Table Of Contents
Category
Artificial Intelligence
Healthcare & Medicine
1. Introduction to AI in Medical Imaging
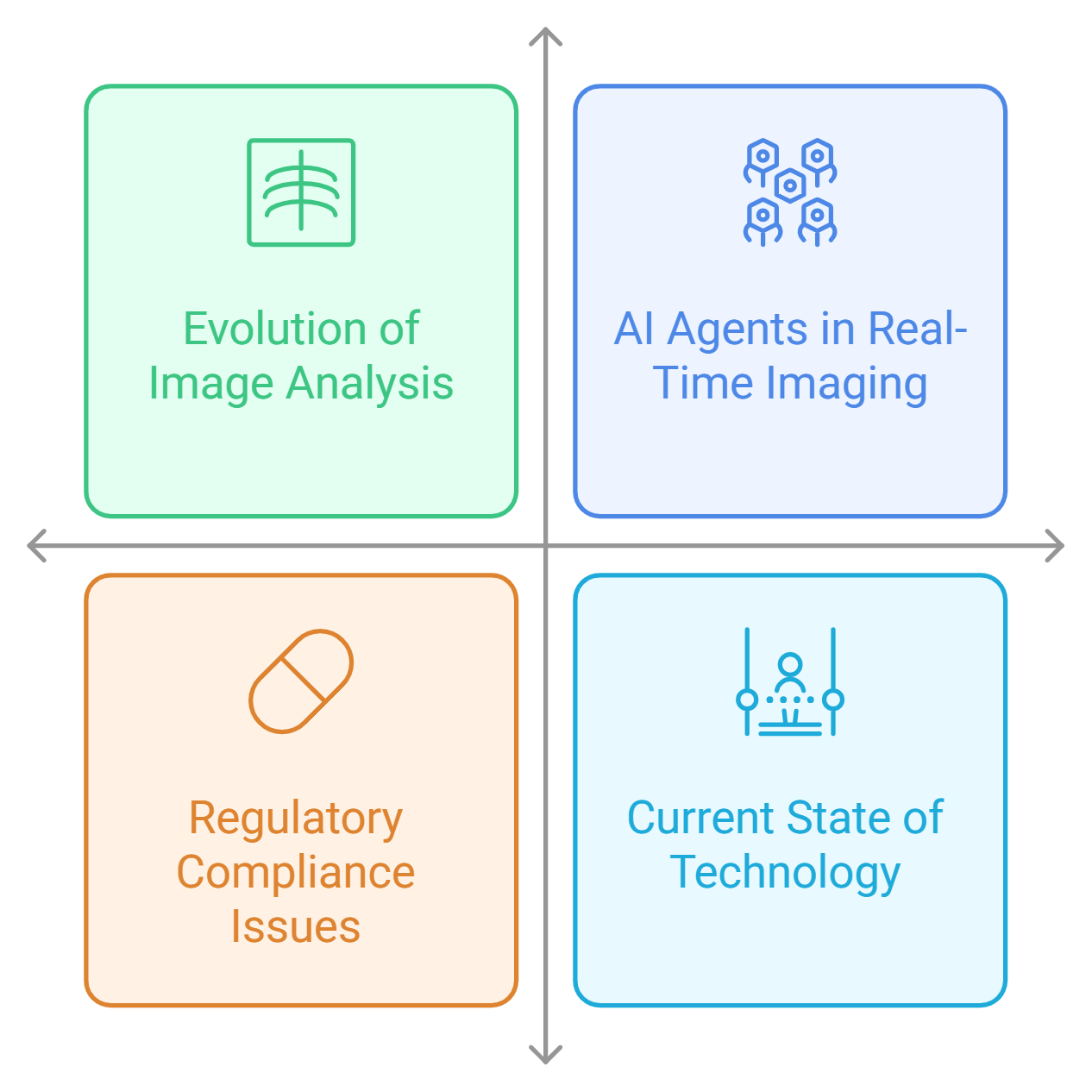
‍
Artificial Intelligence (AI) is revolutionizing the field of medical imaging, enhancing the accuracy and efficiency of diagnostics. By leveraging advanced algorithms and machine learning techniques, AI agents can analyze medical images such as X-rays, MRIs, and CT scans with remarkable precision. This integration of AI into medical imaging is not just a trend; it is a transformative approach that is reshaping how healthcare professionals interpret and utilize imaging data.
- AI algorithms can process vast amounts of imaging data quickly, reducing the time required for diagnosis.
- Machine learning models are trained on large datasets, improving their ability to identify patterns and anomalies in images.
- AI can assist radiologists by providing second opinions, thereby increasing diagnostic confidence.
At Rapid Innovation, we specialize in developing tailored AI solutions that empower healthcare providers to harness the full potential of medical imaging technologies, including ai imaging and ai medical imaging. Our expertise in AI allows us to create systems that not only enhance diagnostic accuracy but also streamline workflows, ultimately leading to greater return on investment (ROI) for our clients.
The use of AI in medical imaging is supported by a growing body of research and clinical applications. According to a report by the American College of Radiology, AI can enhance the detection of conditions such as tumors, fractures, and other abnormalities, leading to earlier interventions and better patient outcomes. As the healthcare industry continues to embrace digital transformation, the role of artificial intelligence in medical imaging is expected to expand, offering new possibilities for improving patient care and operational efficiency. Rapid Innovation is committed to guiding our clients through this evolution, ensuring they achieve their business goals effectively and efficiently. The integration of artificial intelligence in healthcare images is a key component of this transformation, as is the focus on ai medical image analysis.
1.1. Evolution of Medical Image Analysis
Medical image analysis has undergone significant evolution since its inception. Initially, the field relied heavily on traditional imaging techniques such as X-rays and CT scans. Over the years, advancements in technology have transformed how medical images are captured, processed, and analyzed. Early imaging techniques were primarily manual, requiring radiologists to interpret images visually. The introduction of digital imaging in the late 20th century allowed for better storage, retrieval, and sharing of images. The development of MRI and ultrasound technologies provided more detailed and non-invasive imaging options, including mri image analysis. In the 2000s, the integration of computer-aided detection (CAD) systems began to assist radiologists in identifying abnormalities. The rise of machine learning and deep learning in the 2010s marked a significant turning point, enabling automated analysis of medical images with high accuracy, particularly in areas like pathology image analysis and digital pathology image analysis.
These advancements have led to improved diagnostic capabilities, reduced interpretation time, and enhanced patient outcomes. At Rapid Innovation, we leverage these technological advancements to develop tailored AI solutions that enhance the efficiency and effectiveness of medical image analysis, including biomedical image analysis and histology image analysis, ultimately helping our clients achieve greater ROI.
1.2. The Role of AI Agents
Artificial Intelligence (AI) agents play a crucial role in the field of medical image analysis. They enhance the capabilities of traditional imaging techniques and provide innovative solutions for healthcare professionals. AI agents can analyze vast amounts of imaging data quickly, identifying patterns that may be missed by human eyes. They assist in diagnosing conditions such as tumors, fractures, and other abnormalities with high precision. AI algorithms can learn from large datasets, improving their accuracy over time through continuous training. These agents can reduce the workload of radiologists, allowing them to focus on more complex cases and patient care. AI-driven tools can provide real-time feedback, enabling faster decision-making in critical situations.
The integration of AI agents in medical image analysis not only improves diagnostic accuracy but also enhances workflow efficiency in healthcare settings. At Rapid Innovation, we specialize in developing AI-driven tools that empower healthcare providers to optimize their operations and improve patient outcomes, leading to a significant return on investment. This includes applications in medical imaging analytics and medical image processing and analysis.
1.3. Current State of Technology
The current state of technology in medical image analysis is characterized by rapid advancements and increasing adoption of AI and machine learning techniques. This evolution has led to several key developments in the field. AI algorithms are now capable of performing tasks such as segmentation, classification, and detection of abnormalities in medical images. Many healthcare institutions are implementing AI-powered tools to assist radiologists, leading to improved diagnostic accuracy and reduced turnaround times. The use of cloud computing and big data analytics has enabled the storage and processing of large volumes of imaging data, facilitating collaborative research and development in areas like brain image analysis and dicom image analysis. Regulatory bodies are beginning to approve AI-based diagnostic tools, paving the way for their integration into clinical practice. Ongoing research focuses on enhancing the interpretability of AI models, ensuring that healthcare professionals can understand and trust the AI's recommendations.
As technology continues to evolve, the future of medical image analysis looks promising, with the potential for even greater improvements in patient care and diagnostic capabilities. Rapid Innovation is at the forefront of this evolution, providing cutting-edge AI and blockchain solutions that help our clients navigate the complexities of medical image analysis, including soft computing based medical image analysis and pattern recognition and signal analysis in medical imaging, ensuring they achieve their business goals efficiently and effectively.
1.4. Regulatory Framework and Compliance
The regulatory framework surrounding technology, particularly in sectors like finance, healthcare, and data privacy, is crucial for ensuring that organizations operate within legal boundaries. Compliance with these regulations not only protects consumers but also enhances the credibility of businesses. This is where regtech, or regulatory technology, plays a significant role in streamlining compliance processes.
- Key regulations include: Â
- General Data Protection Regulation (GDPR): This European Union regulation governs data protection and privacy, imposing strict guidelines on data handling and user consent.
- Health Insurance Portability and Accountability Act (HIPAA): In the U.S., HIPAA sets standards for protecting sensitive patient information, requiring healthcare providers to implement security measures.
- Payment Card Industry Data Security Standard (PCI DSS): This standard is essential for organizations that handle credit card transactions, ensuring secure processing and storage of cardholder data.
- Compliance challenges: Â
- Keeping up with evolving regulations can be daunting for organizations, especially those operating in multiple jurisdictions. The integration of technology regulatory compliance solutions, such as regtech software, can help mitigate these challenges.
- Non-compliance can lead to severe penalties, including fines and legal action, which can damage a company's reputation.
- Best practices for compliance: Â
- Regular audits and assessments to identify compliance gaps, utilizing big data regulatory compliance tools to enhance accuracy.
- Employee training programs to ensure that staff understand regulatory requirements, particularly in areas like information technology regulatory compliance.
- Implementing robust data management systems to track and secure sensitive information, including compliance regtech solutions that leverage blockchain and regulatory compliance technologies.
Understanding and adhering to the regulatory framework is vital for organizations to mitigate risks and foster trust with their stakeholders. At Rapid Innovation, we assist clients in navigating these complex regulations by providing tailored compliance solutions that integrate seamlessly with their existing systems, ensuring they meet all necessary legal requirements while focusing on their core business objectives. Our expertise in regtech and compliance allows us to offer innovative solutions that enhance regulatory reporting and streamline compliance processes, including insights on AI and machine learning for regulatory compliance.
2. Fundamental Technologies
Fundamental technologies form the backbone of modern innovations, driving advancements across various industries. These technologies enable the development of new products, services, and processes that enhance efficiency and effectiveness.
- Key fundamental technologies include: Â
- Artificial Intelligence (AI): AI encompasses machine learning, natural language processing, and robotics, enabling systems to perform tasks that typically require human intelligence.
- Internet of Things (IoT): IoT connects devices and systems, allowing them to communicate and share data, leading to smarter homes, cities, and industries.
- Blockchain: This decentralized ledger technology ensures secure and transparent transactions, making it a game-changer for finance, supply chain, and more.
- Impact on industries: Â
- Healthcare: Technologies like AI and IoT are revolutionizing patient care through telemedicine and personalized treatment plans.
- Finance: Blockchain is enhancing security and efficiency in transactions, while AI is improving fraud detection and customer service.
- Manufacturing: Automation and IoT are streamlining production processes, reducing costs, and increasing productivity.
- Future trends: Â
- Continued integration of AI and machine learning into everyday applications.
- Expansion of IoT devices, leading to more interconnected systems.
- Increased focus on cybersecurity as reliance on digital technologies grows.
Understanding these fundamental technologies is essential for businesses looking to innovate and stay competitive in a rapidly changing landscape. Rapid Innovation leverages these technologies to help clients achieve greater ROI by developing customized solutions that drive efficiency and enhance operational capabilities.
2.1. Deep Learning Architectures
Deep learning architectures are a subset of machine learning that utilize neural networks with multiple layers to analyze data and make predictions. These architectures have gained prominence due to their ability to process vast amounts of data and extract meaningful insights.
- Common deep learning architectures include: Â
- Convolutional Neural Networks (CNNs): Primarily used for image processing, CNNs excel at recognizing patterns and features in visual data.
- Recurrent Neural Networks (RNNs): RNNs are designed for sequential data, making them ideal for tasks like language modeling and time series prediction.
- Generative Adversarial Networks (GANs): GANs consist of two neural networks that compete against each other, enabling the generation of realistic synthetic data.
- Applications of deep learning: Â
- Image and speech recognition: Deep learning models are widely used in applications like facial recognition and voice assistants.
- Natural language processing: These architectures power chatbots, translation services, and sentiment analysis tools.
- Autonomous systems: Deep learning is crucial for the development of self-driving cars and drones, enabling them to navigate and make decisions in real-time.
- Challenges in deep learning: Â
- Data requirements: Deep learning models often require large datasets for training, which can be a barrier for some organizations.
- Interpretability: Understanding how deep learning models arrive at their decisions can be complex, raising concerns about transparency and accountability.
- Computational resources: Training deep learning models can be resource-intensive, necessitating powerful hardware and infrastructure.
Deep learning architectures are transforming industries by enabling advanced analytics and automation, making them a critical area of focus for businesses aiming to leverage AI technologies. Rapid Innovation specializes in implementing deep learning solutions that not only enhance decision-making processes but also drive significant business value.
2.1.1. Convolutional Neural Networks (CNNs)
Convolutional Neural Networks (CNNs) are a class of deep learning models primarily used for image processing and computer vision tasks. They are designed to automatically and adaptively learn spatial hierarchies of features from images.
- Key components of CNNs include: Â
- Convolutional Layers: These layers apply convolution operations to the input, allowing the model to learn local patterns such as edges and textures. Convolutional neural networks layers are essential for this process.
- Activation Functions: Commonly used functions like ReLU (Rectified Linear Unit) introduce non-linearity, enabling the network to learn complex patterns.
- Pooling Layers: These layers reduce the spatial dimensions of the input, which helps in decreasing the computational load and controlling overfitting.
- Fully Connected Layers: At the end of the network, these layers connect every neuron from the previous layer to every neuron in the next layer, allowing for classification tasks.
- CNNs have several advantages: Â
- Parameter Sharing: This reduces the number of parameters, making the model more efficient.
- Translation Invariance: CNNs can recognize objects in images regardless of their position, enhancing their robustness.
- Hierarchical Feature Learning: They learn features at multiple levels, from simple edges to complex shapes.
CNNs have been widely adopted in various applications, including image classification, object detection, and segmentation tasks. Their effectiveness is evident in popular models like AlexNet, VGGNet, and ResNet. At Rapid Innovation, we leverage convolutional neural networks and deep learning models to develop tailored solutions that enhance image analysis capabilities for our clients, ultimately driving greater ROI through improved operational efficiency.
2.1.2. U-Net Architecture
U-Net is a specialized architecture designed for biomedical image segmentation. It is particularly effective in tasks where precise localization is crucial, such as identifying cells or organs in medical images.
- The U-Net architecture consists of two main parts: Â
- Contracting Path: This part captures context through a series of convolutional and pooling layers. It progressively reduces the spatial dimensions while increasing the number of feature channels.
- Expansive Path: This part enables precise localization by upsampling the feature maps and concatenating them with corresponding feature maps from the contracting path. This skip connection helps retain spatial information lost during downsampling.
- Key features of U-Net include: Â
- Skip Connections: These connections allow the model to combine high-resolution features from the contracting path with upsampled features, improving segmentation accuracy.
- Symmetrical Architecture: The U-shaped design facilitates efficient learning and helps in maintaining the spatial dimensions throughout the network.
- Data Augmentation: U-Net is often trained on limited datasets, and data augmentation techniques are employed to enhance the model's robustness.
U-Net has become a standard in medical image analysis, achieving state-of-the-art results in various segmentation challenges. Its flexibility and effectiveness make it a popular choice for researchers and practitioners in the field. Rapid Innovation utilizes U-Net to provide clients in the healthcare sector with advanced image segmentation solutions, leading to better diagnostic accuracy and improved patient outcomes.
2.1.3. Transfer Learning Approaches
Transfer learning is a powerful technique in deep learning that allows models to leverage knowledge gained from one task to improve performance on another related task. This approach is particularly beneficial when labeled data is scarce.
- Key aspects of transfer learning include: Â
- Pre-trained Models: Models like VGG, ResNet, and Inception are trained on large datasets (e.g., ImageNet) and can be fine-tuned for specific tasks. This reduces training time and improves accuracy.
- Feature Extraction: In this approach, the pre-trained model is used as a fixed feature extractor. The output from one of the intermediate layers is fed into a new classifier tailored for the specific task.
- Fine-tuning: This involves unfreezing some of the layers of the pre-trained model and training them on the new dataset. Fine-tuning allows the model to adapt to the new task while retaining the learned features.
- Benefits of transfer learning include: Â
- Reduced Training Time: Since the model starts with pre-learned features, it requires less time to converge.
- Improved Performance: Transfer learning often leads to better performance, especially in tasks with limited data.
- Lower Computational Resources: It reduces the need for extensive computational resources, making it accessible for smaller organizations or individual researchers.
Transfer learning has been successfully applied in various domains, including natural language processing, image classification, and even reinforcement learning, making it a versatile tool in the machine learning toolkit. At Rapid Innovation, we harness transfer learning to accelerate project timelines and enhance model performance for our clients, ensuring they achieve their business objectives efficiently and effectively. Additionally, we explore the integration of recurrent neural networks (RNNs) and deep belief networks in our projects to further enhance our machine learning capabilities.
