovation provides tailored training programs that incorporate AI insights, ensuring employees are equipped to handle potential risks effectively.
9.5. Investment Considerations
When evaluating investment opportunities, several key factors must be taken into account to ensure a sound decision. These considerations can significantly impact the potential return on investment (ROI) and overall risk profile.
- Market Research: Understanding the market landscape is crucial. This includes analyzing trends, consumer behavior, and competitive dynamics. A thorough market analysis can help identify growth opportunities and potential threats. At Rapid Innovation, we leverage AI-driven analytics to provide clients with deep insights into market trends, enabling them to make data-informed investment decisions.
- Risk Assessment: Every investment carries inherent risks. Investors should assess both systematic risks (market-wide) and unsystematic risks (specific to the investment). Tools like SWOT analysis can help in identifying strengths, weaknesses, opportunities, and threats. Our blockchain solutions can enhance transparency and traceability, allowing clients to better assess and manage risks associated with their investments.
- Financial Health: Evaluating the financial statements of a potential investment is essential. Key metrics to consider include revenue growth, profit margins, debt levels, and cash flow. Rapid Innovation employs AI algorithms to analyze financial data, providing clients with predictive insights that can lead to more informed investment choices.
- Diversification: Spreading investments across various asset classes can mitigate risk. A diversified portfolio can help cushion against market volatility and reduce the impact of poor-performing investments. Our consulting services guide clients in creating diversified portfolios that leverage both AI and blockchain technologies for optimal performance.
- Time Horizon: The investment time frame can influence the choice of assets. Long-term investments may tolerate more volatility, while short-term investments require more stability. We assist clients in aligning their investment strategies with their specific time horizons, ensuring they achieve their financial objectives.
- Exit Strategy: Having a clear exit strategy is vital. Investors should determine how and when they plan to liquidate their investments, whether through selling, merging, or other means. Our expertise in blockchain technology can facilitate smoother exit processes through smart contracts, ensuring that transactions are executed efficiently and transparently.
- Regulatory Environment: Understanding the regulatory landscape is crucial. Compliance with laws and regulations can affect the viability of an investment. Rapid Innovation stays abreast of regulatory changes in the AI and blockchain sectors, providing clients with the necessary guidance to navigate compliance challenges effectively.
- Ethical Considerations: Investors should consider the ethical implications of their investments. This includes evaluating the social and environmental impact of the business practices of potential investments. We promote ethical investing by helping clients identify opportunities that align with their values, utilizing AI to assess the social impact of potential investments. This is particularly relevant in the context of esg considerations and esg investment considerations, which are increasingly important for investors today.
- Investment Considerations for Specific Sectors: Different sectors may have unique investment considerations. For instance, esg considerations for private equity firms and mlp etfs require careful consideration due to their specific regulatory and operational frameworks. Additionally, reit tax considerations can significantly impact the overall return on investment in real estate sectors.
- Family Investment Considerations: For mature families, investment considerations may include succession planning and wealth preservation strategies. Key investment considerations should align with the family's long-term financial goals and values.