2.2. Computer Vision Techniques
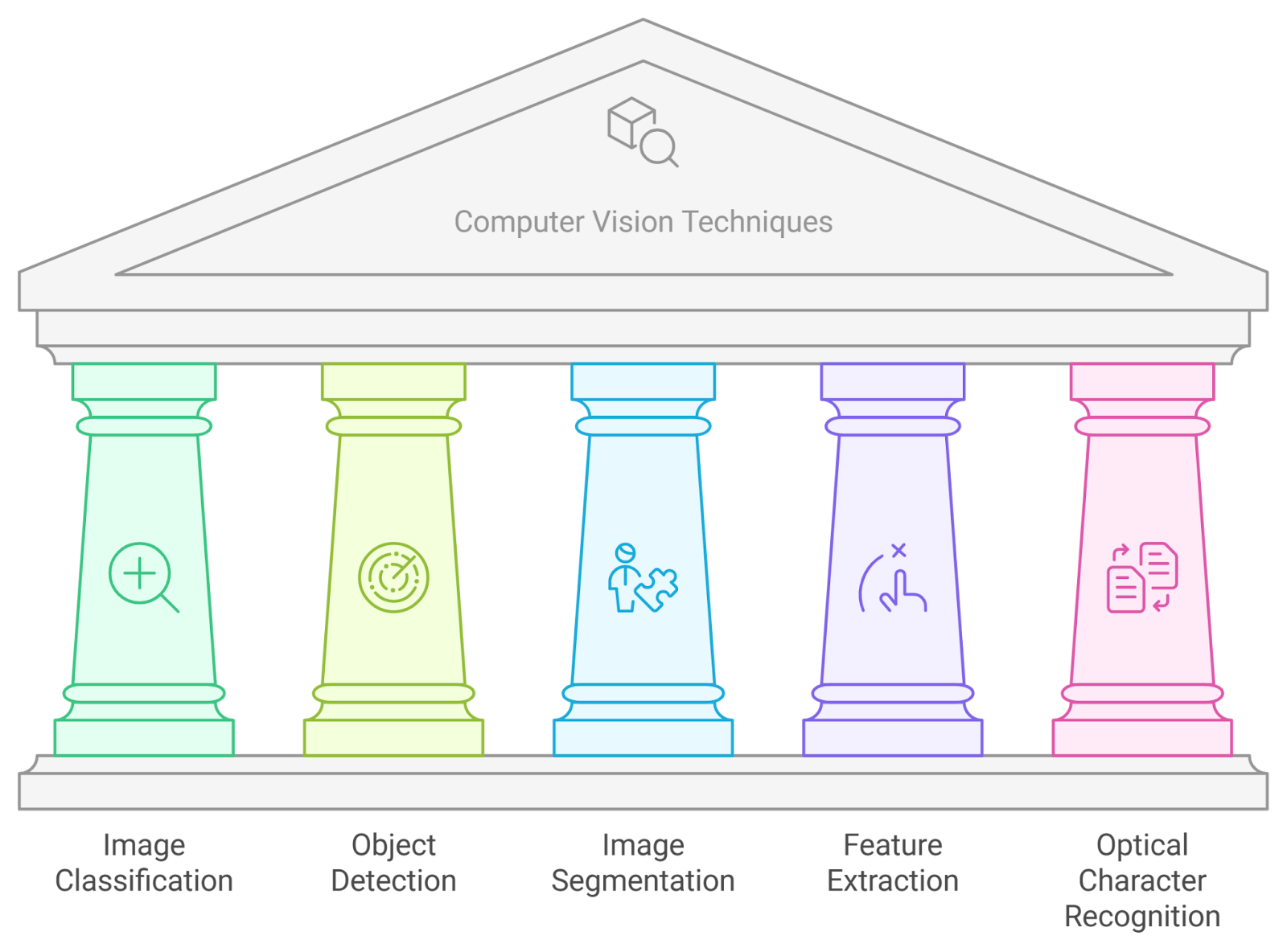
‍
Computer vision is a field of artificial intelligence that enables machines to interpret and make decisions based on visual data. Various techniques are employed to enhance the capabilities of computer vision systems, making them essential in numerous applications.
- Image Classification: This technique involves categorizing images into predefined classes. Convolutional Neural Networks (CNNs) are commonly used for this purpose, allowing for high accuracy in identifying objects within images. Rapid Innovation leverages CNNs to help clients in sectors like retail and healthcare, improving product recognition and patient diagnostics, respectively.
- Object Detection: This technique not only identifies objects within an image but also locates them. Algorithms like YOLO (You Only Look Once) and Faster R-CNN are popular for real-time object detection, making them suitable for applications such as autonomous vehicles and surveillance systems. By implementing these algorithms, Rapid Innovation assists clients in enhancing security measures and automating logistics operations. This includes the use of object detection techniques in computer vision and traditional computer vision techniques.
- Image Segmentation: This process divides an image into segments to simplify its representation. Techniques like semantic segmentation and instance segmentation help in understanding the context of images, which is crucial for tasks like medical imaging and scene understanding. Rapid Innovation employs these techniques, including computer vision image segmentation, to provide clients with detailed insights, leading to better decision-making in fields such as urban planning and healthcare. This also encompasses image segmentation in computer vision and computer vision segmentation algorithms.
- Feature Extraction: This involves identifying and isolating various features within an image, such as edges, textures, and shapes. Techniques like Scale-Invariant Feature Transform (SIFT) and Histogram of Oriented Gradients (HOG) are widely used for feature extraction. Rapid Innovation utilizes these methods to enhance image analysis capabilities for clients, resulting in improved product development and quality control processes. This is part of the broader scope of classical computer vision techniques and advanced methods and deep learning in computer vision.
- Optical Character Recognition (OCR): OCR technology converts different types of documents, such as scanned paper documents or PDFs, into editable and searchable data. This is particularly useful in digitizing printed texts and automating data entry processes. Rapid Innovation's OCR solutions help clients streamline their operations, reduce manual errors, and enhance data accessibility. This technology can also be applied in machine vision techniques and image processing techniques in computer vision.
2.3. Natural Language Processing for Report Generation
Natural Language Processing (NLP) is a branch of artificial intelligence that focuses on the interaction between computers and humans through natural language. In the context of report generation, NLP techniques play a crucial role in automating and enhancing the process.
- Text Summarization: This technique condenses lengthy documents into shorter summaries while retaining essential information. There are two main approaches: extractive summarization, which selects key sentences from the text, and abstractive summarization, which generates new sentences to convey the main ideas. Rapid Innovation employs these techniques to help clients quickly derive insights from large volumes of text, improving efficiency in reporting.
- Sentiment Analysis: This involves determining the sentiment expressed in a piece of text, whether positive, negative, or neutral. By analyzing sentiments, organizations can gauge public opinion and customer feedback, which can be valuable for report generation. Rapid Innovation's sentiment analysis tools enable clients to better understand market trends and customer preferences, leading to more informed business strategies.
- Named Entity Recognition (NER): NER identifies and classifies key entities in text, such as names, organizations, and locations. This is particularly useful in generating reports that require specific data points, allowing for more structured and informative outputs. Rapid Innovation integrates NER into its solutions to enhance data accuracy and relevance for clients' reporting needs.
- Language Generation: Advanced NLP models, such as GPT-3, can generate human-like text based on prompts. This capability can be leveraged to create comprehensive reports, making the process faster and more efficient. Rapid Innovation utilizes these models to automate report writing, saving clients time and resources.
- Data Extraction: NLP techniques can extract relevant data from unstructured text, such as emails or articles. This data can then be organized and presented in reports, enhancing the quality and relevance of the information provided. Rapid Innovation's data extraction solutions empower clients to harness valuable insights from diverse data sources.
2.4. Data Preprocessing Methods
Data preprocessing is a critical step in data analysis and machine learning, as it prepares raw data for further processing. Effective preprocessing methods can significantly improve the performance of models and the quality of insights derived from data.
- Data Cleaning: This involves identifying and correcting errors or inconsistencies in the data. Common tasks include removing duplicates, handling missing values, and correcting data types. Clean data is essential for accurate analysis and modeling. Rapid Innovation emphasizes data cleaning to ensure that clients' analyses are based on reliable datasets.
- Data Transformation: This process modifies data into a suitable format for analysis. Techniques include normalization, which scales data to a specific range, and standardization, which centers data around the mean. These transformations help in improving model performance. Rapid Innovation applies these techniques to enhance the effectiveness of clients' machine learning models.
- Feature Engineering: This involves creating new features from existing data to enhance model accuracy. Techniques include polynomial features, interaction terms, and binning continuous variables. Well-engineered features can lead to better predictive performance. Rapid Innovation collaborates with clients to develop tailored feature engineering strategies that drive better outcomes.
- Data Reduction: This technique reduces the volume of data while preserving its integrity. Methods like Principal Component Analysis (PCA) and feature selection help in eliminating irrelevant or redundant features, making the dataset more manageable. Rapid Innovation employs data reduction techniques to optimize clients' data processing workflows, leading to faster insights.
- Data Encoding: Categorical data often needs to be converted into numerical format for machine learning algorithms. Techniques such as one-hot encoding and label encoding are commonly used to facilitate this conversion, ensuring that models can effectively interpret the data. Rapid Innovation ensures that clients' datasets are properly encoded, enhancing the performance of their machine learning applications.
3. Key Use Cases
3.1. Radiological Analysis
Radiological analysis plays a crucial role in modern medicine, enabling healthcare professionals to diagnose and treat various conditions effectively. This field leverages advanced imaging technologies to visualize the internal structures of the body, allowing for accurate assessments of health. Key aspects of radiological analysis include:
- Enhanced diagnostic accuracy: Radiological imaging provides detailed views of organs and tissues, helping to identify abnormalities.
- Non-invasive procedures: Many imaging techniques, such as X-rays, CT scans, and MRIs, allow for internal examination without the need for surgical intervention.
- Early detection of diseases: Radiological analysis can reveal conditions like tumors or fractures at an early stage, improving treatment outcomes.
3.1.1. X-Ray Analysis
X-ray analysis is one of the most common forms of radiological imaging. It uses electromagnetic radiation to create images of the body's internal structures. The process involves several key components:
- How X-rays work: X-rays pass through the body and are absorbed by different tissues at varying rates. Dense tissues, like bones, appear white on the X-ray film, while softer tissues appear darker.
- Common applications: X-ray analysis is widely used for diagnosing fractures, infections, and tumors. It is also essential in dental imaging and chest examinations.
- Safety considerations: While X-rays involve exposure to radiation, the levels are generally low and considered safe for diagnostic purposes. However, precautions are taken to minimize exposure, especially in vulnerable populations like children and pregnant women.
Benefits of X-ray analysis include:
- Quick results: X-ray imaging is fast, often providing results within minutes, which is critical in emergency situations.
- Cost-effectiveness: X-ray procedures are typically less expensive than other imaging modalities, making them accessible for many patients.
- Versatility: X-rays can be used in various medical fields, including orthopedics, oncology, and cardiology.
At Rapid Innovation, we harness AI technologies to enhance radiological analysis by automating image interpretation and improving diagnostic accuracy. Our AI-driven solutions can analyze X-ray images rapidly, flagging potential issues for healthcare professionals to review, thus streamlining workflows and reducing the time to diagnosis. This not only enhances patient care but also contributes to greater ROI for healthcare providers by optimizing resource allocation and minimizing unnecessary procedures.
In addition to X-ray analysis, quantitative analysis in nuclear medicine imaging is another vital aspect of radiological analysis. This approach allows for precise measurements of radiopharmaceutical distribution in the body, aiding in the diagnosis and treatment of various conditions.
Furthermore, radiographic critique pdfs serve as valuable resources for training and education, providing insights into the evaluation of radiographic images and enhancing the skills of healthcare professionals.
In conclusion, radiological analysis, particularly X-ray analysis and quantitative analysis in nuclear medicine imaging, is an indispensable tool in modern healthcare, facilitating accurate diagnoses and timely interventions. By integrating AI solutions, Rapid Innovation empowers healthcare organizations to achieve their business goals efficiently and effectively.
3.1.2. CT Scan Interpretation
CT scans, or computed tomography scans, are essential diagnostic tools in modern medicine. They provide detailed images of internal structures, allowing healthcare professionals to identify various conditions.
- CT scans use X-ray technology to create cross-sectional images of the body.
- Interpretation involves analyzing these images for abnormalities such as tumors, fractures, or infections.
- Radiologists look for specific signs, including:
- Density differences in tissues
- Abnormal shapes or sizes of organs
- Presence of fluid or air in unexpected areas
- The interpretation process often requires correlating CT findings with clinical history and other diagnostic tests.
- Advanced software can assist in enhancing images and providing 3D reconstructions for better visualization.
- Radiologists must be aware of the patient's history, including previous imaging studies, to make accurate assessments.
At Rapid Innovation, we leverage AI algorithms to enhance the interpretation of CT scans. By integrating machine learning models, we can assist radiologists in identifying subtle abnormalities that may be overlooked, ultimately improving diagnostic accuracy and patient outcomes. This not only streamlines the workflow but also contributes to a higher return on investment (ROI) for healthcare providers by reducing the time spent on image analysis.
CT scan interpretation encompasses various aspects, including chest CT interpretation, brain CT interpretation, and abdominal CT interpretation. Radiologists often engage in reading chest CT and performing CT thorax interpretation to assess lung conditions. Additionally, the basics of CT scan interpretation are crucial for medical students learning to read CT scan reports. Advanced techniques such as CT perfusion interpretation can provide insights into blood flow dynamics, while CT scan interpretation of findings is essential for accurate diagnosis.
3.1.3. MRI Analysis
Magnetic Resonance Imaging (MRI) is a non-invasive imaging technique that uses strong magnets and radio waves to generate detailed images of organs and tissues. MRI analysis is crucial for diagnosing various medical conditions.
- MRI is particularly effective for soft tissue imaging, making it ideal for brain, spinal cord, and joint assessments.
- The analysis involves interpreting different MRI sequences, which highlight various tissue characteristics.
- Key aspects of MRI analysis include:
- Identifying lesions or abnormalities in soft tissues
- Evaluating the integrity of ligaments and cartilage
- Assessing brain structures for conditions like tumors or strokes
- Radiologists must consider the patient's clinical symptoms and history when interpreting MRI results.
- Advanced techniques, such as functional MRI (fMRI), can assess brain activity by measuring blood flow changes.
- MRI analysis can also involve comparing images over time to monitor disease progression or treatment response.
Rapid Innovation employs AI-driven tools to enhance MRI analysis, enabling more precise identification of abnormalities and improved monitoring of treatment responses. By automating routine tasks, we help healthcare professionals focus on critical decision-making, thereby increasing efficiency and ROI for medical facilities.
3.1.4. PET Scan Assessment
Positron Emission Tomography (PET) scans are advanced imaging techniques that provide insights into metabolic processes in the body. PET scan assessment is vital for diagnosing and monitoring various diseases, particularly cancer.
- PET scans use radioactive tracers to visualize metabolic activity in tissues.
- The assessment focuses on areas of increased or decreased tracer uptake, which can indicate disease presence.
- Key points in PET scan assessment include:
- Identifying cancerous lesions based on their metabolic activity
- Evaluating the effectiveness of cancer treatments by comparing pre- and post-treatment scans
- Assessing brain function and detecting neurological disorders
- PET scans are often combined with CT or MRI scans to provide comprehensive anatomical and functional information.
- Radiologists must interpret PET images in conjunction with clinical data and other imaging modalities for accurate diagnosis.
- The assessment process may involve quantifying tracer uptake using standardized uptake values (SUVs) to aid in diagnosis and treatment planning.
At Rapid Innovation, we integrate blockchain technology to ensure the secure sharing of PET scan data among healthcare providers. This not only enhances collaboration but also ensures data integrity and patient privacy, leading to improved patient care and a significant ROI for healthcare organizations. By streamlining data management processes, we help clients achieve their business goals efficiently and effectively.
3.2. Cancer Detection and Classification
Cancer detection and classification are critical components in the fight against cancer. Early detection significantly increases the chances of successful treatment and survival. Various methods are employed to identify cancerous cells and classify the type of cancer, which helps in determining the most effective treatment options. Advanced imaging techniques and laboratory tests are essential for accurate diagnosis. The classification of cancer helps in understanding the stage and aggressiveness of the disease. Furthermore, early detection can lead to better outcomes and lower treatment costs.
At Rapid Innovation, we leverage AI algorithms to enhance the accuracy and efficiency of cancer detection processes. By integrating machine learning models with imaging technologies, we can assist healthcare providers in identifying abnormalities with greater precision, ultimately leading to improved patient outcomes and higher ROI for healthcare institutions.
3.2.1. Mammography
Mammography is a specialized medical imaging technique used primarily for the early detection of breast cancer. It involves taking X-ray images of the breast to identify any abnormalities.
- Screening Tool: Mammograms are used as a screening tool for women, especially those aged 40 and above, to detect breast cancer before symptoms appear.
- Types of Mammograms: There are two main types of mammograms: Â
- Screening Mammograms: Performed on women with no symptoms to check for signs of breast cancer.
- Diagnostic Mammograms: Conducted when there are symptoms or abnormalities detected in a screening mammogram.
- Effectiveness: Studies show that regular mammography can reduce breast cancer mortality by about 20-30% in women aged 40-74.
- Digital Mammography: This modern technique provides clearer images and can be more effective in detecting cancer in women with dense breast tissue.
- Follow-Up Procedures: If a mammogram shows suspicious results, further tests such as ultrasound or biopsy may be recommended for confirmation.
3.2.2. Lung Cancer Screening
Lung cancer screening is a process aimed at detecting lung cancer at an early stage when it is more treatable. The primary method for lung cancer screening is low-dose computed tomography (LDCT).
- Target Population: Screening is generally recommended for high-risk individuals, particularly those aged 50-80 with a significant smoking history (e.g., 20 pack-years).
- Benefits of LDCT: Â
- LDCT has been shown to reduce lung cancer mortality by 20% in high-risk populations compared to chest X-rays.
- It provides detailed images of the lungs, allowing for the detection of small nodules that may indicate cancer.
- Screening Frequency: The recommended frequency for lung cancer screening is annually, as long as the individual remains at high risk.
- Follow-Up Care: If a lung nodule is detected, further evaluation through imaging or biopsy may be necessary to determine if it is cancerous.
- Limitations: While screening can save lives, it also has limitations, including false positives and the potential for overdiagnosis, which can lead to unnecessary anxiety and procedures.
In conclusion, both mammography and lung cancer screening play vital roles in the early detection and classification of cancer, ultimately improving patient outcomes and survival rates. Regular screenings and awareness of risk factors are essential for effective cancer management. Rapid Innovation is committed to enhancing these processes through AI-driven solutions, ensuring that healthcare providers can achieve greater efficiency and effectiveness in their cancer detection efforts.
3.2.3. Brain Tumor Detection
Brain tumor detection is a critical area in medical imaging and diagnostics. Early detection significantly improves treatment outcomes and patient survival rates. Various imaging techniques are employed to identify brain tumors, including:
- Magnetic Resonance Imaging (MRI): MRI is the gold standard for brain tumor detection. It provides high-resolution images of brain structures and can differentiate between tumor types based on their characteristics.
- Computed Tomography (CT) Scan: CT scans are often used in emergency settings. They can quickly identify large tumors and assess any associated bleeding or swelling in the brain.
- Positron Emission Tomography (PET): PET scans can help determine the metabolic activity of a tumor, providing insights into its aggressiveness and potential response to treatment.
Advancements in artificial intelligence (AI) and machine learning are enhancing brain tumor detection. AI algorithms can analyze imaging data to identify tumors with high accuracy, often outperforming human radiologists. Studies have shown that AI can reduce diagnostic errors and improve the speed of detection. The use of contrast agents in MRI and CT scans can enhance the visibility of tumors, making it easier to identify their size and location. Regular screening and monitoring are essential for patients with a family history of brain tumors or those exhibiting symptoms such as persistent headaches, seizures, or cognitive changes.
At Rapid Innovation, we leverage our expertise in AI to develop tailored solutions that enhance the accuracy and efficiency of brain tumor detection. By integrating advanced machine learning algorithms into existing imaging systems, we help healthcare providers achieve greater ROI through improved diagnostic capabilities and reduced operational costs. For more information on our services, visit our AI Healthcare Management page. Additionally, you can learn more about the role of AI in patient care by exploring our article on AI agents for patient care: use cases, benefits, and challenges.
3.3. Cardiovascular Imaging
Cardiovascular imaging plays a vital role in diagnosing and managing heart diseases. It encompasses various techniques that visualize the heart's structure and function, helping healthcare providers make informed decisions about treatment. Key methods include:
- Echocardiography: This ultrasound-based technique provides real-time images of the heart, allowing for the assessment of heart chambers, valves, and blood flow.
- Cardiac MRI: This non-invasive imaging technique offers detailed images of the heart's anatomy and function, particularly useful for evaluating complex conditions.
- Coronary Angiography: This procedure involves injecting a contrast dye into the coronary arteries to visualize blockages or abnormalities.
The importance of cardiovascular imaging cannot be overstated, as heart disease remains a leading cause of death worldwide. Early detection through imaging can lead to timely interventions, reducing morbidity and mortality rates. Advances in imaging technology, such as 3D echocardiography and cardiac CT, provide more detailed views of the heart, improving diagnostic accuracy. Integration of imaging with other diagnostic tools, like blood tests and patient history, enhances the overall assessment of cardiovascular health.
3.3.1. Echocardiogram Analysis
Echocardiogram analysis is a crucial component of cardiovascular imaging, providing insights into heart function and structure. This non-invasive test uses sound waves to create images of the heart, allowing for the evaluation of various conditions. Key aspects of echocardiogram analysis include:
- Assessment of Heart Chambers: Echocardiograms can measure the size and function of the heart's chambers, helping to identify conditions like cardiomyopathy or heart failure.
- Evaluation of Valvular Function: The test can assess the function of heart valves, detecting issues such as stenosis or regurgitation that may require surgical intervention.
- Measurement of Ejection Fraction: This critical parameter indicates how well the heart pumps blood. A reduced ejection fraction can signal heart failure or other cardiac conditions.
Echocardiogram analysis is often complemented by Doppler ultrasound, which evaluates blood flow through the heart and vessels. This combination provides a comprehensive view of cardiovascular health. Additionally, stress echocardiography can be performed to assess how the heart functions under physical stress, aiding in the diagnosis of coronary artery disease. The use of advanced techniques, such as 3D echocardiography, enhances visualization and improves diagnostic accuracy.
Regular echocardiogram analysis is essential for patients with known heart conditions or risk factors, enabling timely interventions and monitoring of disease progression. Rapid Innovation's expertise in AI can further enhance echocardiogram analysis by automating image interpretation and providing predictive analytics, ultimately leading to improved patient outcomes and increased operational efficiency for healthcare providers.
3.3.2. Coronary Artery Disease Detection
Coronary artery disease (CAD) is a leading cause of morbidity and mortality worldwide. Early detection is crucial for effective management and treatment. Various imaging techniques and diagnostic tools are employed to identify CAD, including:
- Coronary Angiography: This is the gold standard for diagnosing CAD. It involves injecting a contrast dye into the coronary arteries and taking X-ray images to visualize blockages or narrowing.
- Computed Tomography (CT) Angiography: A non-invasive alternative to traditional angiography, CT angiography provides detailed images of the coronary arteries. It is particularly useful for assessing coronary artery anomalies and calcifications.
- Magnetic Resonance Imaging (MRI): Cardiac MRI can evaluate heart function and detect areas of ischemia or scarring in the heart muscle. It is beneficial for patients with contraindications to other imaging modalities.
- Stress Testing: This involves monitoring the heart's performance under physical stress, often using treadmill tests or pharmacological agents. Abnormal results may indicate the presence of CAD.
- Echocardiography: This ultrasound-based technique assesses heart structure and function. It can help identify areas of the heart that are not receiving adequate blood flow due to blocked arteries.
Advancements in artificial intelligence (AI) and machine learning are enhancing CAD detection. These technologies analyze imaging data to identify patterns indicative of CAD, improving diagnostic accuracy and speed. At Rapid Innovation, we leverage AI algorithms to develop solutions that assist healthcare providers in early CAD detection, ultimately leading to improved patient outcomes and greater return on investment (ROI) for healthcare systems.
3.4. Pathology Image Analysis
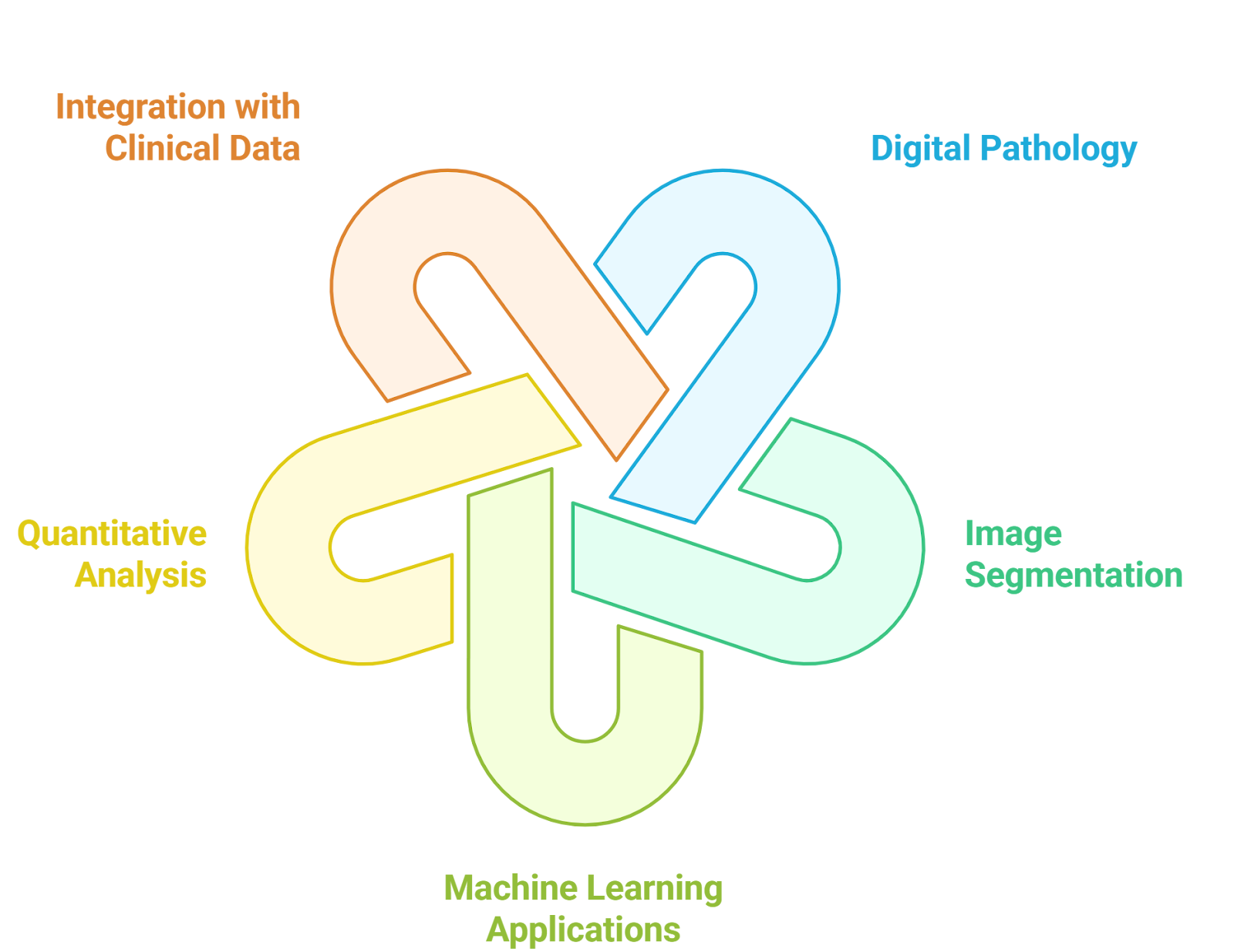
‍
Pathology image analysis plays a critical role in diagnosing diseases by examining tissue samples under a microscope. This process is essential for identifying cancer, infections, and other conditions. Key aspects include:
- Digital Pathology: This involves converting glass slides into digital images, allowing pathologists to analyze samples on computers. Digital pathology enhances collaboration and enables remote consultations.
- Image Segmentation: This technique isolates specific regions of interest within pathology images, such as tumors or abnormal cells. Accurate segmentation is vital for quantifying disease characteristics and guiding treatment decisions.
- Machine Learning Applications: AI algorithms are increasingly used to assist pathologists in diagnosing diseases. These algorithms can identify patterns in large datasets, improving diagnostic accuracy and reducing human error.
- Quantitative Analysis: Advanced imaging techniques allow for the quantification of various features in pathology images, such as cell density and tumor size. This data can provide insights into disease progression and treatment response.
- Integration with Clinical Data: Combining pathology image analysis with clinical data enhances the understanding of disease mechanisms and patient outcomes. This holistic approach can lead to more personalized treatment strategies.
The future of pathology image analysis lies in the integration of AI and big data, which promises to revolutionize diagnostics and improve patient care. Rapid Innovation is at the forefront of this transformation, providing AI-driven solutions that enhance diagnostic capabilities and streamline workflows, ultimately leading to increased efficiency and ROI for healthcare providers.
3.5. Dental Imaging
Dental imaging is a crucial component of modern dentistry, providing essential information for diagnosis, treatment planning, and monitoring oral health. Various imaging modalities are utilized, including:
- X-rays: Traditional dental X-rays, such as bitewings and periapical images, are fundamental for detecting cavities, bone loss, and other dental issues. They are quick, cost-effective, and widely used.
- Cone Beam Computed Tomography (CBCT): This advanced imaging technique provides three-dimensional images of the teeth, jaws, and surrounding structures. CBCT is particularly useful for implant planning, orthodontics, and evaluating complex dental conditions.
- Intraoral Cameras: These small cameras allow dentists to capture high-resolution images of the inside of the mouth. They enhance patient education by providing visual evidence of dental issues.
- Panoramic Radiography: This technique captures a single image of the entire mouth, including the teeth, jaws, and surrounding structures. It is useful for assessing wisdom teeth, jaw disorders, and overall dental health.
- Digital Imaging: Digital radiography offers several advantages over traditional film, including reduced radiation exposure and immediate image availability. It also allows for easier storage and sharing of images.
- 3D Imaging: Advanced imaging technologies enable the creation of three-dimensional models of dental structures. These models assist in treatment planning and can improve the accuracy of procedures such as orthodontics and restorative dentistry.
The integration of dental imaging with digital technologies is transforming the field, enhancing diagnostic capabilities and improving patient outcomes. Rapid Innovation's expertise in AI and blockchain can further enhance these processes by ensuring secure data management and facilitating seamless integration of imaging technologies, ultimately driving better patient care and higher ROI for dental practices.
3.6. Ophthalmological Imaging
Ophthalmological imaging refers to a range of techniques used to visualize the structures of the eye and its surrounding tissues. These imaging modalities are crucial for diagnosing and managing various eye conditions.
- Optical Coherence Tomography (OCT): Â
- Provides high-resolution cross-sectional images of the retina.
- Useful for detecting retinal diseases such as macular degeneration and diabetic retinopathy.
- Fundus Photography: Â
- Captures detailed images of the interior surface of the eye, including the retina, optic disc, and macula.
- Helps in monitoring changes over time in conditions like glaucoma and diabetic eye disease.
- Fluorescein Angiography: Â
- Involves injecting a fluorescent dye into the bloodstream to visualize blood flow in the retina.
- Essential for diagnosing retinal vascular diseases and assessing the effectiveness of treatments.
- Ultrasound Biomicroscopy: Â
- Uses high-frequency sound waves to create images of the anterior segment of the eye.
- Valuable for evaluating tumors, cysts, and other abnormalities in the eye.
- Binocular Indirect Ophthalmoscopy Inverted Image: Â
- Allows for a wide-field view of the retina, providing detailed visualization of the retinal structures.
- Useful in detecting and monitoring various retinal conditions.
- Indirect Ophthalmoscopy Inverted Image: Â
- Offers a comprehensive view of the fundus, aiding in the diagnosis of ocular diseases.
- Enhances the ability to assess the optic nerve and peripheral retina.
These imaging techniques have revolutionized ophthalmology by providing detailed insights into eye health, enabling early detection and intervention for various ocular diseases. At Rapid Innovation, we leverage AI and blockchain technologies to enhance these imaging processes, ensuring that healthcare providers can achieve greater efficiency and effectiveness in their practices, ultimately leading to improved ROI and patient care. For more information on how technology is transforming healthcare.
4. Clinical Benefits
The clinical benefits of advanced ophthalmological imaging are significant, impacting both patient outcomes and the efficiency of eye care practices.
- Enhanced Patient Care: Â
- Early detection of eye diseases leads to timely treatment, reducing the risk of vision loss.
- Continuous monitoring allows for adjustments in treatment plans based on real-time data.
- Comprehensive Data Collection: Â
- Imaging provides objective data that can be used to track disease progression.
- Facilitates better communication between healthcare providers and patients regarding treatment options.
- Improved Surgical Planning: Â
- Detailed imaging assists surgeons in planning complex eye surgeries.
- Reduces the likelihood of complications during procedures.
4.1. Improved Diagnostic Accuracy
One of the most significant advantages of ophthalmological imaging is its ability to enhance diagnostic accuracy.
- Precision in Diagnosis: Â
- Advanced imaging techniques allow for the identification of subtle changes in the eye that may not be visible through traditional examination methods. For instance, OCT can detect early signs of retinal diseases, leading to more accurate diagnoses.
- Reduction in Misdiagnosis: Â
- With detailed imaging, the likelihood of misdiagnosis decreases significantly. Studies have shown that the use of OCT can improve diagnostic accuracy for conditions like glaucoma by up to 90%.
- Tailored Treatment Plans: Â
- Accurate imaging results enable healthcare providers to create personalized treatment plans based on the specific needs of each patient. This individualized approach can lead to better outcomes and higher patient satisfaction.
- Integration with Artificial Intelligence: Â
- The incorporation of AI in ophthalmological imaging is further enhancing diagnostic accuracy. AI algorithms can analyze imaging data to identify patterns and anomalies that may be missed by the human eye, leading to earlier and more accurate diagnoses.
In summary, the advancements in ophthalmological imaging have transformed the field of eye care, providing clinicians with powerful tools to improve diagnostic accuracy and patient outcomes.
4.2. Early Disease Detection
Early disease detection is crucial in improving patient outcomes and reducing healthcare costs. With advancements in technology, healthcare providers can now identify diseases at their nascent stages, allowing for timely intervention.
- Enhanced diagnostic tools, such as AI and machine learning algorithms, analyze medical images and patient data to detect anomalies that may indicate early disease. Rapid Innovation leverages these technologies to develop tailored solutions that empower healthcare providers to make informed decisions swiftly.
- Regular screenings and preventive measures can lead to the identification of conditions like cancer, diabetes, and cardiovascular diseases before they progress. Our consulting services guide clients in implementing effective screening programs that align with best practices.
- Studies show that early detection can increase survival rates significantly. For instance, breast cancer detected at an early stage has a 99% five-year survival rate compared to 27% for late-stage detection (source: American Cancer Society). By integrating AI-driven analytics, we help clients enhance their diagnostic capabilities, ultimately improving patient outcomes.
- Implementing population health management strategies can help identify at-risk individuals and facilitate early intervention. Rapid Innovation assists healthcare organizations in developing data-driven strategies that target high-risk populations effectively.
- Telemedicine and remote monitoring technologies enable continuous health assessments, allowing for quicker responses to potential health issues. Our expertise in blockchain ensures secure and efficient data sharing, enhancing the effectiveness of telehealth solutions.
4.3. Workflow Optimization
Workflow optimization in healthcare refers to streamlining processes to enhance efficiency and improve patient care. By refining workflows, healthcare facilities can reduce waste, minimize errors, and ensure that resources are utilized effectively.
- Implementing electronic health records (EHR) systems can centralize patient information, making it easily accessible to healthcare providers. Rapid Innovation specializes in developing customized EHR solutions that integrate seamlessly with existing systems.
- Standardizing procedures and protocols can reduce variability in care delivery, leading to improved patient outcomes. Our consulting services help clients establish best practices that enhance care consistency.
- Utilizing data analytics can help identify bottlenecks in workflows, allowing for targeted improvements. We provide advanced analytics solutions that empower healthcare organizations to make data-driven decisions.
- Training staff on best practices and new technologies can enhance productivity and job satisfaction. Rapid Innovation offers comprehensive training programs to ensure staff are well-equipped to utilize new systems effectively.
- Automation of routine tasks, such as appointment scheduling and billing, can free up staff to focus on patient care. Our automation solutions streamline administrative processes, allowing healthcare providers to allocate more time to patient interactions.
- A well-optimized workflow can lead to shorter patient wait times and increased patient throughput, ultimately improving the overall patient experience. By implementing our solutions, clients can achieve significant improvements in operational efficiency.