10. Regulatory and Ethical Framework
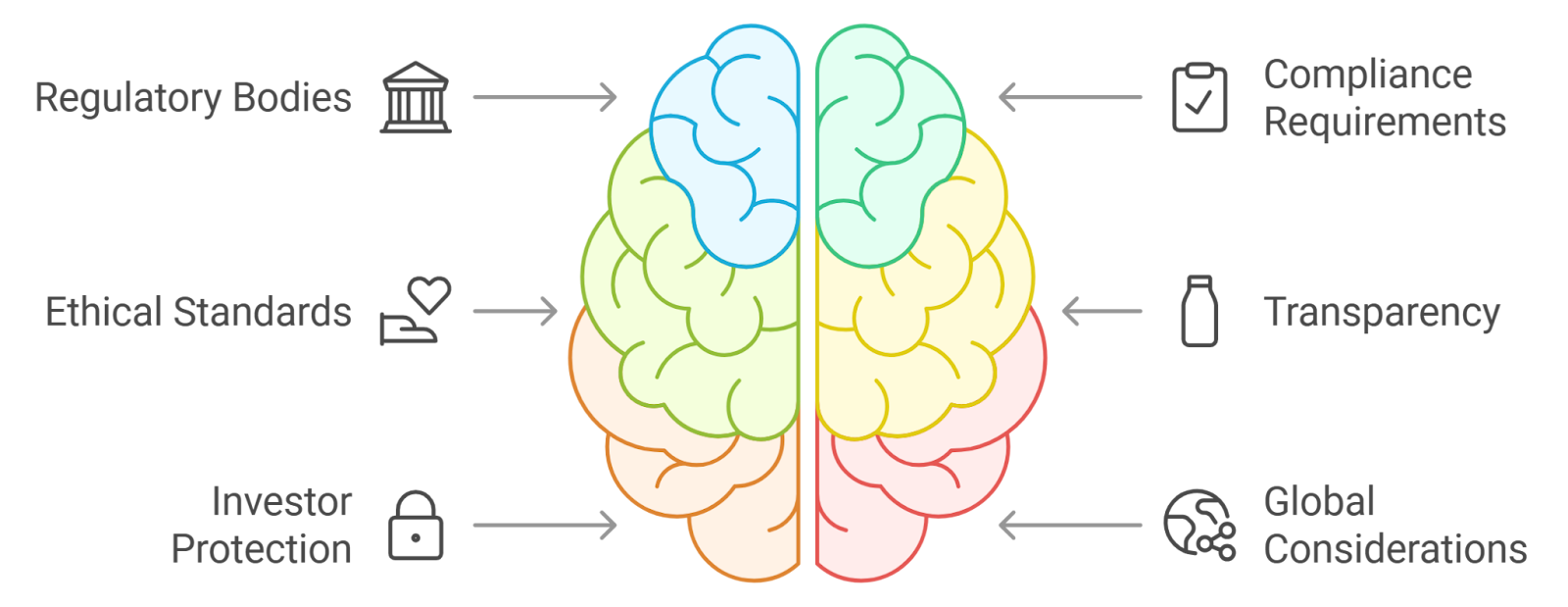
The regulatory and ethical framework surrounding investments is essential for maintaining market integrity and protecting investors. This framework encompasses laws, regulations, and ethical standards that govern investment practices.
- Regulatory Bodies: Various regulatory agencies oversee investment activities. In the U.S., the Securities and Exchange Commission (SEC) plays a pivotal role in enforcing securities laws and protecting investors.
- Compliance Requirements: Companies must adhere to specific compliance requirements, including the disclosure of financial information, adherence to anti-fraud regulations, and compliance with insider trading laws.
- Ethical Standards: Ethical investing has gained traction, with many investors seeking to align their portfolios with their values. This includes socially responsible investing (SRI), environmental, social, and governance (ESG) criteria, and impact investing.
- Transparency: A commitment to transparency is vital for building trust with investors. Companies should provide clear and accurate information regarding their operations and financial health.
- Investor Protection: Regulatory frameworks are designed to protect investors from fraud and malpractice. This includes mechanisms for reporting violations and seeking redress.
- Global Considerations: As investments become increasingly global, understanding international regulations is crucial. Different countries have varying laws that can impact cross-border investments.
10.1. Legal Compliance
Legal compliance is a fundamental aspect of the regulatory framework that governs investments. It ensures that companies and investors operate within the law, thereby minimizing legal risks and enhancing credibility.
- Understanding Laws: Investors must familiarize themselves with relevant laws, including securities laws, tax regulations, and employment laws.
- Due Diligence: Conducting thorough due diligence is essential before making any investment. This involves reviewing legal documents, assessing potential liabilities, and ensuring compliance with local and international laws.
- Contractual Obligations: Investors should be aware of their contractual obligations. This includes understanding the terms of investment agreements and any associated risks.
- Regulatory Filings: Companies are often required to file reports with regulatory bodies. Investors should monitor these filings to stay informed about any changes that may affect their investments.
- Legal Counsel: Engaging legal counsel can provide valuable insights into compliance issues. Legal experts can help navigate complex regulations and ensure adherence to laws.
- Penalties for Non-Compliance: Failing to comply with legal requirements can result in severe penalties, including fines and legal action. Understanding these risks is crucial for investors.
- Continuous Monitoring: Legal compliance is not a one-time effort. Ongoing monitoring of regulatory changes and compliance requirements is necessary to mitigate risks and ensure adherence to the law.