4.4. Reduced Reading Time
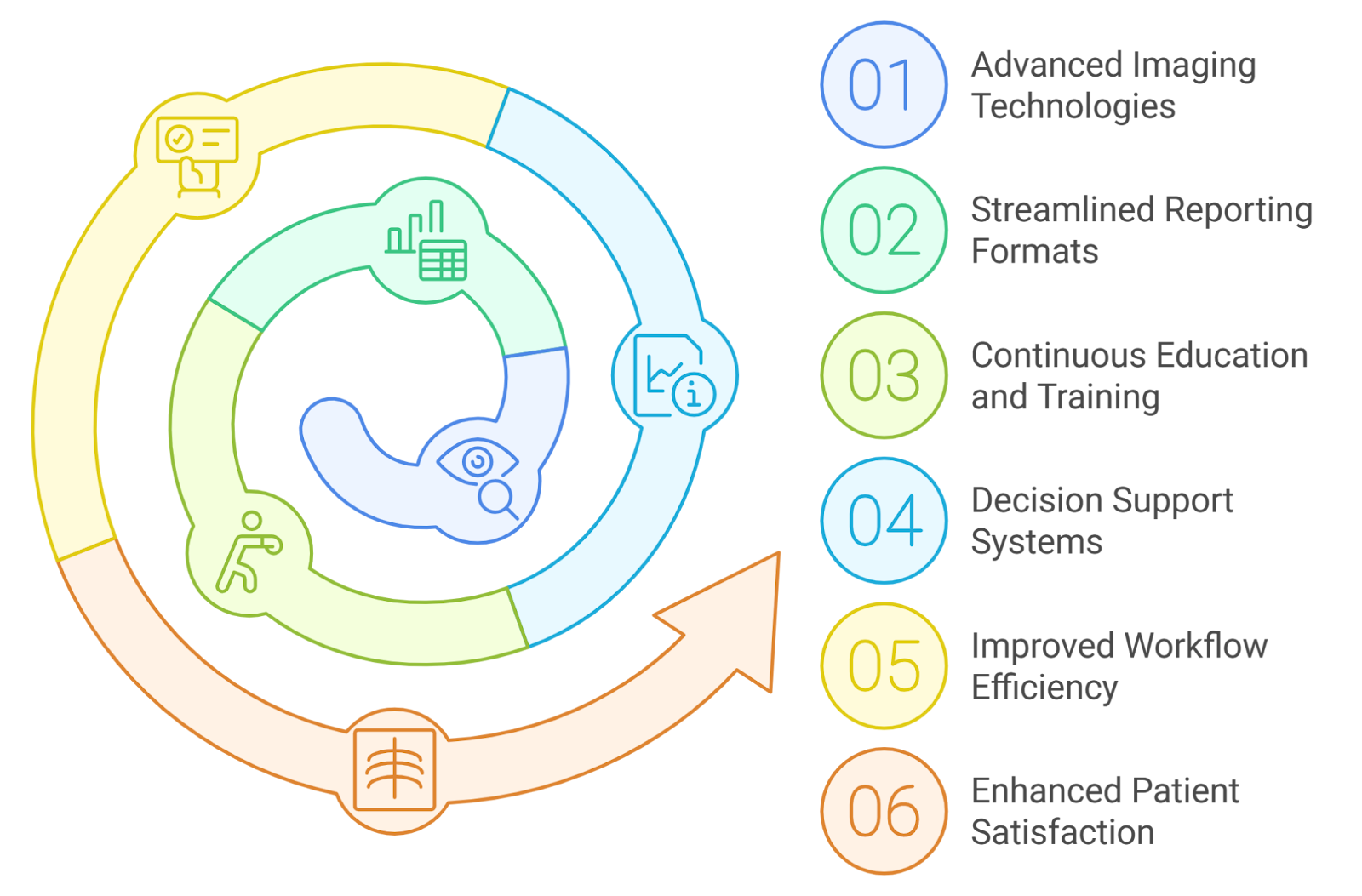
‍
Reduced reading time in healthcare refers to the efficiency gained in interpreting medical data, images, and reports. This is particularly important in radiology and pathology, where timely diagnosis can significantly impact patient care.
- Advanced imaging technologies and AI-assisted tools can expedite the analysis of medical images, allowing radiologists to focus on critical findings. Rapid Innovation develops AI solutions that enhance image analysis, leading to faster and more accurate diagnoses.
- Streamlined reporting formats can present essential information more clearly, reducing the time needed to interpret results. Our team works with clients to design intuitive reporting systems that facilitate quick decision-making.
- Continuous education and training for healthcare professionals can enhance their ability to quickly assess and interpret data. We provide ongoing training resources to ensure healthcare professionals stay updated on the latest technologies and methodologies.
- Implementing decision support systems can provide clinicians with relevant information at their fingertips, aiding in faster decision-making. Our expertise in AI allows us to create sophisticated decision support tools that enhance clinical workflows.
- Reducing reading time not only improves workflow efficiency but also enhances patient satisfaction by providing quicker diagnoses and treatment plans.
- Studies indicate that AI can reduce the time radiologists spend on image interpretation by up to 50%, allowing for more cases to be reviewed in a shorter period (source: Radiology Business). Rapid Innovation's AI solutions are designed to maximize efficiency, ultimately leading to improved patient care and greater ROI for healthcare organizations.
4.5. Standardization of Analysis
Standardization of analysis in healthcare refers to the establishment of consistent methods and protocols for evaluating healthcare data and outcomes. This is crucial for ensuring that healthcare practices are based on reliable and comparable information.
- Promotes uniformity in data collection and interpretation. Â
- Facilitates the comparison of results across different studies and healthcare settings. Â
- Enhances the reliability of clinical guidelines and recommendations. Â
- Supports regulatory compliance and quality assurance in healthcare practices. Â
- Encourages the use of evidence-based practices, leading to improved patient outcomes. Â
At Rapid Innovation, we leverage AI-driven analytics to standardize healthcare data analysis, enabling providers to make informed decisions based on consistent metrics. This not only enhances patient care but also drives operational efficiencies, ultimately leading to greater ROI for healthcare organizations. Our focus on healthcare data analytics and predictive analytics in healthcare allows us to refine our methodologies continuously.
4.6. Support for Rural Healthcare
Support for rural healthcare is essential to address the unique challenges faced by rural communities. These areas often experience limited access to medical services, healthcare professionals, and resources.
- Increases access to essential healthcare services for rural populations. Â
- Promotes telemedicine and mobile health initiatives to reach remote areas. Â
- Encourages partnerships between urban and rural healthcare facilities to share resources. Â
- Provides training and support for healthcare professionals working in rural settings. Â
- Addresses social determinants of health that disproportionately affect rural communities. Â
Rapid Innovation utilizes blockchain technology to create secure and efficient telehealth platforms, ensuring that rural populations receive timely medical attention. By bridging the gap between urban and rural healthcare, we contribute to reducing health disparities and improving quality of life through healthcare analytics platform initiatives.
4.7. Cost-Effectiveness
Cost-effectiveness in healthcare refers to the evaluation of the economic efficiency of medical interventions and treatments. It is a critical factor in decision-making for healthcare providers, policymakers, and patients.
- Helps in determining the best use of limited healthcare resources. Â
- Assesses the value of treatments in relation to their costs and outcomes. Â
- Supports the implementation of cost-effective interventions that improve patient care. Â
- Encourages the adoption of preventive measures that can reduce long-term healthcare costs. Â
- Aids in prioritizing funding and resources for high-impact healthcare programs. Â
By focusing on cost-effectiveness, healthcare systems can ensure that they are providing high-quality care while managing expenses. Rapid Innovation's AI solutions enable healthcare organizations to analyze cost data effectively, ensuring that resources are allocated to high-impact programs. Our expertise in big data analytics in healthcare and healthcare claims data analytics further enhances our ability to deliver sustainable solutions that benefit patients and healthcare systems alike.
5. Technical Implementation
5.1. Data Collection and Preparation
Data collection and preparation are critical steps in any technical implementation, especially in fields like machine learning, computer vision, and data analysis. The quality and relevance of the data directly influence the performance of the models and systems being developed. This includes preparation for data collection, data collection and preparation, and any process of preparing and collecting data.
- Importance of data quality: Â
- High-quality data leads to better model accuracy, which translates to improved decision-making and operational efficiency for businesses.
- Clean and well-structured data reduces processing time, allowing for faster insights and quicker responses to market changes.
- Diverse datasets help in building robust models that can generalize well across different scenarios, enhancing the reliability of AI solutions.
- Steps in data preparation: Â
- Data cleaning: Removing duplicates, correcting errors, and handling missing values ensures that the data used for training is accurate and reliable.
- Data transformation: Normalizing or standardizing data to ensure consistency is essential for effective model training and performance.
- Data augmentation: Enhancing the dataset by creating variations of existing data, especially in image datasets, can significantly improve model robustness and accuracy.
5.1.1. Image Acquisition
Image acquisition is a fundamental aspect of data collection in projects involving computer vision and image processing. It involves capturing images that will be used for training, testing, and validating models.
- Methods of image acquisition: Â
- Digital cameras: High-resolution cameras can capture detailed images for analysis, ensuring that the data quality meets the requirements of advanced AI models.
- Smartphones: Convenient and widely available, smartphones can be used for quick image capture, making data collection more accessible and cost-effective.
- Scanners: Useful for digitizing printed images or documents, scanners can help in creating a comprehensive dataset from existing materials.
- Considerations for image acquisition: Â
- Resolution: Higher resolution images provide more detail but require more storage and processing power, which should be balanced against project needs and resources.
- Lighting conditions: Proper lighting is essential to avoid shadows and reflections that can distort images, ensuring that the data collected is of the highest quality.
- Angle and perspective: Capturing images from various angles can enhance the dataset's diversity, leading to more robust model training.
- Sources of images: Â
- Public datasets: Many organizations provide free access to large image datasets for research purposes, which can be leveraged to jumpstart projects.
- Web scraping: Automated tools can collect images from websites, but ethical considerations must be taken into account to ensure compliance with regulations.
- User-generated content: Crowdsourcing can be an effective way to gather diverse images from a wide audience, enriching the dataset and improving model performance.
- Tools for image acquisition: Â
- OpenCV: A popular library for image processing that can also assist in capturing images from cameras, facilitating efficient data collection.
- TensorFlow: Offers tools for image data handling and preprocessing, streamlining the workflow from data collection to model training.
- ImageJ: A powerful image processing program that can be used for scientific image analysis, providing advanced capabilities for data preparation.
By focusing on effective data collection and preparation, including image acquisition, Rapid Innovation ensures that projects achieve better outcomes and more reliable results, ultimately leading to greater ROI for our clients. This comprehensive approach to data gathering and preparation is essential for successful technical implementation.
5.1.2. Data Cleaning
Data cleaning is a crucial step in the data preparation process, ensuring that the dataset is accurate, consistent, and usable for analysis or model training. This process involves identifying and rectifying errors or inconsistencies in the data.
- Remove duplicates: Duplicate entries can skew results and lead to inaccurate conclusions. Identifying and removing these duplicates is essential.
- Handle missing values: Missing data can occur for various reasons. Strategies include: Â
- Imputation: Filling in missing values using statistical methods.
- Deletion: Removing records with missing values if they are not significant.
- Correct inconsistencies: Data may come from various sources, leading to inconsistencies in formats (e.g., date formats, capitalization). Standardizing these formats is vital.
- Outlier detection: Identifying and addressing outliers can prevent them from disproportionately influencing the model. Techniques include: Â
- Statistical methods (e.g., Z-scores).
- Visualization tools (e.g., box plots).
- Validate data accuracy: Ensuring that the data reflects real-world conditions is essential. This can involve cross-referencing with reliable sources or conducting manual checks.
Effective data cleaning and annotation enhance the quality of the dataset, leading to more reliable and valid results in subsequent analyses or model training. At Rapid Innovation, we leverage advanced AI techniques to automate and optimize the data cleaning process, ensuring that our clients can trust the integrity of their data, which ultimately leads to greater ROI.
5.1.3. Annotation Methods
Annotation methods are essential for preparing data, especially in supervised machine learning tasks. They involve labeling data to provide context and meaning, enabling models to learn from the data effectively.
- Manual annotation: Human annotators review and label data. This method is often used for complex tasks where context is crucial, such as image classification and sentiment analysis in text.
- Automated annotation: Algorithms or software tools automatically label data. This method is faster but may require initial manual validation to ensure accuracy.
- Semi-automated annotation: A combination of manual and automated methods. For instance, an algorithm may suggest labels, which human annotators then verify or adjust.
- Crowdsourcing: Leveraging a large group of people to annotate data can speed up the process. Platforms like Amazon Mechanical Turk allow for diverse input but require quality control measures.
- Quality assurance: Regardless of the method used, implementing quality checks is vital. This can include: Â
- Inter-annotator agreement: Measuring consistency among different annotators.
- Regular audits: Periodically reviewing annotated data for accuracy.
Choosing the right annotation method depends on the specific requirements of the project, including the complexity of the data and the resources available. Rapid Innovation employs a tailored approach to annotation, ensuring that our clients' datasets are accurately labeled, which is critical for effective model training and ultimately enhances their business outcomes.
5.2. Model Development
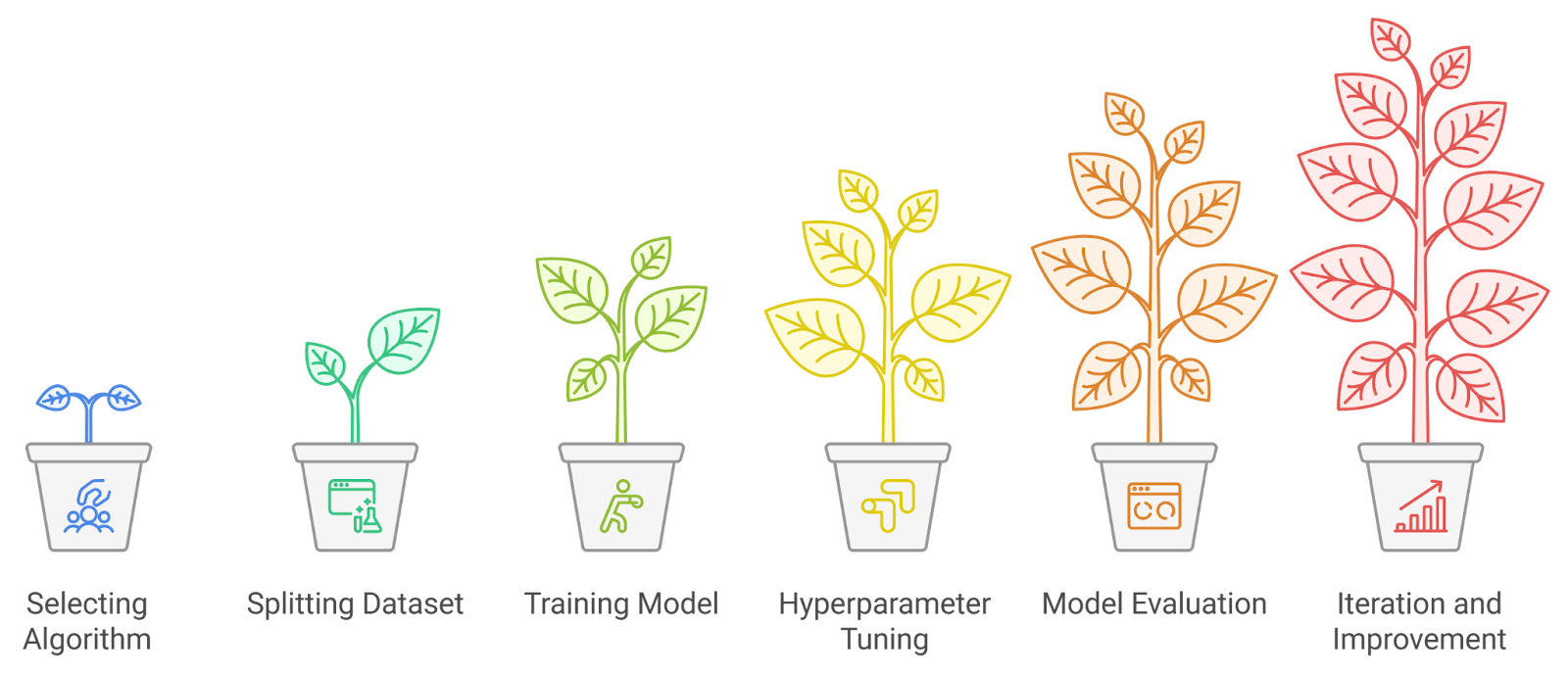
‍
Model development is the process of creating a predictive model using the cleaned and annotated data. This phase involves several key steps that ensure the model is effective and reliable.
- Selecting the right algorithm: The choice of algorithm depends on the nature of the data and the problem being solved. Common algorithms include: Â
- Linear regression for continuous outcomes.
- Decision trees for classification tasks.
- Neural networks for complex patterns.
- Splitting the dataset: Dividing the dataset into training, validation, and test sets is crucial for evaluating model performance. Typical splits include: Â
- 70% training, 15% validation, 15% testing.
- Training the model: This involves feeding the training data into the selected algorithm, allowing it to learn patterns and relationships within the data.
- Hyperparameter tuning: Adjusting the model's parameters to optimize performance. Techniques include: Â
- Grid search: Testing various combinations of parameters.
- Random search: Randomly sampling parameter combinations.
- Model evaluation: Assessing the model's performance using the validation set. Key metrics include: Â
- Accuracy: The proportion of correct predictions.
- Precision and recall: Important for imbalanced datasets.
- F1 score: A balance between precision and recall.
- Iteration and improvement: Based on evaluation results, the model may require adjustments. This could involve: Â
- Revisiting data cleaning or annotation.
- Trying different algorithms or tuning parameters further.
Effective model development is essential for creating robust predictive models that can provide valuable insights and drive decision-making. At Rapid Innovation, we focus on delivering high-quality models that not only meet our clients' needs but also enhance their operational efficiency and profitability.
5.2.1. Architecture Selection
Choosing the right architecture is crucial for the success of any machine learning or deep learning project. The architecture serves as the backbone of the model, influencing its performance, scalability, and ability to generalize. At Rapid Innovation, we leverage our expertise in AI to guide clients in selecting the most suitable architecture tailored to their specific business needs, ensuring optimal outcomes.
- Types of Architectures: Â
- Convolutional Neural Networks (CNNs) are ideal for image processing tasks, enabling businesses to enhance their visual data analysis capabilities.
- Recurrent Neural Networks (RNNs) are suited for sequential data, such as time series or natural language, allowing organizations to derive insights from historical data trends.
- Transformer models have gained popularity for their efficiency in handling large datasets and parallel processing, making them a powerful choice for complex applications. This includes architectures like transformer architecture deep learning, which are particularly effective in natural language processing tasks.
- Factors to Consider: Â
- Complexity: More complex architectures, such as lstm networks, may yield better results but require more computational resources. Rapid Innovation helps clients balance complexity with resource availability to maximize ROI.
- Data Availability: The amount and type of data available can dictate the choice of architecture. For instance, CNNs require large labeled datasets for effective training, and we assist clients in data preparation and augmentation strategies. This is particularly relevant in the context of machine learning data architecture.
- Task Requirements: Different tasks may necessitate different architectures. For example, a simple linear model may suffice for basic regression tasks, while complex tasks like image recognition may require deep learning architectures. Our team evaluates task requirements to recommend the most effective solutions, including machine learning model architecture and architecture for machine learning.
- Performance Metrics: Â
- Evaluate architectures based on metrics such as accuracy, precision, recall, and F1 score to determine the best fit for your specific application. Rapid Innovation emphasizes the importance of these metrics in aligning technology with business objectives, particularly in the context of machine learning pipeline architecture.
5.2.2. Training Strategies
Training strategies are essential for optimizing model performance and ensuring that the model learns effectively from the data. The choice of training strategy can significantly impact the model's ability to generalize to unseen data, and at Rapid Innovation, we implement best practices to enhance model efficacy.
- Batch Size: Â
- Smaller batch sizes can lead to better generalization but may increase training time.
- Larger batch sizes can speed up training but may lead to overfitting.
- Learning Rate: Â
- A well-tuned learning rate is critical. Too high a learning rate can cause the model to converge too quickly to a suboptimal solution, while too low a learning rate can result in prolonged training times.
- Regularization Techniques: Â
- Techniques such as dropout, L1/L2 regularization, and data augmentation can help prevent overfitting and improve model robustness.
- Transfer Learning: Â
- Utilizing pre-trained models can significantly reduce training time and improve performance, especially when working with limited data. Rapid Innovation employs transfer learning to accelerate project timelines and enhance results, particularly in the context of machine learning operations overview definition and architecture.
- Early Stopping: Â
- Monitoring validation loss during training can help in implementing early stopping, which halts training when performance on the validation set begins to degrade.
5.2.3. Validation Approaches
Validation is a critical step in the machine learning pipeline, ensuring that the model performs well on unseen data. Effective validation approaches help in assessing the model's generalization capabilities, and Rapid Innovation employs rigorous validation techniques to ensure client success.
- Cross-Validation: Â
- K-Fold Cross-Validation is a popular method where the dataset is divided into K subsets. The model is trained K times, each time using a different subset as the validation set and the remaining data for training. This method provides a more reliable estimate of model performance.
- Train-Test Split: Â
- A simple yet effective approach where the dataset is divided into a training set and a test set. The model is trained on the training set and evaluated on the test set to gauge its performance.
- Holdout Method: Â
- Similar to train-test split, but typically involves setting aside a portion of the data (e.g., 20%) for validation before training begins.
- Performance Metrics: Â
- Use metrics such as accuracy, precision, recall, and ROC-AUC to evaluate model performance during validation. These metrics provide insights into how well the model is likely to perform in real-world scenarios.
- Confusion Matrix: Â
- A confusion matrix can help visualize the performance of the model, showing true positives, false positives, true negatives, and false negatives, which aids in understanding where the model may be making errors.
- Hyperparameter Tuning: Â
- Validation approaches can also be used to fine-tune hyperparameters, ensuring that the model is optimized for the best performance. Techniques like Grid Search or Random Search can be employed for this purpose. Rapid Innovation's expertise in hyperparameter tuning ensures that our clients achieve the highest possible model performance, translating to greater ROI, particularly in the context of mlops architecture and aws machine learning architecture.
5.3. Integration with Clinical Workflows
Integration with clinical workflows is essential for enhancing the efficiency and effectiveness of healthcare delivery. By seamlessly connecting various systems and processes, healthcare providers can improve patient care, reduce errors, and streamline operations. Effective integration allows for better data sharing, communication, and collaboration among healthcare professionals. Key benefits include:
- Enhances patient safety by reducing the risk of errors.
- Improves the speed of information retrieval.
- Facilitates better decision-making through comprehensive data access.
- Supports regulatory compliance and reporting requirements.
- Increases overall operational efficiency.
At Rapid Innovation, we leverage our expertise in AI and Blockchain to facilitate this integration, ensuring that healthcare organizations can achieve greater ROI through enhanced operational capabilities, including integrated medical services and integrated health services.
5.3.1. PACS Integration
Picture Archiving and Communication Systems (PACS) integration is crucial for managing medical imaging data. PACS allows healthcare providers to store, retrieve, and share images digitally, eliminating the need for physical film. Integrating PACS with other clinical systems enhances workflow efficiency and improves patient care. The integration offers several advantages:
- Streamlines access to imaging data for radiologists and clinicians.
- Reduces the time taken to retrieve images, leading to faster diagnoses.
- Facilitates collaboration among specialists by enabling easy sharing of images.
- Supports advanced imaging techniques and modalities.
- Enhances the ability to track and manage imaging studies.
PACS integration can also improve the overall patient experience by providing timely access to imaging results. This integration ensures that healthcare providers have the necessary information at their fingertips, allowing for more informed clinical decisions. Rapid Innovation employs AI algorithms to optimize image processing and retrieval, further enhancing the efficiency of PACS systems, which is a key aspect of integrated healthcare providers.
5.3.2. EMR/EHR Integration
Electronic Medical Records (EMR) and Electronic Health Records (EHR) integration is vital for creating a comprehensive view of a patient's health history. EMR/EHR systems store patient data, including medical history, medications, allergies, and treatment plans. Integrating these systems with other clinical workflows enhances data accessibility and improves care coordination. The benefits of EMR/EHR integration include:
- Provides a holistic view of patient health information.
- Reduces duplication of tests and procedures.
- Enhances communication between different healthcare providers.
- Supports clinical decision support tools for better patient outcomes.
- Facilitates billing and coding processes through accurate data capture.
Effective EMR/EHR integration can lead to improved patient engagement, as patients can access their health information and participate in their care. This integration also supports population health management initiatives by enabling healthcare organizations to analyze data trends and improve overall health outcomes. At Rapid Innovation, we utilize Blockchain technology to ensure the security and integrity of patient data, fostering trust and compliance in healthcare environments, which is essential for integrated healthcare services and mobile integrated healthcare. For more information on how NLP applications can enhance these processes, visit NLP Applications in Healthcare.
5.3.3. Cloud Infrastructure
Cloud infrastructure refers to the collection of hardware and software components that are required to support cloud computing, including IaaS (Infrastructure as a Service). This infrastructure is essential for delivering services such as storage, networking, and computing power over the internet.
- Components of Cloud Infrastructure: Â
- Physical Servers: These are the backbone of cloud services, providing the necessary computing power.
- Storage Systems: Cloud storage solutions allow for scalable data storage, enabling users to access their data from anywhere.
- Networking Equipment: Routers, switches, and firewalls are crucial for ensuring secure and efficient data transfer.
- Virtualization Software: This technology allows multiple virtual machines to run on a single physical server, optimizing resource use.
- Management Tools: These tools help in monitoring, managing, and automating cloud resources.
- Types of Cloud Infrastructure: Â
- Public Cloud: Services are offered over the public internet and shared across multiple organizations.
- Private Cloud: Infrastructure is dedicated to a single organization, providing enhanced security and control, often referred to as a private cloud in cloud computing.
- Hybrid Cloud: Combines both public and private clouds, allowing for greater flexibility and scalability.
- Benefits of Cloud Infrastructure: Â
- Scalability: Easily scale resources up or down based on demand, a key feature of IaaS services.
- Cost Efficiency: Reduces the need for physical hardware and maintenance costs.
- Accessibility: Access data and applications from anywhere with an internet connection.
- Disaster Recovery: Enhanced data backup and recovery options.
- Key Providers: Major cloud infrastructure providers include Amazon Web Services (AWS), Microsoft Azure, Google Cloud Platform (GCP), and Oracle Cloud Infrastructure services, each offering a range of services tailored to different business needs.
6. Challenges and Limitations
While cloud infrastructure offers numerous advantages, it also presents several challenges and limitations that organizations must navigate to maximize their cloud investments.
- Security Concerns: Â
- Data breaches and unauthorized access are significant risks.
- Compliance with regulations such as GDPR and HIPAA can be complex.
- Downtime and Reliability: Â
- Cloud services can experience outages, impacting business operations.
- Organizations must assess the reliability of their chosen cloud provider.
- Cost Management: Â
- While cloud services can be cost-effective, unexpected costs can arise from overuse or mismanagement of resources.
- Organizations need to implement effective budgeting and monitoring strategies.
- Vendor Lock-In: Â
- Switching providers can be challenging due to proprietary technologies and data migration issues.
- Organizations should consider multi-cloud strategies to mitigate this risk.
- Performance Issues: Â
- Latency and bandwidth limitations can affect application performance.
- Organizations must evaluate their network infrastructure to ensure optimal performance.
6.1. Technical Challenges

‍
Technical challenges in cloud infrastructure can hinder the effective deployment and management of cloud services. Understanding these challenges is crucial for organizations looking to leverage cloud technology.
- Integration with Legacy Systems: Â
- Many organizations have existing legacy systems that may not easily integrate with cloud solutions, leading to data silos and inefficiencies.
- Data Migration: Â
- Moving data to the cloud can be complex and time-consuming. Organizations must plan for data integrity and security during the migration process, especially when considering IaaS in cloud computing.
- Skill Gaps: Â
- There is often a shortage of skilled professionals who understand cloud technologies. Organizations may need to invest in training or hire specialized talent.
- Compliance and Regulatory Issues: Â
- Navigating compliance requirements can be challenging, especially for industries with strict regulations. Organizations must ensure that their cloud provider meets all necessary compliance standards.
- Resource Management: Â
- Managing cloud resources effectively can be difficult, especially in dynamic environments. Organizations need robust monitoring and management tools to optimize resource use, particularly in IaaS services.
- Performance Monitoring: Â
- Ensuring consistent performance across cloud services requires ongoing monitoring. Organizations must implement performance metrics and analytics to identify and address issues promptly.
At Rapid Innovation, we understand these challenges and are equipped to help organizations navigate them effectively. Our expertise in AI and Blockchain can enhance cloud infrastructure management, ensuring that businesses achieve greater ROI through optimized resource allocation, improved security protocols, and seamless integration with existing systems. By leveraging our consulting solutions, clients can transform their cloud strategies, ensuring they are not only efficient but also aligned with their overall business goals, including the adoption of top cloud computing providers and cloud-based infrastructure solutions.
6.1.1. Data Quality Issues
Data quality is a critical factor in the success of any data-driven project. Poor data quality can lead to inaccurate insights, flawed models, and ultimately, misguided business decisions. Key issues related to data quality include:
- Incomplete Data: Missing values can skew results and lead to incorrect conclusions. It is essential to identify and address gaps in the dataset. Rapid Innovation employs advanced data imputation techniques to fill these gaps, ensuring that your analyses are based on complete datasets.
- Inconsistent Data: Variations in data formats, units, or naming conventions can create confusion. Standardizing data is crucial for effective analysis. Our team utilizes data normalization processes to ensure consistency across all data points, enhancing the reliability of your insights.
- Noisy Data: Outliers and errors can distort the analysis. Techniques such as data cleaning and preprocessing are necessary to mitigate these issues. Rapid Innovation implements robust data cleaning algorithms that effectively identify and rectify noise, leading to more accurate models.
- Biased Data: If the data collected is not representative of the target population, it can lead to biased models. Ensuring diversity in data sources is vital. We assist clients in diversifying their data collection strategies, which helps in building more equitable and effective models.
- Data Validation: Regular checks and validation processes can help maintain data integrity. Implementing automated validation tools can streamline this process. Rapid Innovation offers automated data validation solutions that continuously monitor data quality, ensuring that your datasets remain reliable over time.
- Data Quality Challenges: Organizations often face data quality challenges that can hinder their ability to make informed decisions. Identifying these challenges early on is crucial for effective data management.
- Data Quality Issues in Healthcare: In the healthcare sector, data quality issues can have serious implications for patient care and outcomes. Addressing these issues is essential for improving healthcare delivery.
- Common Data Quality Problems: Understanding common data quality problems can help organizations proactively address potential issues before they escalate.
- Poor Data Quality Costs: The costs associated with poor data quality can be significant, impacting both operational efficiency and decision-making processes.
- Data Quality Issues and Solutions: Implementing effective solutions to address data quality issues is vital for maintaining the integrity of your datasets. For more insights on addressing these challenges, check out common issues in computer vision models.
- Data Quality Observability: Establishing data quality observability allows organizations to monitor and assess data quality in real-time, ensuring ongoing reliability.
6.1.2. Model Generalization
Model generalization refers to a model's ability to perform well on unseen data, not just the data it was trained on. Achieving good generalization is essential for the model's practical application. Important aspects of model generalization include:
- Overfitting: This occurs when a model learns the training data too well, capturing noise rather than the underlying pattern. Techniques like cross-validation can help detect overfitting. Our experts at Rapid Innovation implement rigorous cross-validation techniques to ensure that your models generalize well to new data.
- Underfitting: A model that is too simple may fail to capture the complexity of the data. Striking a balance between complexity and simplicity is key. We work closely with clients to develop models that are appropriately complex, ensuring they capture essential patterns without becoming unwieldy.
- Regularization: Methods such as L1 and L2 regularization can help improve generalization by penalizing overly complex models. Rapid Innovation employs these regularization techniques to enhance model performance, ensuring that your solutions are both effective and efficient.
- Feature Selection: Choosing the right features can enhance a model's ability to generalize. Techniques like recursive feature elimination can aid in this process. Our team utilizes advanced feature selection methods to identify the most impactful variables, optimizing model performance.
- Testing on Diverse Datasets: Evaluating the model on various datasets can provide insights into its generalization capabilities. This practice helps ensure robustness across different scenarios. Rapid Innovation conducts extensive testing across diverse datasets to validate model performance, ensuring reliability in real-world applications.
6.1.3. Computational Requirements
The computational requirements of a model can significantly impact its feasibility and performance. Understanding these requirements is essential for effective implementation. Key considerations include:
- Processing Power: Complex models often require substantial computational resources. Utilizing cloud computing or high-performance computing can alleviate these demands. Rapid Innovation leverages cloud infrastructure to provide scalable solutions that meet your processing needs without compromising performance.
- Memory Usage: Large datasets can consume significant memory. Efficient data handling techniques, such as batch processing, can help manage memory usage. Our team implements memory-efficient algorithms that optimize data handling, ensuring smooth operations even with large datasets.
- Training Time: The time it takes to train a model can vary widely. Optimizing algorithms and using parallel processing can reduce training time. Rapid Innovation focuses on algorithm optimization and parallel processing techniques to minimize training time, allowing for quicker deployment of your AI solutions.
- Scalability: As data volume grows, the model should be able to scale accordingly. Designing models with scalability in mind ensures long-term viability. We prioritize scalability in our model designs, ensuring that your solutions can grow alongside your business needs.
- Cost Considerations: High computational requirements can lead to increased costs. Budgeting for resources and exploring cost-effective solutions is crucial for project sustainability. Rapid Innovation provides cost-effective strategies that balance performance and budget, ensuring that your projects remain financially viable.
6.2. Clinical Challenges
Clinical challenges in healthcare often arise from the integration of new technologies, treatment protocols, and patient management strategies. These challenges can significantly impact the effectiveness of clinical practices and patient outcomes. Addressing these clinical challenges in healthcare is crucial for improving healthcare delivery and ensuring patient safety.
- Integration of new technologies can lead to resistance from healthcare professionals, which can be mitigated through tailored AI solutions that facilitate smoother transitions and enhance user experience.
- Variability in patient responses to treatments complicates clinical decision-making. Leveraging AI analytics can help predict patient outcomes more accurately, allowing for personalized treatment plans that improve efficacy.
- Regulatory hurdles can delay the implementation of innovative therapies. Rapid Innovation can assist clients in navigating these complexities by providing blockchain-based solutions that ensure compliance and streamline documentation processes.
- Ensuring data security and patient privacy is paramount in clinical settings. Our blockchain expertise enables secure data sharing and storage, ensuring that patient information remains confidential and protected against breaches.
- Continuous training and education are necessary to keep healthcare providers updated. Rapid Innovation offers AI-driven training modules that adapt to the learning pace of healthcare professionals, ensuring they are well-equipped to utilize new technologies effectively. For more insights on how AI can enhance personalized care.
6.2.1. Validation and Testing
Validation and testing are critical components in the development of new medical technologies and treatments. These processes ensure that new interventions are safe, effective, and reliable before they are widely adopted in clinical practice.
- Rigorous clinical trials are essential to assess the efficacy of new treatments. Our AI solutions can optimize trial designs and patient recruitment, enhancing the speed and accuracy of results.
- Validation involves comparing new methods against established standards. Rapid Innovation can provide data analytics tools that facilitate this comparison, ensuring that new interventions meet or exceed existing benchmarks.
- Testing must include diverse patient populations to ensure generalizability. Our AI algorithms can analyze data from varied demographics, helping to ensure that treatments are effective across different patient groups.
- Regulatory bodies, such as the FDA, require extensive data before approval. We assist clients in compiling and presenting this data effectively, leveraging our expertise in both AI and blockchain for transparent reporting.
- Post-market surveillance is necessary to monitor long-term effects and safety. Our solutions can automate data collection and analysis, providing ongoing insights into treatment performance.
6.2.2. Clinical Acceptance
Clinical acceptance refers to the willingness of healthcare professionals to adopt new technologies and treatment protocols. This acceptance is influenced by various factors, including perceived benefits, ease of use, and the overall impact on patient care.
To enhance clinical acceptance, it is essential to consider the following:
- Evidence-based research is crucial for gaining clinician trust in new interventions. Rapid Innovation can support clients in conducting and disseminating research that highlights the effectiveness of their solutions.
- Training and support can facilitate smoother transitions to new practices. Our AI-driven training programs are designed to meet the specific needs of healthcare providers, ensuring they feel confident in using new technologies.
- Peer influence and recommendations from respected colleagues can enhance acceptance. We can help clients develop strategies to engage key opinion leaders in their fields, fostering a culture of innovation.
- Addressing concerns about workflow disruptions is vital for successful implementation. Our solutions are designed with user experience in mind, minimizing disruptions and enhancing overall efficiency.
- Continuous feedback from clinicians can help refine new technologies for better integration. Rapid Innovation emphasizes the importance of iterative development, using AI to analyze feedback and make necessary adjustments in real-time.
6.2.3. Integration with Existing Workflows
Integrating new technologies or systems into existing workflows is crucial for maximizing efficiency and minimizing disruption. This process involves several key considerations:
- Assessment of Current Workflows: Before integration, it’s essential to analyze existing workflows to identify areas that can benefit from new technology, such as technology integration in education. This includes understanding the roles of team members, the tools they currently use, and the overall process flow.
- Compatibility: Ensure that the new system is compatible with existing tools and software. This may involve checking APIs, data formats, and user interfaces to facilitate seamless communication between systems, particularly in tech integration in the classroom.
- Training and Support: Providing adequate training for employees is vital. Users must understand how to utilize the new technology effectively within their current workflows, especially when integrating technology in the classroom. Ongoing support should also be available to address any issues that arise post-integration.
- Incremental Implementation: Instead of a full-scale rollout, consider an incremental approach. This allows teams to adapt gradually, reducing resistance to change and minimizing disruptions, which is particularly important in models of technology integration.
- Feedback Mechanism: Establish a feedback loop to gather insights from users about the integration process. This can help identify pain points and areas for improvement, ensuring that the integration meets the needs of the organization, including evidence-based instructional technology integration.
- Monitoring and Evaluation: After integration, continuously monitor the performance of the new system within existing workflows. Evaluate its impact on productivity, efficiency, and user satisfaction to make necessary adjustments, particularly in the context of technology integration planning.
6.3. Ethical Considerations
Ethical considerations are paramount when implementing new technologies, particularly in areas such as data handling, user interaction, and overall impact on society. Organizations must navigate these considerations carefully to maintain trust and compliance.
- Transparency: Organizations should be transparent about how they use technology and data. This includes informing users about data collection practices and the purpose behind them, especially in the context of defining technology integration.
- Accountability: Establish clear accountability for decisions made regarding technology use. This involves defining who is responsible for ethical considerations and ensuring that there are mechanisms in place to address any ethical breaches.
- Inclusivity: Ensure that technology is accessible to all users, regardless of their background or abilities. This promotes fairness and prevents discrimination in technology use, which is essential in integrating digital technologies.