By considering these investment factors and understanding the regulatory and ethical framework, investors can make informed decisions that align with their financial goals and values. Rapid Innovation is committed to supporting clients in navigating these complexities, ensuring they achieve greater ROI through informed and strategic investment practices. For tailored solutions, explore our AI banking solutions.
10.2. Fairness and Non-Discrimination
Fairness and non-discrimination are critical principles in the development and deployment of artificial intelligence (AI) systems. These principles ensure that AI technologies do not perpetuate biases or inequalities, which can lead to harmful consequences for individuals and communities. At Rapid Innovation, we prioritize these principles, including fairness accountability transparency and ethics in ai, to help our clients achieve their business goals efficiently and effectively.
- AI systems must be designed to treat all individuals equitably, regardless of race, gender, age, or other characteristics. Our team works closely with clients to create AI solutions that are inclusive and fair, enhancing their brand reputation and customer loyalty.
- Developers should conduct thorough bias assessments during the AI training process to identify and mitigate any potential biases in the data. We implement robust testing protocols to ensure that our AI models are trained on diverse datasets, leading to more accurate and reliable outcomes.
- Regular audits and evaluations of AI systems can help ensure ongoing fairness and non-discrimination. Rapid Innovation offers continuous monitoring services that allow clients to maintain compliance with fairness standards, ultimately reducing the risk of costly legal issues.
- Stakeholder engagement is essential; involving diverse groups in the development process can provide insights into potential biases and their impacts. We facilitate workshops and focus groups to gather feedback, ensuring that our AI solutions meet the needs of all stakeholders.
- Regulatory frameworks may be necessary to enforce fairness standards and hold organizations accountable for discriminatory practices. Our consulting services include guidance on navigating these regulations, helping clients stay ahead of compliance requirements.
10.3. Transparency Requirements
Transparency in AI systems is vital for building trust and accountability. It involves making the workings of AI systems understandable to users and stakeholders, which can help demystify how decisions are made. At Rapid Innovation, we emphasize transparency to enhance client relationships and foster user confidence, aligning with the principles of ai fairness and transparency.
- Clear documentation of AI algorithms and data sources is essential for transparency. We provide comprehensive documentation for all AI projects, ensuring that clients can easily understand and explain their systems.
- Organizations should provide explanations for AI-driven decisions, especially in high-stakes areas like healthcare, finance, and law enforcement. Our solutions include user-friendly interfaces that allow clients to communicate AI decision-making processes clearly.
- Users should have access to information about how their data is used and the criteria that influence AI outcomes. We help clients implement data governance frameworks that prioritize user privacy and data security.
- Transparency can be enhanced through the use of explainable AI (XAI) techniques, which aim to make AI decision-making processes more interpretable. Rapid Innovation integrates XAI methodologies into our AI solutions, enabling clients to provide clear explanations to their users.
- Regular reporting on AI system performance and any changes made to algorithms can foster a culture of openness. We offer analytics and reporting tools that keep clients informed about their AI systems' performance, ensuring accountability.
10.4. Ethical AI Guidelines
Ethical AI guidelines are frameworks that help organizations navigate the complex moral landscape of AI development and deployment. These guidelines aim to ensure that AI technologies are used responsibly and for the benefit of society. Rapid Innovation is committed to helping clients establish and adhere to ethical AI practices, including fate fairness accountability transparency and ethics in ai.
- Ethical guidelines should prioritize human rights, ensuring that AI systems respect individual dignity and freedom. We assist clients in developing ethical frameworks that align with their corporate values and societal expectations.
- Organizations should establish clear ethical principles that guide AI development, such as accountability, fairness, and respect for privacy. Our consulting services include the creation of tailored ethical guidelines that reflect the unique needs of each client.
- Continuous ethical training for AI developers and stakeholders can promote a culture of responsibility and awareness of potential ethical dilemmas. We provide training programs that equip teams with the knowledge to navigate ethical challenges in AI development.
- Collaboration with ethicists, sociologists, and other experts can provide diverse perspectives on the implications of AI technologies. Rapid Innovation fosters partnerships with experts to ensure that our clients benefit from a wide range of insights.
- Organizations should be prepared to adapt their ethical guidelines in response to new challenges and societal expectations as AI technology evolves. We help clients stay agile by regularly reviewing and updating their ethical frameworks in line with industry advancements.