- Impact Assessment: Conduct assessments to understand the potential societal impacts of new technologies. This includes evaluating how technology may affect employment, privacy, and social interactions, particularly in the integration of technology into education.
6.3.1. Privacy and Security
Privacy and security are critical components of ethical considerations in technology implementation. Organizations must prioritize these aspects to protect user data and maintain trust.
- Data Protection Policies: Develop and enforce robust data protection policies that comply with relevant regulations, such as GDPR or CCPA. This includes outlining how data is collected, stored, and used.
- Encryption and Security Measures: Implement strong encryption methods and security protocols to safeguard sensitive information. Regularly update these measures to counter evolving cyber threats.
- User Consent: Obtain explicit consent from users before collecting or processing their data. This ensures that users are aware of what data is being collected and how it will be used.
- Regular Audits: Conduct regular security audits to identify vulnerabilities and ensure compliance with privacy policies. This proactive approach helps mitigate risks associated with data breaches.
- Incident Response Plan: Establish a clear incident response plan to address potential data breaches or security incidents. This plan should outline steps for containment, investigation, and communication with affected users.
- User Education: Educate users about privacy and security best practices. This includes informing them about how to protect their own data and recognize potential threats.
By focusing on these areas, organizations can effectively integrate new technologies into existing workflows while addressing ethical considerations, particularly regarding privacy and security. At Rapid Innovation, we specialize in guiding organizations through this integration process, ensuring that the adoption of AI and Blockchain technologies aligns with their business goals while maintaining ethical standards and enhancing overall efficiency, including the role of tech integrators in this process.
6.3.2. Bias in AI Systems
Bias in AI systems is a significant concern that can lead to unfair treatment and discrimination. AI algorithms are trained on data sets that may reflect historical biases, which can perpetuate and even amplify these biases in decision-making processes. AI systems can exhibit bias in various forms, including Data Bias, which occurs when the training data is not representative of the entire population, leading to skewed results; Algorithmic Bias, where the algorithms themselves are designed in a way that favors certain outcomes over others; and Human Bias, which happens when developers unintentionally introduce their own biases into the AI system during the design and training phases.
The implications of biased AI systems can be severe, affecting areas such as Hiring Practices, where AI tools used for recruitment may favor candidates from certain demographics, leading to discrimination; Law Enforcement, where predictive policing algorithms can disproportionately target specific communities based on biased historical data; and Healthcare, where AI systems may provide unequal treatment recommendations based on biased data, affecting patient outcomes.
At Rapid Innovation, we understand the critical importance of addressing bias in AI. Our team employs a multi-faceted approach, including regular audits of AI systems to identify and mitigate bias, utilizing diverse data sets that accurately represent the population, and involving ethicists and diverse teams in the development process to ensure a broader perspective. By implementing these strategies, we help our clients create fairer AI systems, ultimately leading to improved trust and greater ROI. For more information on how we can assist you, visit our AI agent services and our ethical AI development guide.
6.3.3. Liability Issues
Liability issues in AI systems arise from the complexities of determining responsibility when an AI system causes harm or makes erroneous decisions. As AI technology becomes more autonomous, the question of who is liable for its actions becomes increasingly complicated. Key considerations in AI liability include Product Liability, where manufacturers may be held liable under product liability laws if an AI system malfunctions or causes harm; Negligence, where developers and companies may face negligence claims if they fail to ensure the safety and reliability of their AI systems; and Vicarious Liability, where employers may be held responsible for the actions of AI systems used in their operations, especially if those actions lead to harm.
The legal landscape surrounding AI liability is still evolving, with challenges such as Attribution of Responsibility, which involves determining whether the fault lies with the AI developer, the user, or the AI itself; Regulatory Frameworks, highlighting the need for clear regulations that define liability in the context of AI technologies; and Insurance Models, which focus on developing insurance products that address the unique risks associated with AI systems.
To navigate these liability issues, stakeholders must engage in proactive risk management and establish clear guidelines for accountability in AI deployment. Rapid Innovation assists clients in understanding these complexities and developing robust frameworks that mitigate liability risks, ensuring a more secure and compliant AI implementation.
6.4. Regulatory Compliance
Regulatory compliance in the context of AI systems is crucial for ensuring that these technologies are developed and used responsibly. Governments and regulatory bodies are increasingly recognizing the need for frameworks that govern AI to protect consumers and society at large. Key aspects of regulatory compliance include Data Protection,
6.4.1. FDA Approval Process
The FDA (Food and Drug Administration) approval process is a critical pathway for ensuring that medical products, including drugs and devices, are safe and effective for public use. This process is essential for maintaining public health and safety.
- Preclinical Testing: Before a product can be tested in humans, it undergoes laboratory and animal studies to assess its safety and biological activity.
- Investigational New Drug (IND) Application: If preclinical results are promising, the manufacturer submits an IND application to the FDA, detailing the proposed clinical trial plan.
- Clinical Trials: The FDA oversees three phases of clinical trials: Â
- Phase 1: Focuses on safety and dosage in a small group of healthy volunteers.
- Phase 2: Expands the study to a larger group to evaluate effectiveness and side effects.
- Phase 3: Involves thousands of participants to confirm effectiveness, monitor side effects, and compare with standard treatments.
- New Drug Application (NDA): After successful trials, the manufacturer submits an NDA, which includes all data from the trials, proposed labeling, and information on manufacturing.
- FDA Review: The FDA reviews the NDA, which can take several months to years. They may request additional information or conduct inspections.
- Post-Marketing Surveillance: Once approved, the product is monitored for long-term effects and safety through post-marketing studies.
The FDA's rigorous approval process ensures that only safe and effective products reach the market, protecting public health. This includes various pathways such as accelerated approval fda and fda drug approval process, which can expedite the availability of important medications. The fda approval process drugs are designed to ensure that new therapies are thoroughly evaluated before reaching patients.
At Rapid Innovation, we leverage our expertise in AI and Blockchain to streamline compliance processes, enhance data security, and improve the efficiency of regulatory submissions. By integrating AI-driven analytics, we help clients predict outcomes and optimize their clinical trial designs, ultimately leading to faster fda drug approval and greater ROI. Our Blockchain solutions ensure secure and transparent data management, facilitating HIPAA compliance and enhancing trust in patient data handling. With our support, clients can navigate the complexities of international regulations more effectively, ensuring their products meet global standards while maximizing market potential. This includes utilizing the fda accelerated approval program and understanding the fda drug approval list for better strategic planning.
6.4.2. HIPAA Compliance
HIPAA (Health Insurance Portability and Accountability Act) compliance is essential for healthcare organizations to protect patient information and maintain confidentiality. HIPAA sets national standards for the protection of health information.
- Privacy Rule: Establishes standards for the protection of individuals' medical records and personal health information. It gives patients rights over their information, including the right to access and request corrections.
- Security Rule: Sets standards for safeguarding electronic protected health information (ePHI). This includes administrative, physical, and technical safeguards to ensure data security.
- Breach Notification Rule: Requires covered entities to notify patients and the Department of Health and Human Services (HHS) of any breaches of unsecured health information.
- Business Associate Agreements: Healthcare providers must have agreements with third-party vendors that handle patient information, ensuring they also comply with HIPAA regulations.
- Training and Awareness: Regular training for employees on HIPAA regulations and data protection practices is crucial for compliance.
Maintaining HIPAA compliance is vital for healthcare organizations to avoid legal penalties and protect patient trust.
6.4.3. International Regulations
International regulations play a significant role in the global healthcare landscape, ensuring that medical products meet safety and efficacy standards across different countries. Various organizations and agreements govern these regulations.
- World Health Organization (WHO): The WHO sets international health standards and guidelines, promoting global health and safety. Their prequalification program evaluates the quality, safety, and efficacy of medicines and vaccines.
- International Conference on Harmonisation (ICH): The ICH develops guidelines for drug development and registration, harmonizing regulatory requirements across Europe, Japan, and the United States. This helps streamline the approval process for pharmaceutical companies.
- European Medicines Agency (EMA): The EMA is responsible for the scientific evaluation, supervision, and safety monitoring of medicines in the European Union. It provides a centralized procedure for drug approval, allowing companies to market their products across EU member states.
- Regulatory Agencies: Each country has its own regulatory body, such as Health Canada or the Therapeutic Goods Administration (TGA) in Australia, which enforces local regulations and standards.
- Compliance Challenges: Companies must navigate varying regulations, which can complicate international marketing and distribution. Understanding local laws and requirements is crucial for successful global operations.
Adhering to international regulations is essential for companies looking to expand their market reach while ensuring the safety and efficacy of their products. This includes understanding the fda new drug approvals and fda medication approval processes.
7. Quality Assurance and Validation
Quality assurance and validation are critical components in various industries, particularly in healthcare, pharmaceuticals, and technology. These processes ensure that products and services meet specified requirements and standards, ultimately safeguarding public health and enhancing user trust. This includes practices such as quality assurance verification and validation in QA.
7.1 Performance Metrics

‍
Performance metrics are essential for evaluating the effectiveness and efficiency of a product or service. They provide quantifiable measures that help organizations assess their performance against established benchmarks. In the context of quality assurance and validation, performance metrics can include:
- Accuracy: Measures how closely a product's output aligns with the expected results. For instance, in diagnostic tools, accuracy is crucial for ensuring correct diagnoses.
- Reliability: Indicates the consistency of a product's performance over time. A reliable product will perform the same way under similar conditions, which is vital in clinical settings.
- Usability: Assesses how easy and intuitive a product is for users. High usability can lead to better user satisfaction and fewer errors in application.
- Compliance: Evaluates adherence to regulatory standards and guidelines. Compliance metrics ensure that products meet legal and safety requirements, which is particularly important in healthcare, especially in the context of FDA computer software assurance.
- Efficiency: Measures the resources used in relation to the output produced. Efficient processes can reduce costs and improve service delivery.
- Customer Satisfaction: Gauges user feedback and satisfaction levels. High customer satisfaction often correlates with product quality and effectiveness.
By regularly monitoring these performance metrics, organizations can identify areas for improvement, enhance product quality, and ensure that they meet the needs of their users. For example, a study found that organizations that implement performance metrics can improve their operational efficiency by up to 30%.
7.2 Clinical Validation Protocols
Clinical validation protocols are systematic processes designed to ensure that medical products, devices, or procedures are safe and effective for patient use. These protocols are essential for maintaining high standards in healthcare and involve several key components:
- Study Design: Establishing a robust study design is crucial for clinical validation. This includes defining the objectives, selecting appropriate methodologies, and determining the sample size to ensure statistically significant results.
- Regulatory Compliance: Adhering to regulatory requirements set by authorities such as the FDA or EMA is vital. These regulations dictate the necessary steps for clinical trials, including preclinical studies, clinical phases, and post-market surveillance, which are integral to computer software assurance FDA guidelines.
- Data Collection: Gathering accurate and comprehensive data is essential for validating clinical outcomes. This includes patient demographics, treatment protocols, and outcome measures.
- Statistical Analysis: Employing appropriate statistical methods to analyze the collected data helps determine the efficacy and safety of the product. This analysis is critical for drawing valid conclusions from the study.
- Risk Assessment: Identifying potential risks associated with the product or procedure is a key aspect of clinical validation. This includes evaluating adverse events and ensuring that benefits outweigh risks.
- Peer Review: Engaging in peer review processes can enhance the credibility of clinical validation. Independent experts can provide insights and feedback, ensuring that the protocols meet scientific and ethical standards.
- Post-Market Surveillance: Continuous monitoring of a product's performance after it has been released into the market is essential. This helps identify any long-term effects or unforeseen issues that may arise, which is a critical aspect of quality assurance validation.
Implementing rigorous clinical validation protocols not only ensures patient safety but also builds trust in healthcare products. According to a report, effective clinical validation can reduce the risk of product recalls by up to 50%.
In conclusion, both performance metrics and clinical validation protocols play a vital role in quality assurance. They help organizations maintain high standards, ensure compliance, and ultimately deliver safe and effective products to users. At Rapid Innovation, we leverage our expertise in AI and Blockchain to enhance these processes, ensuring that our clients achieve greater ROI through improved efficiency, compliance, and user satisfaction. This includes a focus on process validation in quality assurance and types of validation in quality assurance to further strengthen our approach. For more information, visit AI for Quality Assurance.
7.3. Monitoring and Maintenance
Monitoring and maintenance are critical components in ensuring the long-term success and reliability of any system, whether it be software, hardware, or a combination of both. Effective monitoring allows for the early detection of issues, while regular maintenance helps to prevent problems from escalating.
- Continuous Monitoring: Â
- Implement real-time monitoring tools to track system performance, leveraging AI algorithms to predict potential failures before they occur.
- Use metrics such as uptime, response time, and error rates to gauge system health, ensuring that your operations run smoothly and efficiently.
- Set up alerts for anomalies to facilitate immediate action, minimizing downtime and enhancing user satisfaction.
- Scheduled Maintenance: Â
- Conduct regular updates to software and hardware to ensure compatibility and security, utilizing blockchain technology for secure and transparent update processes.
- Perform routine checks on system components to identify wear and tear, ensuring that your infrastructure remains robust and reliable. This includes resetting tpms after tire rotation to maintain optimal performance in fleet management.
- Schedule downtime during off-peak hours to minimize disruption, optimizing resource allocation and maintaining productivity.
- Documentation: Â
- Maintain detailed logs of system performance and maintenance activities, creating a comprehensive history that can inform future decisions.
- Document any changes made to the system for future reference, ensuring that all stakeholders are aligned and informed.
- Create a knowledge base for troubleshooting common issues, empowering users and support teams to resolve problems quickly.
- User Feedback: Â
- Encourage users to report issues or suggest improvements, fostering a culture of continuous improvement and user-centric design.
- Analyze user feedback to identify areas needing attention, allowing for targeted enhancements that drive user satisfaction.
- Use surveys to gauge user satisfaction and system usability, ensuring that your solutions meet evolving needs.
7.4. Error Analysis and Correction
Error analysis and correction are essential for maintaining system integrity and user satisfaction. Identifying the root causes of errors can lead to more effective solutions and prevent future occurrences.
- Error Identification: Â
- Utilize logging mechanisms to capture error details, employing AI-driven analytics to identify patterns and trends in error occurrences.
- Categorize errors based on severity and frequency, allowing for prioritized responses that focus on critical issues.
- Employ automated tools to detect and report errors in real-time, ensuring swift action and minimal disruption.
- Root Cause Analysis: Â
- Conduct thorough investigations to determine the underlying causes of errors, using techniques such as the "5 Whys" or fishbone diagrams to facilitate analysis.
- Involve cross-functional teams to gain diverse perspectives on the issues, fostering collaboration and comprehensive problem-solving.
- Correction Strategies: Â
- Develop a systematic approach to address identified errors, ensuring that solutions are effective and sustainable.
- Prioritize fixes based on impact and urgency, focusing resources on the most critical issues that affect user experience.
- Implement changes in a controlled manner to avoid introducing new issues, maintaining system stability.
- Testing and Validation: Â
- After corrections are made, conduct rigorous testing to ensure effectiveness, utilizing automated testing frameworks to streamline the process.
- Use regression testing to confirm that existing functionalities remain intact, safeguarding against unintended consequences.
- Gather user feedback post-correction to assess satisfaction, ensuring that solutions meet user expectations.
8. Future Developments
Future developments in technology and systems are crucial for staying competitive and meeting evolving user needs. Organizations must be proactive in adapting to changes and exploring new opportunities.
- Emerging Technologies: Â
- Keep an eye on advancements in artificial intelligence, machine learning, and automation, integrating these technologies to enhance operational efficiency and decision-making.
- Explore the potential of blockchain for enhanced security and transparency, ensuring that your systems are resilient against threats and fraud.
- Investigate the use of Internet of Things (IoT) devices for improved data collection and analysis, enabling real-time insights that drive strategic initiatives, particularly in tracking fleet management software.
- User-Centric Design: Â
- Focus on enhancing user experience through intuitive interfaces and accessibility features, ensuring that your solutions are inclusive and user-friendly.
- Conduct user research to understand changing preferences and behaviors, allowing for agile adaptations that keep pace with market demands.
- Implement agile methodologies to quickly adapt to user feedback, fostering a responsive development environment.
- Sustainability Initiatives: Â
- Consider eco-friendly practices in system design and operation, aligning with corporate social responsibility goals and enhancing brand reputation.
- Explore energy-efficient technologies to reduce carbon footprints, contributing to a sustainable future.
- Engage in corporate social responsibility initiatives to enhance brand reputation, demonstrating commitment to ethical practices.
- Continuous Learning and Adaptation: Â
- Foster a culture of innovation within the organization, encouraging creativity and experimentation to drive growth.
- Encourage ongoing training and development for staff to keep skills current, ensuring that your team remains at the forefront of industry advancements.
- Stay informed about industry trends and competitor strategies to remain relevant, positioning your organization for sustained success.
By focusing on these areas, organizations can ensure they are well-prepared for future challenges and opportunities, ultimately leading to sustained growth and success. Rapid Innovation is committed to helping clients navigate these complexities, leveraging our expertise in AI and blockchain to drive greater ROI and operational excellence.
8.1. Emerging Technologies
Emerging technologies are reshaping industries and driving innovation across various sectors. These technologies often leverage advancements in artificial intelligence, machine learning, and data analytics to create solutions that were previously unimaginable. As businesses and organizations adopt these technologies, including new technology and new advanced technology, they can enhance efficiency, improve decision-making, and foster new business models. Key emerging technologies include:
- Artificial Intelligence (AI)
- Blockchain
- Internet of Things (IoT)
- Quantum Computing
- 5G Technology
- New AI technology
- Latest technological advancements
- Emerging technologies
- New technologies 2023
The rapid evolution of these technologies presents both opportunities and challenges, necessitating a deeper understanding of their implications and applications.
8.1.1. Federated Learning
Federated learning is a decentralized approach to machine learning that allows multiple devices to collaboratively learn a shared prediction model while keeping their data local. This technology is particularly significant in the context of privacy and data security, as it minimizes the need to transfer sensitive data to a central server.
Key features of federated learning include:
- Data remains on the device, enhancing privacy.
- Only model updates are shared, reducing data exposure.
- It enables learning from diverse datasets without compromising individual data privacy.
At Rapid Innovation, we harness federated learning to help clients in sectors such as healthcare and finance develop predictive models while ensuring compliance with data protection regulations like GDPR. For instance, in healthcare, we can assist organizations in creating accurate predictive models using patient data from multiple hospitals without compromising sensitive information. This not only protects patient privacy but also enhances the model's accuracy by leveraging a broader dataset, ultimately leading to greater ROI.
8.1.2. Explainable AI
Explainable AI (XAI) refers to methods and techniques in artificial intelligence that make the outputs of AI systems understandable to humans. As AI systems become more complex, the need for transparency and interpretability has become paramount. Explainable AI aims to bridge the gap between advanced AI algorithms and human comprehension.
Key aspects of explainable AI include:
- Transparency: Providing insights into how AI models make decisions.
- Interpretability: Ensuring that users can understand the rationale behind AI outputs.
- Trust: Building confidence in AI systems by making them more understandable.
At Rapid Innovation, we prioritize the implementation of explainable AI in our solutions, particularly in sectors where decisions have significant consequences, such as healthcare, finance, and autonomous vehicles. For example, we can develop AI systems that predict patient outcomes while providing clear explanations for their recommendations. This ensures that healthcare professionals can trust and validate the AI's suggestions, leading to improved patient care and operational efficiency.
Moreover, with regulatory bodies increasingly emphasizing the need for explainability in AI systems, our expertise in this area helps organizations comply with legal standards and ethical considerations, further enhancing their ROI.
In summary, both federated learning and explainable AI represent significant advancements in the field of artificial intelligence. They address critical issues related to data privacy and the need for transparency, making them essential components of the future of AI technology. At Rapid Innovation, we are committed to leveraging these technologies, including emerging tech and new battery technology, to help our clients achieve their business goals efficiently and effectively.
8.1.3. AutoML in Medical Imaging
AutoML, or Automated Machine Learning, is revolutionizing the field of medical imaging by simplifying the process of developing machine learning models. This technology automates the selection, training, and tuning of models, making it accessible to healthcare professionals who may not have extensive data science expertise.
- Streamlined workflows: AutoML in medical imaging tools can significantly reduce the time and effort required to build predictive models, allowing radiologists and clinicians to focus on patient care. Rapid Innovation can assist healthcare organizations in implementing AutoML solutions that enhance operational efficiency.
- Enhanced accuracy: By leveraging advanced algorithms, AutoML can improve the accuracy of image analysis in medical imaging, leading to better diagnostic outcomes. Our expertise in AI development ensures that clients benefit from state-of-the-art algorithms tailored to their specific needs.
- Scalability: AutoML can handle large datasets typical in medical imaging, enabling the analysis of vast amounts of data from various imaging modalities such as MRI, CT, and X-rays. Rapid Innovation's solutions are designed to scale with your data needs, ensuring that you can manage and analyze information effectively.
- Customization: Users can tailor AutoML solutions to specific medical imaging tasks, such as tumor detection or organ segmentation, enhancing the relevance of the models. We work closely with clients to develop customized solutions that align with their unique requirements.
- Integration with existing systems: AutoML can be integrated into existing healthcare IT systems, facilitating seamless adoption in clinical settings. Rapid Innovation specializes in ensuring that new technologies work harmoniously with your current infrastructure.
8.2. Research Directions
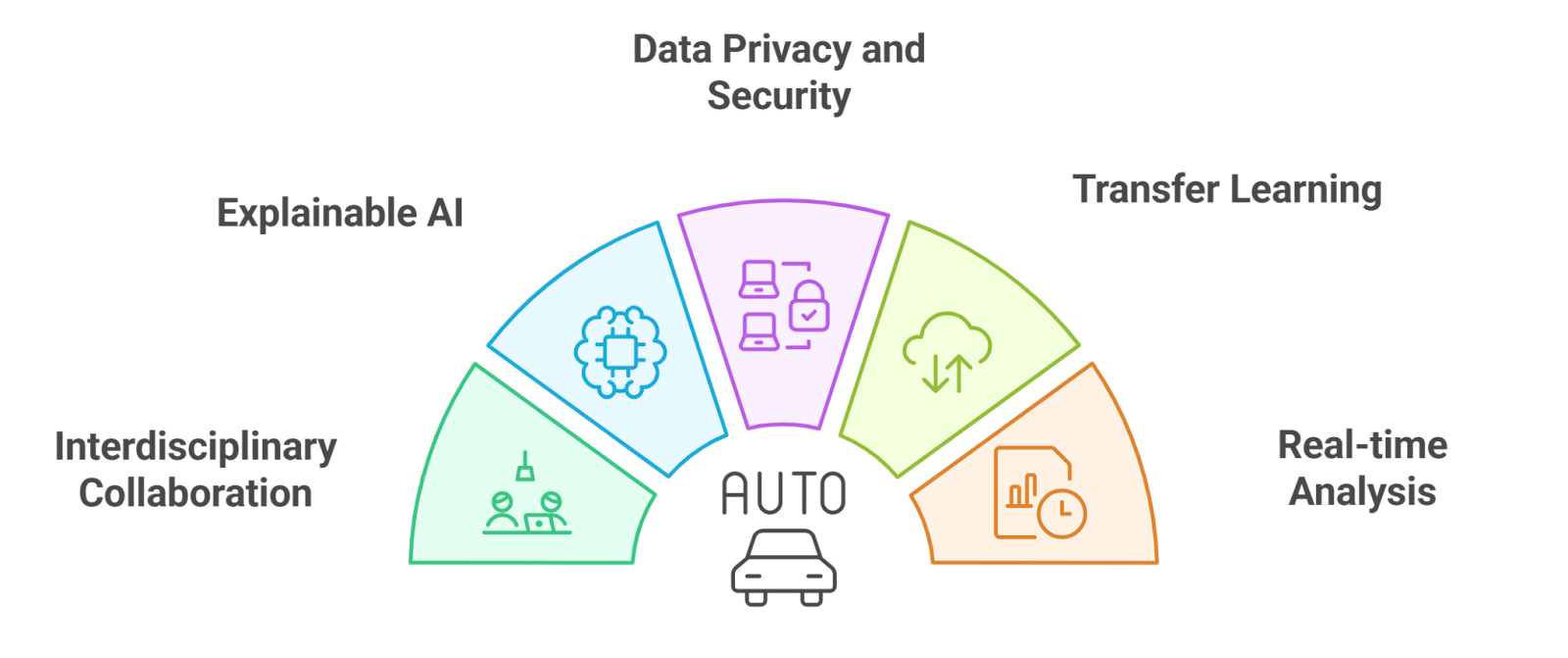
‍
The field of medical imaging is rapidly evolving, and several research directions are emerging that focus on enhancing the capabilities of AutoML and its applications in healthcare.
- Interdisciplinary collaboration: Researchers are increasingly collaborating across disciplines, combining expertise in computer science, radiology, and clinical practice to develop more effective AutoML solutions. Rapid Innovation fosters such collaborations to drive innovation in healthcare.
- Explainable AI: There is a growing emphasis on creating models that not only provide accurate predictions but also offer insights into their decision-making processes, which is crucial for clinical acceptance. Our team is dedicated to developing explainable AI solutions that enhance trust and transparency in medical applications.
- Data privacy and security: As medical imaging data is sensitive, research is focusing on developing AutoML techniques that ensure patient privacy while still delivering high-quality results. Rapid Innovation prioritizes data security in all our AI solutions, ensuring compliance with industry standards.
- Transfer learning: This approach allows models trained on one dataset to be adapted for another, which is particularly useful in medical imaging where labeled data can be scarce. We leverage transfer learning techniques to maximize the utility of existing datasets for our clients.
- Real-time analysis: Researchers are exploring ways to implement AutoML for real-time image analysis, which could significantly enhance the speed of diagnosis and treatment. Rapid Innovation is at the forefront of developing real-time solutions that empower healthcare providers to make timely decisions.
8.3. Potential Applications
The potential applications of AutoML in medical imaging are vast and varied, offering significant benefits to both healthcare providers and patients.
- Disease detection: AutoML can be used to develop models that detect diseases such as cancer, cardiovascular conditions, and neurological disorders from imaging data. Our solutions enable healthcare organizations to enhance their diagnostic capabilities.
- Predictive analytics: By analyzing historical imaging data, AutoML can help predict patient outcomes, enabling proactive treatment strategies. Rapid Innovation's predictive analytics tools empower clients to make informed decisions based on data-driven insights.
- Workflow optimization: AutoML can streamline imaging workflows by automating routine tasks, such as image preprocessing and quality control, allowing radiologists to focus on more complex cases. We help clients optimize their workflows for improved efficiency and patient care.
- Personalized medicine: By analyzing individual patient data, AutoML can assist in tailoring treatment plans based on specific imaging findings, leading to more effective care. Our expertise in AI allows us to support personalized medicine initiatives that enhance patient outcomes.
- Research and clinical trials: AutoML can facilitate the analysis of imaging data in clinical trials, helping researchers identify trends and outcomes more efficiently. Rapid Innovation provides the tools and expertise necessary for effective data analysis in research settings.
In conclusion, AutoML is poised to transform medical imaging by enhancing diagnostic accuracy, streamlining workflows, and enabling personalized patient care. As research continues to advance in this area, the potential applications will only expand, leading to improved healthcare outcomes. Rapid Innovation is committed to helping clients leverage AutoML to achieve their business goals efficiently and effectively. For more insights, check out the future of AI-powered healthcare solutions.
8.4. Industry Trends
The landscape of various industries is constantly evolving, influenced by technological advancements, consumer preferences, and global events. Understanding these trends is crucial for businesses to remain competitive and relevant. Here are some key industry trends to consider:
- Digital Transformation: Companies are increasingly adopting digital technologies to enhance operations, improve customer experiences, and drive innovation. This includes the use of artificial intelligence, machine learning, and big data analytics, which can lead to more informed decision-making and increased efficiency. The manufacturing industry trends are particularly focused on integrating these technologies to optimize production processes.
- Sustainability: There is a growing emphasis on sustainable practices across industries. Businesses are focusing on reducing their carbon footprint, utilizing renewable resources, and implementing eco-friendly processes to meet consumer demand for responsible products. Rapid Innovation can assist clients in integrating blockchain solutions to enhance transparency in supply chains, thereby promoting sustainability. This trend is also evident in the food and beverage industry overview, where sustainability is becoming a key differentiator.
- Remote Work: The shift towards remote work has become a permanent trend in many sectors. Organizations are investing in collaboration tools and technologies to support a distributed workforce, leading to changes in workplace culture and employee engagement strategies. AI-driven tools can help streamline communication and project management in remote settings, which is crucial for industries adapting to current industry trends.
- E-commerce Growth: The rise of e-commerce continues to reshape retail and service industries. Companies are enhancing their online presence and optimizing supply chains to meet the increasing demand for online shopping. Rapid Innovation can leverage AI to personalize customer experiences and optimize inventory management, driving higher sales and customer satisfaction. This trend is also reflected in the automotive manufacturing trends, where online sales channels are becoming more prominent.
- Health and Wellness: The focus on health and wellness has surged, particularly in the wake of the pandemic. Industries such as fitness, nutrition, and mental health are seeing increased investment and innovation to cater to consumer needs. AI applications can provide personalized health recommendations, improving user engagement and outcomes. Recent trends in manufacturing technology are also focusing on health-related products and services.
- Personalization: Consumers are seeking personalized experiences, prompting businesses to leverage data analytics to tailor products and services. This trend is evident in marketing strategies, product recommendations, and customer service. Rapid Innovation can help clients implement AI algorithms that analyze consumer behavior, leading to more effective marketing campaigns and increased ROI. This is particularly relevant in trend industries where customization is key.
- Cybersecurity: As digital transformation accelerates, the need for robust cybersecurity measures has become paramount. Companies are investing in advanced security solutions to protect sensitive data and maintain customer trust. Blockchain technology can enhance security protocols, providing immutable records and reducing the risk of data breaches. This is especially important in the technology industry 4.0, where data security is critical.
- Automation: Automation is transforming various sectors by streamlining processes and reducing operational costs. Industries are adopting robotic process automation (RPA) and other technologies to enhance efficiency. Rapid Innovation can assist clients in identifying areas for automation, leading to significant cost savings and improved productivity. Recent trends in manufacturing highlight the increasing reliance on automation technologies.
9. Implementation Guidelines
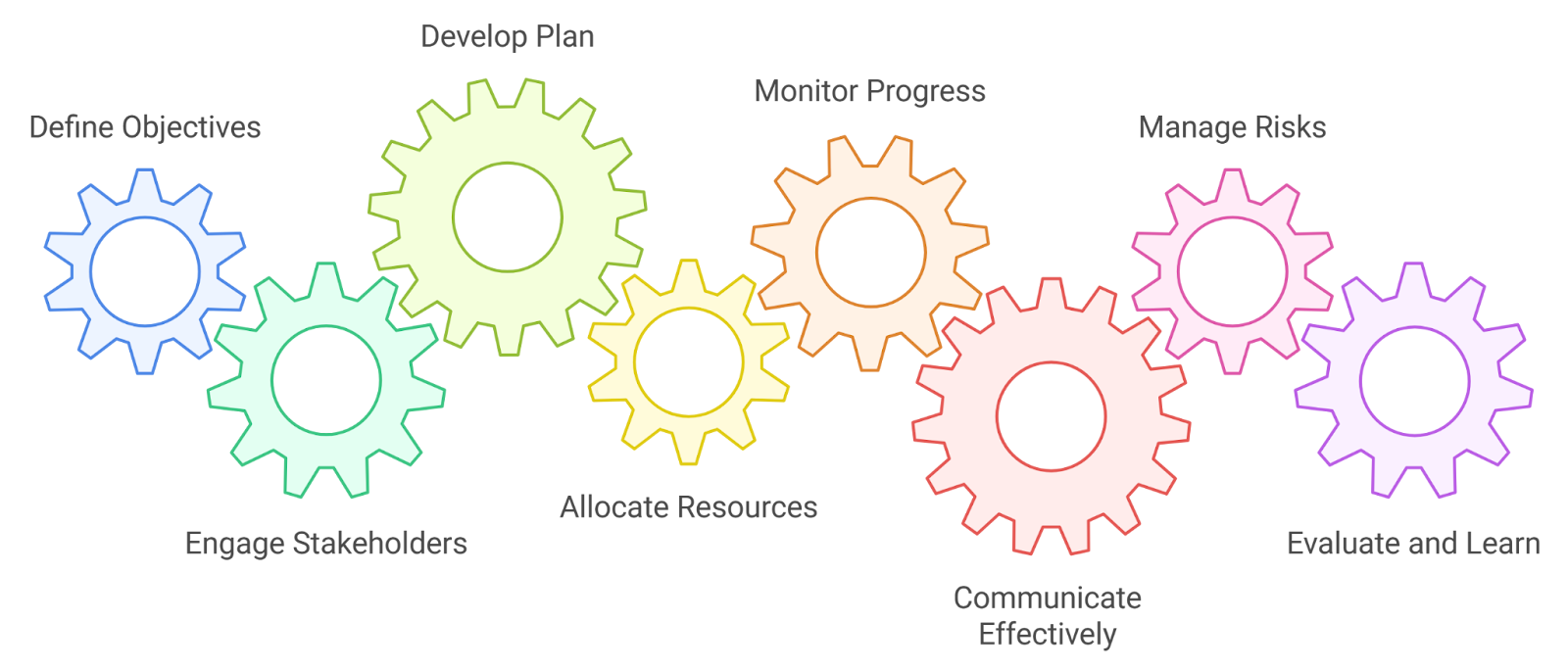
‍
Implementing a project successfully requires careful planning and execution. Here are some essential guidelines to follow during the implementation phase:
- Define Clear Objectives: Establish specific, measurable, achievable, relevant, and time-bound (SMART) objectives to guide the project. This clarity helps in aligning the team and resources effectively.
- Engage Stakeholders: Involve all relevant stakeholders early in the process. Their input can provide valuable insights and foster a sense of ownership, which is crucial for project success.
- Develop a Detailed Plan: Create a comprehensive project plan that outlines tasks, timelines, resources, and responsibilities. This plan serves as a roadmap for the team and helps in tracking progress.
- Allocate Resources Wisely: Ensure that the necessary resources, including budget, personnel, and technology, are allocated appropriately. This prevents bottlenecks and ensures smooth execution.
- Monitor Progress: Regularly review the project’s progress against the established objectives. Use key performance indicators (KPIs) to measure success and make adjustments as needed.
- Communicate Effectively: Maintain open lines of communication among team members and stakeholders. Regular updates and feedback loops help in addressing issues promptly and keeping everyone informed.
- Risk Management: Identify potential risks early in the project and develop mitigation strategies. This proactive approach minimizes disruptions and enhances project resilience.
- Evaluate and Learn: After project completion, conduct a thorough evaluation to assess what worked well and what didn’t. Document lessons learned to inform future projects and improve processes.
9.1. Project Planning
Effective project planning is the foundation of successful implementation. It involves several critical steps that ensure all aspects of the project are considered and addressed:
- Scope Definition: Clearly define the project scope, including deliverables, boundaries, and constraints. This helps in managing expectations and preventing scope creep.
- Timeline Creation: Develop a realistic timeline that outlines key milestones and deadlines. Use project management tools to visualize the timeline and track progress.
- Resource Identification: Identify the resources required for the project, including human resources, technology, and materials. Ensure that these resources are available when needed.
- Task Breakdown: Break down the project into smaller, manageable tasks. Assign responsibilities to team members and establish deadlines for each task to ensure accountability.
- Budget Planning: Create a detailed budget that outlines all costs associated with the project. Monitor expenses throughout the project to stay within budget and make adjustments as necessary.
- Stakeholder Analysis: Identify all stakeholders involved in the project and assess their interests and influence. Develop a stakeholder engagement plan to ensure their needs are addressed.
- Communication Plan: Establish a communication plan that outlines how information will be shared among team members and stakeholders. This includes regular meetings, updates, and reporting structures.
- Contingency Planning: Prepare for potential challenges by developing contingency plans. This ensures that the project can adapt to unforeseen circumstances without significant disruption.
9.2. Resource Requirements
Resource requirements are critical for the successful execution of any project or initiative. Identifying and allocating the necessary resources ensures that the team can operate efficiently and effectively.
- Human Resources: Skilled personnel are essential for project success, including project managers, developers, designers, and support staff. At Rapid Innovation, we emphasize the importance of specialized roles based on project complexity, such as data analysts for AI projects or blockchain developers for decentralized applications.
- Financial Resources: A well-defined budget is crucial, encompassing costs for personnel, technology, and operational expenses. Rapid Innovation assists clients in creating comprehensive budgets that allocate funds for unexpected expenses, thereby avoiding project delays and ensuring a greater return on investment (ROI).
- Technological Resources: Identify the software and hardware needed for project execution, which may include project management tools, communication platforms, and development environments. Tools for project tracking and task and project management tools are essential for maintaining oversight. Rapid Innovation ensures that the technology is scalable to accommodate future growth, particularly in AI and blockchain applications, where adaptability is key.
- Physical Resources: Consider the need for office space, equipment, and supplies, including computers, printers, and meeting rooms. Evaluate whether remote work options are viable to reduce physical resource needs. Rapid Innovation supports clients in optimizing their resource allocation, whether in a traditional office setting or a remote work environment.
- Time Resources: Establish a realistic timeline for project milestones and deliverables, factoring in time for team meetings, training, and unforeseen delays. Utilizing project schedule templates and project planner templates can aid in this process. Rapid Innovation's expertise in project management helps clients set achievable timelines that align with their business goals.