By integrating these principles into our AI and Blockchain solutions, Rapid Innovation empowers clients to achieve greater ROI while maintaining ethical standards and fostering trust in their technologies. For more information on our services, visit our AI consulting company.
10.5. Global Regulatory Landscape
The global regulatory landscape is a complex framework that governs various industries, including finance, healthcare, technology, and environmental sectors. Understanding this landscape is crucial for businesses operating internationally, as regulations can significantly impact operations, compliance, and market entry strategies.
- Regulatory bodies vary by region, with organizations like the European Union (EU), the U.S. Securities and Exchange Commission (SEC), and the International Organization for Standardization (ISO) playing pivotal roles.
- Compliance with regulations is essential for maintaining market integrity and consumer trust. Non-compliance can lead to severe penalties, including fines and reputational damage.
- The rise of digital technologies has prompted regulators to adapt existing frameworks or create new regulations. This includes data protection laws like the General Data Protection Regulation (GDPR) in the EU, which sets stringent guidelines for data privacy.
- Environmental regulations are becoming increasingly important as governments worldwide focus on sustainability. Initiatives like the Paris Agreement aim to regulate carbon emissions and promote renewable energy sources.
- The regulatory landscape is also influenced by geopolitical factors, such as trade agreements and tensions, which can lead to changes in tariffs and import/export regulations.
- Companies must stay informed about regulatory changes to ensure compliance and avoid disruptions. This often involves investing in legal expertise and compliance programs.
At Rapid Innovation, we understand the intricacies of the global regulatory landscape and offer tailored consulting solutions to help businesses navigate these complexities. Our expertise in AI and Blockchain can assist clients in automating compliance processes, ensuring adherence to regulations, and minimizing risks associated with non-compliance. Additionally, we provide insights on AI in financial regulatory compliance to help organizations stay ahead in this evolving landscape.
11. Future Trends and Outlook

The future of various industries is shaped by emerging trends that reflect technological advancements, changing consumer behaviors, and evolving regulatory frameworks. Understanding these trends is vital for businesses to remain competitive and innovative.
- Digital transformation continues to accelerate, with businesses adopting cloud computing, artificial intelligence (AI), and big data analytics to enhance efficiency and decision-making.
- Sustainability is becoming a core focus for companies, driven by consumer demand for environmentally friendly products and practices. This trend is leading to increased investment in green technologies and sustainable supply chains.
- Remote work and flexible working arrangements are reshaping workplace dynamics. Companies are investing in digital collaboration tools to support a distributed workforce.
- The rise of e-commerce is transforming retail, with businesses increasingly adopting omnichannel strategies to meet consumer expectations for convenience and accessibility.
- Health and wellness trends are influencing product development across various sectors, from food and beverage to personal care, as consumers prioritize their well-being.
11.1. Emerging Technologies
Emerging technologies are at the forefront of innovation, driving change across industries and creating new opportunities for businesses. Understanding these technologies is essential for organizations looking to leverage their potential.
- Artificial Intelligence (AI) is revolutionizing industries by enabling automation, enhancing customer experiences, and providing data-driven insights. AI applications range from chatbots in customer service to predictive analytics in supply chain management.
- Blockchain technology is gaining traction for its ability to provide secure, transparent transactions. It is being utilized in finance, supply chain management, and even healthcare for secure patient data sharing.
- Internet of Things (IoT) devices are becoming increasingly prevalent, connecting everyday objects to the internet. This technology enables real-time data collection and analysis, leading to improved operational efficiency and smarter decision-making.
- Augmented Reality (AR) and Virtual Reality (VR) are transforming customer experiences, particularly in retail and entertainment. These technologies allow consumers to interact with products in immersive ways, enhancing engagement and satisfaction.
- 5G technology is set to revolutionize connectivity, offering faster data speeds and lower latency. This advancement will enable the proliferation of IoT devices and enhance mobile experiences for consumers.
By staying abreast of these emerging technologies, businesses can position themselves for success in an ever-evolving landscape. At Rapid Innovation, we leverage our expertise in AI and Blockchain to help clients harness these technologies, driving innovation and achieving greater ROI.
11.2. Advanced Predictive Capabilities
Advanced predictive capabilities refer to the ability of systems to analyze vast amounts of data and forecast future trends or behaviors. This is increasingly important in various sectors, including finance, healthcare, and marketing. Organizations are leveraging advanced predictive analytics, such as predictive data analytics and prescriptive analytics, to stay competitive. For instance, in retail, companies can predict inventory needs based on seasonal trends, leading to reduced waste and increased sales. According to a report by McKinsey, businesses that effectively use predictive analytics can increase their profitability by 5-10%.
- Utilizes machine learning algorithms to identify patterns in historical data, enabling Rapid Innovation to develop tailored solutions that enhance operational efficiency through predictive modeling.
- Enhances decision-making processes by providing actionable insights, allowing clients to make informed choices that align with their strategic goals, including prescriptive analysis.
- Improves customer experience by anticipating needs and preferences, which can lead to increased customer satisfaction and loyalty, particularly in predictive analytics in healthcare.
- Enables businesses to optimize operations and reduce costs through better resource allocation, ultimately driving higher ROI, as seen in power bi predictive analytics.
- Supports risk management by predicting potential challenges before they arise, helping organizations mitigate risks proactively, especially in predictive analytics and healthcare.
11.3. Quantum Computing Integration
Quantum computing integration represents a significant leap in computational power, allowing for complex problem-solving that classical computers struggle with. This technology is still in its infancy but holds immense potential across various industries. As quantum computing continues to evolve, its integration into existing systems will likely transform industries. Companies like IBM and Google are at the forefront of this technology, exploring its applications in finance, logistics, and healthcare. The potential economic impact of quantum computing could reach trillions of dollars in the coming decades.
- Processes information at unprecedented speeds, enabling real-time data analysis that can enhance decision-making for Rapid Innovation's clients.
- Solves complex optimization problems that are currently infeasible for traditional computers, providing clients with innovative solutions to intricate challenges.
- Enhances cryptography, making data security more robust, which is crucial for businesses operating in sensitive sectors.
- Facilitates breakthroughs in drug discovery and materials science by simulating molecular interactions, showcasing the transformative potential of quantum computing in healthcare.
- Promises to revolutionize artificial intelligence by improving machine learning algorithms, further enhancing the capabilities of Rapid Innovation's AI solutions.
11.4. Hyper-Personalization
Hyper-personalization takes personalization to the next level by using real-time data and advanced analytics to tailor experiences to individual users. This approach is becoming increasingly vital in marketing and customer service. Brands that adopt hyper-personalization can significantly improve their customer relationships. For example, Netflix uses hyper-personalization to recommend shows based on viewing history, resulting in higher user retention rates. According to a study by Epsilon, 80% of consumers are more likely to make a purchase when brands offer personalized experiences.
- Leverages AI and machine learning to analyze user behavior and preferences, enabling Rapid Innovation to create customized solutions for clients.
- Delivers customized content, product recommendations, and services based on individual needs, enhancing client engagement and satisfaction.
- Increases customer engagement and loyalty by creating a more relevant experience, which can lead to improved business outcomes.
- Utilizes data from multiple sources, including social media, browsing history, and purchase behavior, to provide comprehensive insights for clients.
- Enhances marketing effectiveness by targeting specific segments with precision, ultimately driving higher conversion rates and ROI for businesses, including the use of predictive analytics with power bi.
11.5. Predictive Intelligence Evolution
Predictive intelligence has undergone significant evolution over the years, transforming how businesses leverage data to make informed decisions. This evolution can be attributed to advancements in technology, data analytics, and machine learning.
- Enhanced Data Collection:
- The rise of big data has enabled organizations to collect vast amounts of information from various sources, including social media, IoT devices, and customer interactions. Improved data collection methods have led to more accurate and comprehensive datasets.
- Advanced Algorithms:
- Machine learning algorithms have become more sophisticated, allowing for better pattern recognition and predictive modeling. Techniques such as deep learning and neural networks have enhanced the ability to analyze complex datasets.
- Real-time Analytics:
- Businesses can now access real-time data, enabling them to make quicker decisions based on current trends and behaviors. This immediacy allows for proactive strategies rather than reactive ones.
- Integration with AI:
- The integration of artificial intelligence with predictive analytics has led to more intelligent systems that can learn and adapt over time. AI-driven predictive models can provide insights that were previously unattainable, allowing organizations to optimize their operations and enhance customer experiences.