9.3. Team Structure and Roles
A well-defined team structure is essential for clarity and accountability within a project. Each team member should understand their roles and responsibilities to ensure smooth collaboration.
- Project Manager: Oversees the project from start to finish, ensuring that it stays on track and within budget. Acts as the primary point of contact for stakeholders and team members, facilitating communication and alignment with Rapid Innovation's strategic objectives.
- Team Leads: Responsible for specific areas of the project, such as development, design, or marketing. They coordinate with their respective teams to ensure alignment with project goals, leveraging Rapid Innovation's expertise in AI and blockchain technologies.
- Developers: Focus on coding and technical implementation, working closely with designers to bring concepts to life. They are also responsible for testing and debugging to ensure a high-quality product, particularly in the context of AI algorithms or blockchain smart contracts.
- Designers: Create the visual elements of the project, including user interfaces and graphics. They collaborate with developers to ensure design feasibility and functionality, enhancing user experience in AI applications and blockchain platforms.
- Quality Assurance (QA) Specialists: Test the product to identify bugs and ensure it meets quality standards. They provide feedback to developers for improvements, ensuring that Rapid Innovation's solutions are robust and reliable.
- Support Staff: Handle administrative tasks, documentation, and communication within the team. They ensure that the project runs smoothly by managing logistics and resources, allowing technical teams to focus on delivering innovative solutions.
9.4. Training and Education
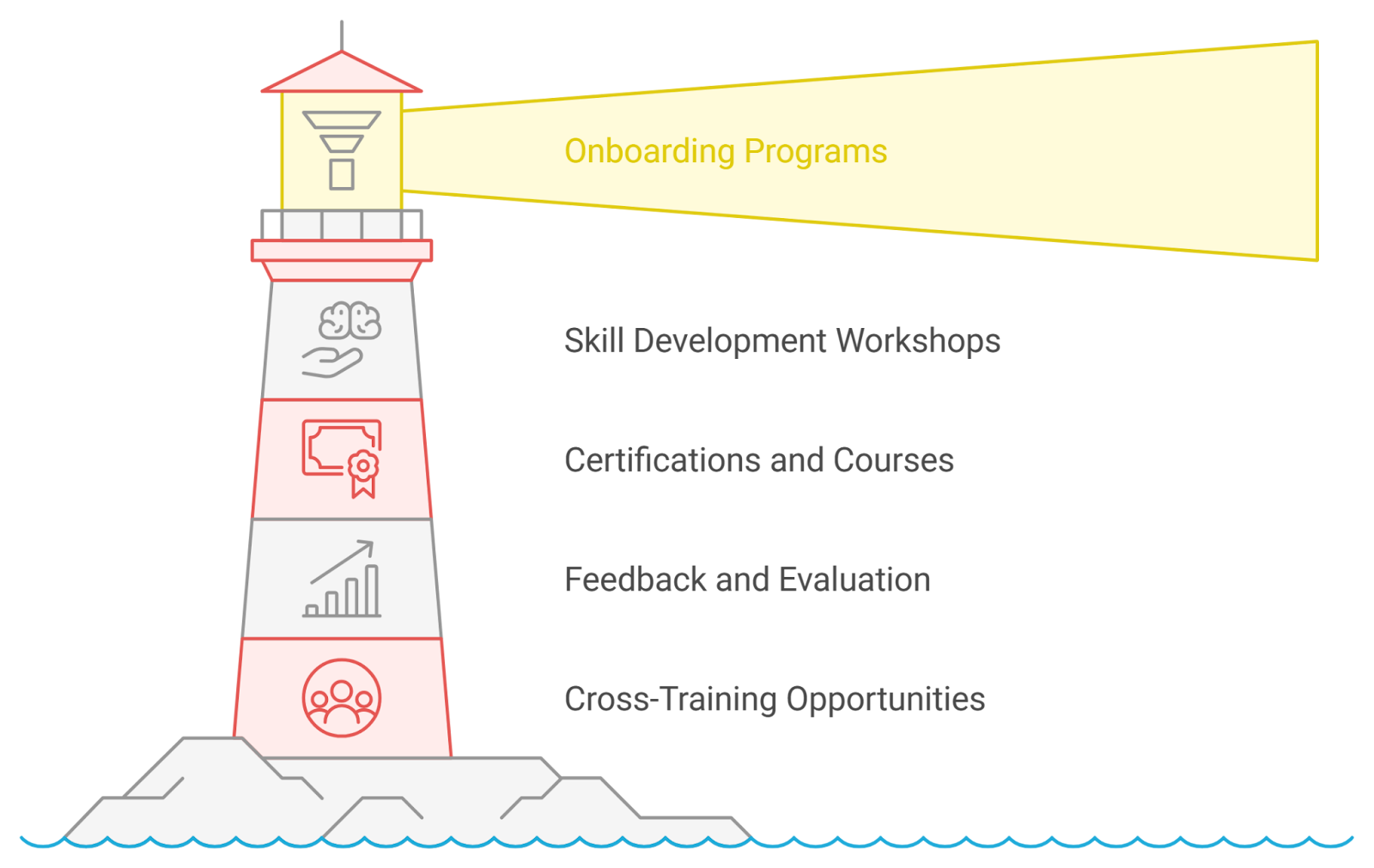
‍
Training and education are vital for enhancing team skills and ensuring that everyone is equipped to meet project demands. Continuous learning fosters a culture of improvement and innovation.
- Onboarding Programs: New team members should undergo a structured onboarding process to familiarize themselves with company culture, tools, and processes. Rapid Innovation provides resources such as manuals, videos, and mentorship to facilitate learning.
- Skill Development Workshops: Organize regular workshops to enhance specific skills relevant to the project, such as coding languages, design software, or project management techniques. Encourage team members to share knowledge and best practices, particularly in the rapidly evolving fields of AI and blockchain.
- Certifications and Courses: Support team members in pursuing relevant certifications that can enhance their expertise and contribute to project success. Rapid Innovation considers partnerships with educational institutions for access to specialized training programs in AI and blockchain technologies.
- Feedback and Evaluation: Implement a system for regular feedback and performance evaluations to identify areas for improvement. Use this information to tailor training programs to meet the team's evolving needs, ensuring that Rapid Innovation remains at the forefront of industry standards.
- Cross-Training Opportunities: Encourage team members to learn about different roles within the project, promoting versatility and collaboration. Cross-training can help mitigate risks associated with personnel changes or absences, ensuring that Rapid Innovation's projects maintain momentum.
By addressing resource requirements, establishing a clear team structure, and prioritizing training and education, organizations can enhance their project execution capabilities and drive successful outcomes, ultimately achieving greater ROI through the innovative solutions provided by Rapid Innovation. Tools management project and resource breakdown structure can further assist in optimizing these processes.
9.5. Risk Management
Risk management is a critical component of any project or business strategy, particularly in the realms of risk management in AI and Blockchain, where the landscape is constantly evolving. It involves identifying, assessing, and prioritizing risks followed by coordinated efforts to minimize, monitor, and control the probability or impact of unfortunate events. Effective risk management can safeguard resources and ensure project success.
- Identify Risks: Â
- Conduct thorough assessments to identify potential risks, including technological, regulatory, and market-related challenges.
- Use tools like SWOT analysis (Strengths, Weaknesses, Opportunities, Threats) to pinpoint vulnerabilities specific to AI and Blockchain implementations.
- Assess Risks: Â
- Evaluate the likelihood and impact of each identified risk, particularly focusing on data privacy, compliance with regulations, and the volatility of blockchain markets.
- Classify risks into categories such as operational, financial, strategic, and compliance, ensuring a comprehensive understanding of potential pitfalls.
- Develop Mitigation Strategies: Â
- Create action plans to address high-priority risks, leveraging AI-driven analytics to predict and respond to potential issues proactively.
- Implement preventive measures, such as robust cybersecurity protocols and compliance checks, to reduce the likelihood of risks occurring.
- Monitor and Review: Â
- Continuously monitor risks throughout the project lifecycle, utilizing real-time data analytics to stay ahead of emerging threats.
- Adjust strategies as necessary based on new information or changes in the project environment, ensuring agility in risk management.
- Communicate: Â
- Ensure that all stakeholders are aware of potential risks and mitigation strategies, fostering a culture of transparency and open communication regarding risk management.
- Utilize blockchain technology to create immutable records of risk assessments and decisions, enhancing accountability.
9.6. Success Metrics
Success metrics are essential for evaluating the performance and effectiveness of a project or business initiative, especially in AI and Blockchain projects. They provide quantifiable measures that help determine whether objectives are being met.
- Define Clear Objectives: Â
- Establish specific, measurable, achievable, relevant, and time-bound (SMART) goals that align with the unique capabilities of AI and Blockchain technologies.
- Align success metrics with overall business objectives, ensuring that they reflect the strategic vision of the organization.
- Key Performance Indicators (KPIs): Â
- Identify KPIs that reflect the success of the project, such as the accuracy of AI models, transaction speed in blockchain applications, and user adoption rates.
- Common KPIs include revenue growth, customer satisfaction, and project completion time, tailored to the specific context of AI and Blockchain initiatives.
- Data Collection: Â
- Implement systems for collecting relevant data, utilizing AI tools for data mining and analysis to gather insights efficiently.
- Use surveys, analytics tools, and performance tracking software to gather insights that inform decision-making.
- Analyze Results: Â
- Regularly review collected data to assess performance against success metrics, employing AI algorithms to identify trends and anomalies.
- Use data visualization tools to present findings clearly, making it easier for stakeholders to understand performance outcomes.
- Adjust Strategies: Â
- Based on the analysis, make informed decisions to improve performance, leveraging insights gained from AI analytics.
- Be flexible and willing to adapt strategies to enhance outcomes, ensuring that the organization remains responsive to changing market conditions.
10. Economic Considerations
Economic considerations play a vital role in decision-making processes for businesses and projects, particularly in the rapidly changing fields of AI and Blockchain. Understanding the economic environment can help organizations navigate challenges and seize opportunities.
- Market Analysis: Â
- Conduct thorough market research to understand economic trends affecting AI and Blockchain, including shifts in consumer behavior and technological advancements.
- Analyze factors such as inflation rates, interest rates, and consumer spending patterns to inform strategic decisions.
- Cost-Benefit Analysis: Â
- Evaluate the financial implications of projects or investments, particularly in terms of the ROI associated with AI and Blockchain technologies.
- Compare the expected benefits against the costs to determine feasibility, ensuring that investments align with long-term business goals.
- Budgeting: Â
- Develop a comprehensive budget that accounts for all potential expenses, including development costs, regulatory compliance, and ongoing maintenance.
- Monitor financial performance against the budget to ensure fiscal responsibility and optimize resource allocation.
- Funding Sources: Â
- Identify potential funding sources, including loans, grants, and investors interested in AI and Blockchain innovations.
- Assess the economic conditions that may affect funding availability, ensuring that the organization is well-positioned to secure necessary resources.
- Economic Impact: Â
- Consider the broader economic impact of business decisions, particularly how AI and Blockchain projects contribute to local economies, job creation, and community development.
- Evaluate how projects can drive innovation and efficiency, ultimately enhancing the organization's competitive advantage.
By focusing on risk management in AI and Blockchain, success metrics, and economic considerations, organizations can enhance their strategic planning and operational effectiveness, ultimately achieving greater ROI through the innovative solutions offered by Rapid Innovation.
10.1. Cost Analysis
Cost analysis is a critical component in evaluating the financial viability of a project or investment, including cost benefit analysis in healthcare. It involves a detailed examination of all costs associated with a particular initiative, allowing stakeholders to make informed decisions.
- Direct Costs: These are expenses that can be directly attributed to a project, such as materials, labor, and equipment.
- Indirect Costs: These costs are not directly tied to a specific project but are necessary for overall operations, such as utilities and administrative expenses.
- Fixed Costs: These remain constant regardless of the level of production or service delivery, such as rent and salaries.
- Variable Costs: These fluctuate based on the level of output, such as raw materials and hourly wages.
- Opportunity Costs: This refers to the potential benefits lost when choosing one alternative over another.
Conducting a thorough cost analysis helps organizations identify areas where they can reduce expenses and improve efficiency. For instance, cost effectiveness analysis in healthcare can provide insights into the most efficient use of resources. At Rapid Innovation, we leverage AI-driven analytics to provide deeper insights into cost structures, enabling clients to optimize their budgets and forecast future financial performance more accurately. Additionally, understanding revenue cycle management, where patient care meets financial wellness, is essential for enhancing overall financial health in healthcare organizations.
10.2. ROI Calculation
Return on Investment (ROI) calculation is essential for assessing the profitability of an investment. It measures the gain or loss generated relative to the amount invested.
- Formula: ROI is typically calculated using the formula: Â
language="language-plaintext"``` -a1b2c3- Â ROI = (Net Profit / Cost of Investment) x 100
- Net Profit: This is the total revenue generated from the investment minus the total costs associated with it.
- Time Frame: ROI can be calculated over different time frames, such as monthly, quarterly, or annually, depending on the nature of the investment.
- Benchmarking: Comparing ROI against industry standards or similar investments can provide context and help gauge performance.
A positive ROI indicates that the investment is generating more income than it costs, while a negative ROI suggests a loss. Understanding ROI is crucial for making strategic decisions about where to allocate resources. Rapid Innovation employs advanced AI algorithms to enhance ROI calculations, allowing clients to make data-driven decisions that maximize their returns, including those related to cost benefit analysis healthcare.
10.3. Reimbursement Models
Reimbursement models are frameworks that dictate how healthcare providers are compensated for their services. These models can significantly impact the financial health of healthcare organizations.
- Fee-for-Service: Providers are paid for each service rendered, incentivizing volume over value. This model can lead to unnecessary procedures and increased healthcare costs.
- Capitation: Providers receive a fixed amount per patient, regardless of the number of services provided. This model encourages preventive care but may lead to under-treatment.
- Value-Based Care: This model focuses on patient outcomes and quality of care rather than the quantity of services. Providers are rewarded for improving patient health and reducing costs.
- Bundled Payments: Providers receive a single payment for all services related to a specific treatment or condition, promoting coordinated care and cost efficiency.
Understanding these reimbursement models is essential for healthcare organizations to navigate financial challenges and optimize revenue streams. Each model has its advantages and disadvantages, and the choice of model can influence patient care and organizational sustainability. For example, cost benefit analysis health insurance can help determine the most effective reimbursement strategies. At Rapid Innovation, we utilize blockchain technology to enhance transparency and efficiency in reimbursement processes, ensuring that healthcare providers are compensated fairly while maintaining high standards of patient care.
10.4. Market Analysis

‍
Market analysis is a critical component of any business strategy, providing insights into the competitive landscape, customer preferences, and overall industry trends. A thorough market analysis helps businesses identify opportunities and threats, enabling them to make informed decisions. Here are the key elements of market analysis:
- Industry Overview: Understanding the industry in which a business operates is essential. This includes analyzing the size, growth rate, and overall health of the industry. For instance, the global e-commerce market is projected to grow significantly, with estimates suggesting it could reach $6.54 trillion by 2023. Rapid Innovation leverages AI and Blockchain technologies to help clients navigate these industry dynamics, ensuring they remain competitive and capitalize on growth opportunities.
- Target Market Identification: Identifying the target market is crucial for effective marketing strategies. This involves target market segmentation based on demographics, psychographics, and buying behavior. Key factors to consider include age, gender, income level, and lifestyle choices. Rapid Innovation utilizes AI-driven analytics to provide clients with precise insights into their target demographics, enabling tailored marketing strategies that enhance customer engagement and conversion rates.
- Competitive Analysis: Analyzing competitors helps businesses understand their strengths and weaknesses. This includes identifying direct and indirect competitors, evaluating their market share, assessing their marketing strategies, and understanding their product offerings. With our expertise in AI, Rapid Innovation can assist clients in conducting in-depth competitive market analysis, allowing them to identify gaps in the market and develop strategies to outperform their rivals.
- Market Trends: Keeping an eye on market trends is vital for staying relevant. This includes monitoring technological advancements, changes in consumer behavior, economic factors affecting purchasing power, and regulatory changes impacting the industry. Rapid Innovation employs advanced AI algorithms to track and predict market trends, enabling clients to adapt their strategies proactively and maintain a competitive edge.
- Customer Needs and Preferences: Understanding what customers want is key to developing products and services that meet their needs. This can be achieved through surveys and questionnaires, focus groups, and analyzing customer feedback and reviews. Rapid Innovation harnesses AI to analyze customer data, providing actionable insights that help clients refine their offerings and enhance customer satisfaction.
- SWOT Analysis: Conducting a SWOT analysis (Strengths, Weaknesses, Opportunities, Threats) provides a comprehensive view of the business's position in the market. This helps in identifying internal strengths and weaknesses, recognizing external opportunities and threats, and formulating strategies to leverage strengths and mitigate weaknesses. Rapid Innovation can facilitate this process by integrating AI tools that streamline data collection and analysis, ensuring a thorough and accurate assessment. This includes swot analysis for marketing plan and swot analysis for a marketing plan.
- Market Size and Growth Potential: Estimating the market size and growth potential is essential for forecasting revenue and planning for expansion. This involves analyzing historical data, projecting future growth rates, and understanding market saturation levels. Rapid Innovation's expertise in data analytics allows clients to make informed decisions based on robust market forecasts, ultimately driving greater ROI.
- Pricing Strategy: An effective pricing strategy is crucial for attracting customers while maintaining profitability. Factors to consider include competitor pricing, customer willingness to pay, and the cost of production and distribution. Rapid Innovation employs AI-driven pricing models that help clients optimize their pricing strategies, ensuring they remain competitive while maximizing profit margins.
- Distribution Channels: Identifying the most effective distribution channels is vital for reaching the target market. This includes online platforms, retail partnerships, and direct sales. Rapid Innovation can assist clients in leveraging Blockchain technology to enhance supply chain transparency and efficiency, ensuring products reach customers in a timely and cost-effective manner.
- Regulatory Environment: Understanding the regulatory landscape is essential for compliance and risk management. This includes industry-specific regulations, consumer protection laws, and environmental regulations. Rapid Innovation's expertise in Blockchain can help clients navigate complex regulatory environments, ensuring compliance while minimizing risks.
By conducting a comprehensive market analysis, businesses can gain valuable insights that inform their strategic planning and operational decisions. This analysis not only helps in identifying potential growth areas but also in mitigating risks associated with market fluctuations. Rapid Innovation is committed to empowering clients with the tools and insights necessary to achieve their business goals efficiently and effectively, ultimately driving greater ROI. This includes market analysis in business plan, market research strategy, and market planning and research. Additionally, utilizing marketing strategy swot and marketing strategy research can further enhance the effectiveness of the analysis. For those interested in leveraging technology for trading, Rapid Innovation offers specialized services such as top 15 use cases of AI agents in business..