- Industry Applications:
- Predictive intelligence is being utilized across various sectors, including healthcare, finance, retail, and manufacturing. For example, in healthcare, predictive analytics can forecast patient admissions, while in retail, it can optimize inventory management. Rapid Innovation has successfully implemented predictive analytics implementation solutions for clients in these industries, resulting in improved operational efficiency and increased ROI.
- Ethical Considerations:
- As predictive intelligence evolves, ethical considerations regarding data privacy and bias in algorithms have come to the forefront. Organizations must ensure that their predictive models are transparent and fair to avoid discrimination, a principle that Rapid Innovation upholds in all its projects.
12. Implementation Roadmap

An effective implementation roadmap is crucial for organizations looking to adopt predictive intelligence. This roadmap outlines the steps necessary to integrate predictive analytics into business processes successfully.
- Define Objectives:
- Clearly outline the goals of implementing predictive intelligence and identify specific business problems that predictive analytics can address.
- Assess Current Capabilities:
- Evaluate existing data infrastructure, tools, and analytics capabilities to determine the readiness of the organization to adopt predictive intelligence.
- Data Strategy Development:
- Create a comprehensive data strategy that includes data collection, storage, and management. Ensure data quality and integrity to support accurate predictive modeling.
- Technology Selection:
- Choose the right tools and technologies that align with the organization’s needs. Consider cloud-based solutions for scalability and flexibility.
- Build a Skilled Team:
- Assemble a team with the necessary skills in data science, analytics, and domain expertise. Provide training and resources to enhance the team's capabilities.
- Pilot Projects:
- Start with pilot projects to test predictive models and assess their effectiveness. Use the insights gained from pilot projects to refine strategies and approaches.
- Full-scale Implementation:
- Once pilot projects are successful, roll out predictive intelligence across the organization. Ensure that all stakeholders are involved and informed throughout the process.
- Monitor and Optimize:
- Continuously monitor the performance of predictive models and analytics processes. Make adjustments as needed to improve accuracy and effectiveness.
12.1. Initial Assessment
The initial assessment is a critical step in the implementation roadmap for predictive intelligence. This phase involves evaluating the current state of the organization’s data and analytics capabilities.
- Data Inventory:
- Conduct a thorough inventory of existing data sources, including structured and unstructured data. Identify gaps in data that may hinder predictive analytics efforts.
- Stakeholder Engagement:
- Engage key stakeholders to understand their needs and expectations regarding predictive intelligence. Gather insights on how predictive analytics can support their specific business functions.
- Current Technology Assessment:
- Review the existing technology stack to determine its compatibility with predictive analytics tools. Identify any necessary upgrades or new technologies required for implementation.
- Skills Gap Analysis:
- Assess the current skill levels of team members in data analytics and predictive modeling. Identify training needs to ensure the team is equipped to handle predictive intelligence projects.
- Business Process Evaluation:
- Analyze current business processes to identify areas where predictive intelligence can add value. Determine how predictive analytics can enhance decision-making and operational efficiency.
- Risk Assessment:
- Evaluate potential risks associated with implementing predictive intelligence, including data privacy concerns and algorithm bias. Develop strategies to mitigate these risks.
- Feasibility Study:
- Conduct a feasibility study to assess the practicality of implementing predictive intelligence within the organization. Consider factors such as budget, resources, and timeline.
By conducting a thorough initial assessment, organizations can lay a solid foundation for successful predictive intelligence implementation, ensuring that they are well-prepared to leverage data-driven insights for strategic decision-making. Rapid Innovation is committed to guiding clients through this process, ensuring they achieve greater ROI through effective predictive analytics implementation solutions.
12.2. Pilot Program Design
A pilot program is a small-scale implementation of a project or initiative to test its feasibility, time, cost, risk, and performance before a full-scale rollout. Designing an effective pilot program involves several key steps:
- Define Objectives: Clearly outline what the pilot aims to achieve, which could include testing new processes, technologies, or methodologies. For instance, Rapid Innovation can help clients define objectives that align with their business goals, ensuring that the pilot addresses specific challenges and opportunities.
- Select Participants: Choose a representative sample of users or stakeholders who will participate in the pilot. This group should reflect the diversity of the larger population, allowing for comprehensive feedback that can inform future decisions.
- Develop a Timeline: Establish a clear timeline for the pilot, including start and end dates, milestones, and evaluation points. Rapid Innovation can assist in creating a realistic timeline that accommodates the complexities of AI and Blockchain projects.
- Create Evaluation Metrics: Determine how success will be measured, which could include quantitative metrics (e.g., performance data) and qualitative feedback (e.g., user satisfaction). By leveraging advanced analytics, Rapid Innovation can help clients develop robust evaluation metrics that provide actionable insights.
- Plan for Feedback: Implement a structured process for collecting feedback during and after the pilot, which can include surveys, interviews, and focus groups. This feedback loop is crucial for refining the project before full-scale implementation.
- Document Everything: Keep detailed records of the pilot process, including challenges faced and solutions implemented. This documentation will be invaluable for future projects, allowing organizations to learn from their experiences.
A well-designed pilot program can provide critical insights and help mitigate risks before a full-scale implementation, ultimately leading to greater ROI for clients.
12.3. Scalability Strategy
Scalability refers to the ability of a system, process, or organization to grow and manage increased demand without compromising performance. A robust scalability strategy is essential for long-term success. Key components include:
- Assess Current Capacity: Evaluate existing resources, including technology, personnel, and processes, to understand current limitations. Rapid Innovation can conduct thorough assessments to identify bottlenecks and areas for improvement.
- Identify Growth Opportunities: Analyze market trends and customer needs to identify areas for potential growth, which could involve expanding product lines or entering new markets. Our expertise in AI and Blockchain can help clients uncover innovative solutions that drive growth.
- Invest in Technology: Leverage technology solutions that can easily scale, such as cloud computing, which allows for flexible resource allocation. Rapid Innovation can recommend and implement scalable technology solutions tailored to client needs.
- Standardize Processes: Develop standardized procedures that can be replicated across different teams or locations to ensure consistency and efficiency as the organization grows. This standardization is crucial for maintaining quality and performance.
- Build a Flexible Workforce: Create a workforce strategy that allows for easy scaling, such as hiring freelancers or part-time employees during peak times. Rapid Innovation can assist in developing a workforce strategy that aligns with business objectives.
- Monitor Performance: Continuously track key performance indicators (KPIs) to assess how well the organization is scaling and make adjustments as necessary. Our analytics capabilities enable clients to monitor performance effectively and make data-driven decisions.
A well-thought-out scalability strategy can help organizations respond to market demands effectively and sustain growth over time, maximizing ROI.
12.4. Change Management

Change management is the structured approach to transitioning individuals, teams, and organizations from a current state to a desired future state. Effective change management is crucial for minimizing resistance and ensuring successful implementation. Key elements include:
- Communicate Clearly: Develop a communication plan that outlines the reasons for the change, the benefits, and how it will impact stakeholders. Transparency is key to gaining buy-in, and Rapid Innovation can help craft compelling communication strategies.
- Engage Stakeholders: Involve key stakeholders early in the process, as their input can provide valuable insights and foster a sense of ownership over the change. Engaging stakeholders is essential for successful adoption of new technologies.
- Provide Training and Support: Offer training programs to equip employees with the skills needed to adapt to the change. Ongoing support can help ease the transition, and Rapid Innovation can design tailored training programs that address specific needs.
- Monitor and Adjust: Establish mechanisms for monitoring the change process. Collect feedback and be prepared to make adjustments based on what is or isn’t working. This iterative approach is vital for successful change management.
- Celebrate Successes: Recognize and celebrate milestones and successes throughout the change process to help maintain momentum and encourage continued engagement. Celebrating achievements fosters a positive culture during transitions.
- Evaluate Outcomes: After the change has been implemented, assess its impact against the initial objectives. This evaluation can inform future change initiatives and ensure continuous improvement.
Implementing a structured change management process can significantly enhance the likelihood of successful transitions and foster a culture of adaptability within the organization, ultimately leading to improved ROI for clients.
12.5. Continuous Improvement Framework
The Continuous Improvement Framework (CIF) is a structured approach that organizations use to enhance their processes, products, and services over time. This framework is essential for fostering a culture of ongoing improvement and ensuring that businesses remain competitive in a rapidly changing environment. Here’s a detailed look at the key components and benefits of the Continuous Improvement Framework.
- Definition and Purpose
- The Continuous Improvement Framework is designed to systematically identify, analyze, and improve existing processes. Its primary purpose is to enhance efficiency, reduce waste, and increase customer satisfaction. CIF encourages organizations to adopt a mindset of perpetual enhancement rather than settling for the status quo.
- Key Principles
- Customer Focus: Understanding and meeting customer needs is at the heart of continuous improvement.
- Data-Driven Decision Making: Utilizing data and metrics to guide improvement efforts ensures that changes are based on evidence rather than assumptions.
- Employee Involvement: Engaging employees at all levels fosters a sense of ownership and encourages innovative ideas for improvement.
- Incremental Changes: Continuous improvement emphasizes small, manageable changes rather than large-scale transformations, making it easier to implement and sustain.
- Methodologies
- Lean: Focuses on eliminating waste and optimizing processes to create more value for customers.
- Six Sigma: Aims to reduce variation and improve quality by using statistical methods.
- Plan-Do-Check-Act (PDCA): A cyclical process that involves planning changes, implementing them, checking the results, and acting on what is learned.
- Kaizen: A Japanese term meaning "change for better," which promotes continuous, incremental improvements.
- Implementation Steps
- Identify Areas for Improvement: Conduct assessments to pinpoint processes that require enhancement, such as through a process improvement framework or continuous improvement governance framework.
- Set Clear Objectives: Define specific, measurable goals for improvement initiatives, which can be guided by a continuous improvement framework example.
- Develop Action Plans: Create detailed plans outlining the steps needed to achieve the objectives, including the steps of continuous improvement framework.
- Monitor Progress: Regularly track the effectiveness of implemented changes using key performance indicators (KPIs), as seen in itil service improvement.
- Review and Adjust: Continuously evaluate the results and make necessary adjustments to the improvement strategies, utilizing the itil continuous improvement model.
- Benefits of Continuous Improvement Framework
- Enhanced Efficiency: Streamlining processes leads to reduced operational costs and improved productivity, as highlighted in the commercial continuous improvement assessment framework.
- Increased Customer Satisfaction: By focusing on customer needs, organizations can deliver higher quality products and services, aligning with continuous quality improvement framework principles.
- Employee Engagement: Involving employees in improvement initiatives boosts morale and fosters a collaborative work environment.
- Competitive Advantage: Organizations that embrace continuous improvement are better positioned to adapt to market changes and outperform competitors, as demonstrated by baldrige continuous improvement and baldrige process improvement.
- Challenges
- Resistance to Change: Employees may be hesitant to adopt new processes or practices, requiring effective change management strategies.
- Resource Allocation: Continuous improvement initiatives may require time and financial investment, which can be a challenge for some organizations.
- Sustaining Momentum: Maintaining enthusiasm for continuous improvement can be difficult, necessitating ongoing leadership support and communication.
- Tools and Techniques
- Root Cause Analysis: Identifying the underlying causes of problems to address them effectively.
- Process Mapping: Visualizing processes to identify inefficiencies and areas for improvement, which can be part of a process improvement framework example.
- Benchmarking: Comparing performance metrics with industry standards to identify gaps and opportunities for enhancement.
- Surveys and Feedback: Gathering input from customers and employees to inform improvement efforts.
- Real-World Applications
- Manufacturing: Companies often use continuous improvement to enhance production efficiency and reduce defects.
- Healthcare: Hospitals implement CIF to improve patient care and streamline operations, often through itil process improvement.
- Service Industries: Businesses in sectors like retail and hospitality utilize continuous improvement to enhance customer experiences, guided by an itil service improvement plan.
- Conclusion
- The Continuous Improvement Framework is a vital tool for organizations seeking to thrive in a competitive landscape. By fostering a culture of continuous enhancement, businesses can achieve sustainable growth and long-term success.
Incorporating the Continuous Improvement Framework into an organization’s strategy can lead to significant advancements in performance and customer satisfaction. By focusing on incremental changes and engaging employees, organizations can create a dynamic environment that embraces innovation and adaptability.
At Rapid Innovation, we leverage AI and Blockchain technologies to enhance the Continuous Improvement Framework, enabling organizations to achieve greater ROI through data-driven insights and streamlined processes. By integrating advanced analytics and decentralized solutions, we help clients not only meet but exceed their business goals efficiently and effectively. For more information on how we can assist you, visit our AI development guide for businesses.